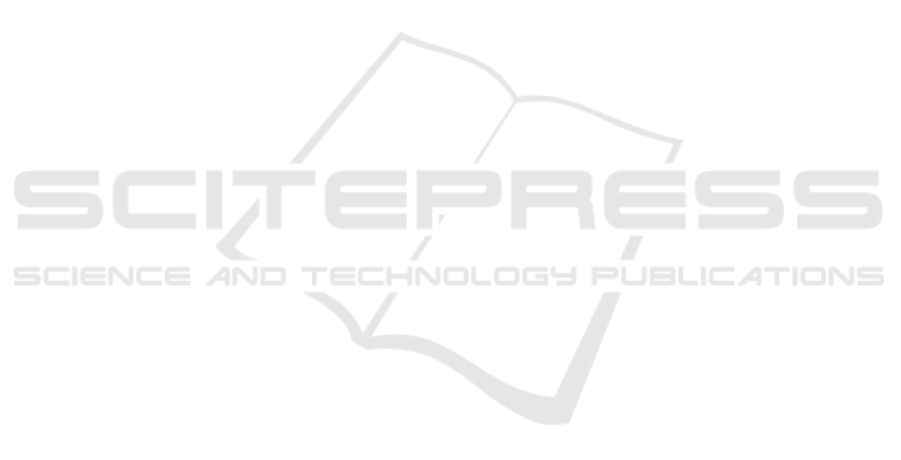
He, R., Sun, S., Yu, X., Xue, C., Zhang, W., Torr, P., Bai, S.,
and Qi, X. (2022). Is synthetic data from generative
models ready for image recognition? arXiv preprint
arXiv:2210.07574.
Hou, X., Shen, L., Sun, K., and Qiu, G. (2017). Deep fea-
ture consistent variational autoencoder. In 2017 IEEE
winter conference on applications of computer vision
(WACV), pages 1133–1141. IEEE.
Huang, H., He, R., Sun, Z., Tan, T., et al. (2018). In-
trovae: Introspective variational autoencoders for pho-
tographic image synthesis. Advances in neural infor-
mation processing systems, 31.
in the Loop, H. (2018). Humans in the loop. https:
//humansintheloop.org/. Accessed: 2023-11-22.
Islam, J. and Zhang, Y. (2020). Gan-based synthetic brain
pet image generation. Brain informatics, 7:1–12.
Jocher, G., Stoken, A., Borovec, J., NanoCode012, Christo-
pherSTAN, Changyu, L., Laughing, tkianai, Hogan,
A., lorenzomammana, yxNONG, AlexWang1900, Di-
aconu, L., Marc, wanghaoyang0106, ml5ah, Doug,
Ingham, F., Frederik, Guilhen, Hatovix, Poznanski, J.,
Fang, J., Yu, L., changyu98, Wang, M., Gupta, N.,
Akhtar, O., PetrDvoracek, and Rai, P. (2020). ultra-
lytics/yolov5: v3.1 - Bug Fixes and Performance Im-
provements.
Kingma, D. P. and Welling, M. (2013). Auto-encoding vari-
ational bayes. CoRR, abs/1312.6114.
Kumar, A., Sattigeri, P., and Balakrishnan, A. (2017).
Variational inference of disentangled latent con-
cepts from unlabeled observations. arXiv preprint
arXiv:1711.00848.
Liu, W., W. Luo, D. L., and Gao, S. (2018). Future frame
prediction for anomaly detection – a new baseline. In
2018 IEEE Conference on Computer Vision and Pat-
tern Recognition (CVPR).
Lu, C., Shi, J., and Jia, J. (2013). Abnormal event detection
at 150 fps in matlab. In Proceedings of the IEEE inter-
national conference on computer vision, pages 2720–
2727.
L
¨
uddecke, T. and Ecker, A. (2022). Image segmentation
using text and image prompts. In Proceedings of the
IEEE/CVF Conference on Computer Vision and Pat-
tern Recognition, pages 7086–7096.
Madan, N., Siemon, M. S. N., Gjerde, M. K., Petersson,
B. S., Grotuzas, A., Esbensen, M. A., Nikolov, I. A.,
Philipsen, M. P., Nasrollahi, K., and Moeslund, T. B.
(2023). Thermalsynth: A novel approach for gener-
ating synthetic thermal human scenarios. In Proceed-
ings of the IEEE/CVF Winter Conference on Applica-
tions of Computer Vision, pages 130–139.
Nikolenko, S. I. (2021). Synthetic Data for Deep Learning.
Number 978-3-030-75178-4 in Springer Optimization
and Its Applications. Springer.
Nikolov, I. (2023). Augmenting Anomaly Detection
Datasets with Reactive Synthetic Elements. In Van-
gorp, P. and Hunter, D., editors, Computer Graphics
and Visual Computing (CGVC). The Eurographics As-
sociation.
Nikolov, I. A., Philipsen, M. P., Liu, J., Dueholm, J. V.,
Johansen, A. S., Nasrollahi, K., and Moeslund, T. B.
(2021). Seasons in drift: A long-term thermal imaging
dataset for studying concept drift. In Thirty-fifth Con-
ference on Neural Information Processing Systems.
Neural Information Processing Systems Foundation.
P
´
erez, P., Gangnet, M., and Blake, A. (2023). Poisson im-
age editing. In Seminal Graphics Papers: Pushing the
Boundaries, Volume 2, pages 577–582.
Rainforth, T., Kosiorek, A., Le, T. A., Maddison, C., Igl,
M., Wood, F., and Teh, Y. W. (2018). Tighter varia-
tional bounds are not necessarily better. In Interna-
tional Conference on Machine Learning, pages 4277–
4285. PMLR.
Razavi, A., Van den Oord, A., and Vinyals, O. (2019).
Generating diverse high-fidelity images with vq-vae-
2. Advances in neural information processing systems,
32.
Rombach, R., Blattmann, A., Lorenz, D., Esser, P., and
Ommer, B. (2022). High-resolution image synthesis
with latent diffusion models. In Proceedings of the
IEEE/CVF Conference on Computer Vision and Pat-
tern Recognition (CVPR), pages 10684–10695.
Ros, G., Sellart, L., Materzynska, J., Vazquez, D., and
Lopez, A. M. (2016). The synthia dataset: A large
collection of synthetic images for semantic segmenta-
tion of urban scenes. In Proceedings of the IEEE con-
ference on computer vision and pattern recognition,
pages 3234–3243.
Tripathi, S., Chandra, S., Agrawal, A., Tyagi, A., Rehg,
J. M., and Chari, V. (2019). Learning to generate
synthetic data via compositing. In Proceedings of the
IEEE/CVF Conference on Computer Vision and Pat-
tern Recognition, pages 461–470.
V7Labs (2019). V7 labs annotation. https://www.v7labs.
com/. Accessed: 2023-11-22.
Voigt, P. and Von dem Bussche, A. (2017). The eu gen-
eral data protection regulation (gdpr). A Practical
Guide, 1st Ed., Cham: Springer International Pub-
lishing, 10(3152676):10–5555.
Wang, Q., Gao, J., Lin, W., and Yuan, Y. (2019). Learn-
ing from synthetic data for crowd counting in the
wild. In Proceedings of the IEEE/CVF conference on
computer vision and pattern recognition, pages 8198–
8207.
VISAPP 2024 - 19th International Conference on Computer Vision Theory and Applications
836