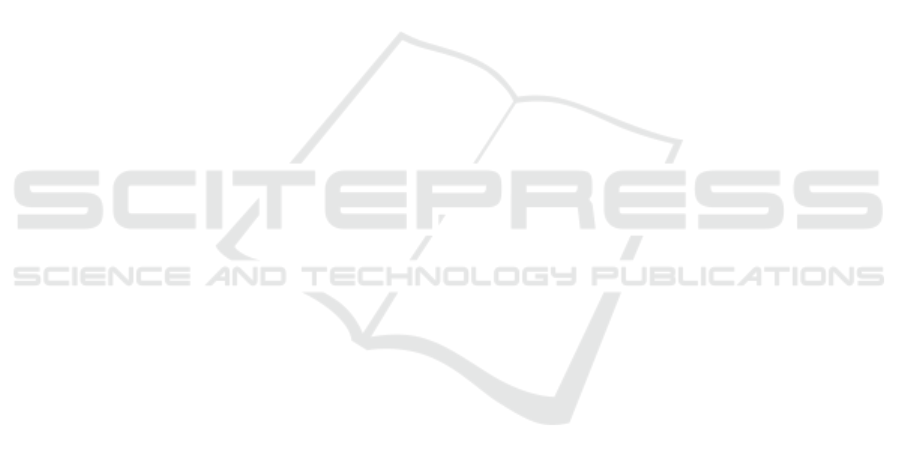
resent a significant step towards the goal of custom-
designed proteins well-focused on specific functions.
We outline that fine-tuning the ProGen Condi-
tional Transformer toward specific protein families
can enable the generation of new proteins that retain
and can also expand their functional characteristics,
with possible relevant applications in pharmacology
(e.g., for the design of new anti-microbic drugs), or
in industrial applications (e.g., for the production of
textiles, biofuels or foods).
In perspective, ProGen model can be fine-tuned on
more complex tasks. For instance, the generation of
functionally characterized protein molecules that can
interact with a specific molecular target (i.e., a target
protein). This is a challenging task, but it represents
our next objective.
ACKNOWLEDGEMENTS
This research was supported by the “National
Center for Gene Therapy and Drugs based on
RNA Technology”, PNRR-NextGenerationEU pro-
gram [G43C22001320007].
REFERENCES
Bengio, Y., Ducharme, R., and Vincent, P. (2000). A neu-
ral probabilistic language model. Advances in neural
information processing systems, 13.
Bommasani, R. et al. (2021). On the opportunities and risks
of foundation models. ArXiv, abs/2108.07258.
Brown, T. et al. (2020). Language models are few-shot
learners. Advances in neural information processing
systems, 33:1877–1901.
Cock, P., Chilton, J., Gr
¨
uning, B., Johnson, J., and Soranzo,
N. (2015). Ncbi blast+ integrated into galaxy. Giga-
science, 4(1):s13742–015.
Das, P. et al. (2021). Accelerated antimicrobial discov-
ery via deep generative models and molecular dy-
namics simulations. Nature Biomedical Engineering,
5(6):613–623.
Devlin, J., Chang, M., Lee, K., and Toutanova, K. (2019).
BERT: Pre-training of deep bidirectional transformers
for language understanding. In Proc. of the 2019 Con-
ference of the North American Chapter of the ACL,
Volume 1 (Long and Short Papers), pages 4171–4186.
ACL.
Ferruz, N., Heinzinger, M., Akdel, M., Goncearenco, A.,
Naef, L., and Dallago, C. (2022a). From sequence to
function through structure: deep learning for protein
design. Computational and Structural Biotechnology
Journal.
Ferruz, N. and H
¨
ocker, B. (2022). Controllable protein de-
sign with language models. Nature Machine Intelli-
gence, 4(6):521–532.
Ferruz, N., Schmidt, S., and H
¨
ocker, B. (2022b). Protgpt2
is a deep unsupervised language model for protein de-
sign. Nature communications, 13(1):4348.
Fu, L., Niu, B., Zhu, Z., Wu, S., and Li, W. (2012). Cd-
hit: accelerated for clustering the next-generation se-
quencing data. Bioinformatics, 28(23):3150–3152.
Jumper, J. et al. (2021). Highly accurate protein structure
prediction with alphafold. Nature, 596(7873):583–
589.
Keskar, N., McCann, B., Varshney, L., Xiong, C., and
Socher, R. (2019). CTRL: A conditional transformer
language model for controllable generation. arXiv
preprint arXiv:1909.05858.
Kilinc, M., Jia, K., and Jernigan, R. (2023). Improved
global protein homolog detection with major gains in
function identification. Proceedings of the National
Academy of Sciences, 120(9):e2211823120.
Kingma, D. and Ba, J. (2014). Adam: A method for
stochastic optimization. CoRR, abs/1412.6980.
Larkin, M. et al. (2007). Clustal w and clustal x version 2.0.
Bioinformatics, 23(21):2947–2948.
Madani, A. et al. (2023). Large language models generate
functional protein sequences across diverse families.
Nature Biotechnology, pages 1–8.
Mistry, J. et al. (2020). Pfam: The protein families database
in 2021. Nucleic Acids Research, 49(D1):D412–
D419.
Moor, M., Banerjee, O., Shakeri, Z., Krumholz, H.,
Leskovec, J., Topol, E., and Rajpurkar, P. (2023).
Foundation models for generalist medical artificial in-
telligence. Nature, 616:259–265.
OpenAI (2023). GPT-4 Technical Report. arXiv.
Price, M., Dehal, P., and Arkin, A. (2009). Fasttree: com-
puting large minimum evolution trees with profiles in-
stead of a distance matrix. Molecular biology and evo-
lution, 26(7):1641–1650.
Schr
¨
odinger, LLC (2023). The PyMOL molecular graphics
system, version 2.5.
Shin, J. et al. (2021). Protein design and variant prediction
using autoregressive generative models. Nature com-
munications, 12(1):2403.
Shuai, R., Ruffolo, J., and Gray, J. (2022). Generative lan-
guage modeling for antibody design. bioRxiv.
The UniProt Consortium (2022). UniProt: the Universal
Protein Knowledgebase in 2023. Nucleic Acids Re-
search, 51(D1):D523–D531.
Valentini, G., Malchiodi, D., Gliozzo, J., Mesiti, M., Soto-
Gomez, M., Cabri, A., Reese, J., Casiraghi, E., and
Robinson, P. (2023). The promises of large language
models for protein design and modeling. Frontiers in
Bioinformatics, 3:1304099.
Vaswani, A. et al. (2017). Attention is all you need. Ad-
vances in neural information processing systems, 30.
Wolf, T. et al. (2020). Transformers: State-of-the-art natural
language processing. In Proc. of the 2020 conference
on empirical methods in Natural Language Process-
ing, pages 38–45.
BIOINFORMATICS 2024 - 15th International Conference on Bioinformatics Models, Methods and Algorithms
568