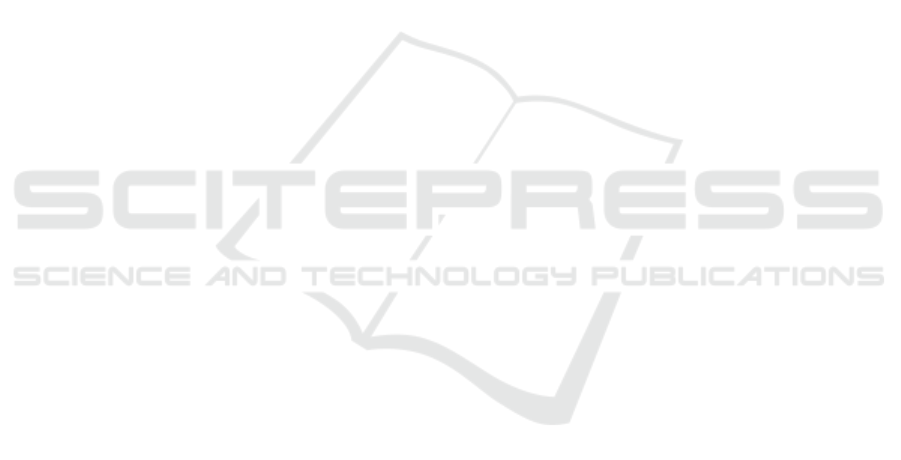
networks for unsupervised domain adaptation. In
Computer Vision–ECCV 2016: 14th European Con-
ference, Amsterdam, The Netherlands, October 11–
14, 2016, Proceedings, Part IV 14, pages 597–613.
Springer.
He, K., Zhang, X., Ren, S., and Sun, J. (2015). Deep resid-
ual learning for image recognition.
Ionescu, C., Papava, D., Olaru, V., and Sminchisescu, C.
(2014). Human3.6m: Large scale datasets and pre-
dictive methods for 3d human sensing in natural envi-
ronments. IEEE Transactions on Pattern Analysis and
Machine Intelligence, 36(7):1325–1339.
Jain, A., Zamir, A. R., Savarese, S., and Saxena, A.
(2016). Structural-rnn: Deep learning on spatio-
temporal graphs.
Lang, Y., Wang, Q., Yang, Y., Hou, C., Huang, D., and Xi-
ang, W. (2019). Unsupervised domain adaptation for
micro-doppler human motion classification via feature
fusion. IEEE Geoscience and Remote Sensing Letters,
16(3):392–396.
Long, M., Wang, J., Ding, G., Sun, J., and Yu, P. S. (2013).
Transfer feature learning with joint distribution adap-
tation. In Proceedings of the IEEE International Con-
ference on Computer Vision (ICCV).
Luo, Y., Huang, Z., Wang, Z., Zhang, Z., and Baktashmot-
lagh, M. (2020). Adversarial bipartite graph learning
for video domain adaptation. In Proceedings of the
28th ACM International Conference on Multimedia,
MM ’20. ACM.
Mitsuzumi, Y., Irie, G., Kimura, A., and Nakazawa, A.
(2024). Phase randomization: A data augmentation
for domain adaptation in human action recognition.
Pattern Recognition, 146:110051.
Na, J., Han, D., Chang, H. J., and Hwang, W. (2022). Con-
trastive vicinal space for unsupervised domain adap-
tation.
Pareek, P. and Thakkar, A. (2021). A survey on video-based
human action recognition: recent updates, datasets,
challenges, and applications. Artificial Intelligence
Review, 54:2259–2322.
Sofianos, T., Sampieri, A., Franco, L., and Galasso, F.
(2021). Space-time-separable graph convolutional
network for pose forecasting. In Proceedings of the
IEEE/CVF International Conference on Computer Vi-
sion (ICCV), pages 11209–11218.
Song, X., Zhao, S., Yang, J., Yue, H., Xu, P., Hu, R., and
Chai, H. (2021). Spatio-temporal contrastive domain
adaptation for action recognition. In Proceedings of
the IEEE/CVF Conference on Computer Vision and
Pattern Recognition, pages 9787–9795.
Tas, Y. and Koniusz, P. (2018). Cnn-based action recog-
nition and supervised domain adaptation on 3d body
skeletons via kernel feature maps.
Tzeng, E., Hoffman, J., Saenko, K., and Darrell, T. (2017).
Adversarial discriminative domain adaptation. In Pro-
ceedings of the IEEE conference on computer vision
and pattern recognition, pages 7167–7176.
Xu, T., Chen, W., Wang, P., Wang, F., Li, H., and Jin, R.
(2022). Cdtrans: Cross-domain transformer for unsu-
pervised domain adaptation.
Zhang, H., Ciss
´
e, M., Dauphin, Y. N., and Lopez-Paz, D.
(2017). mixup: Beyond empirical risk minimization.
CoRR, abs/1710.09412.
Zhu, J., Bai, H., and Wang, L. (2023). Patch-mix trans-
former for unsupervised domain adaptation: A game
perspective.
VISAPP 2024 - 19th International Conference on Computer Vision Theory and Applications
844