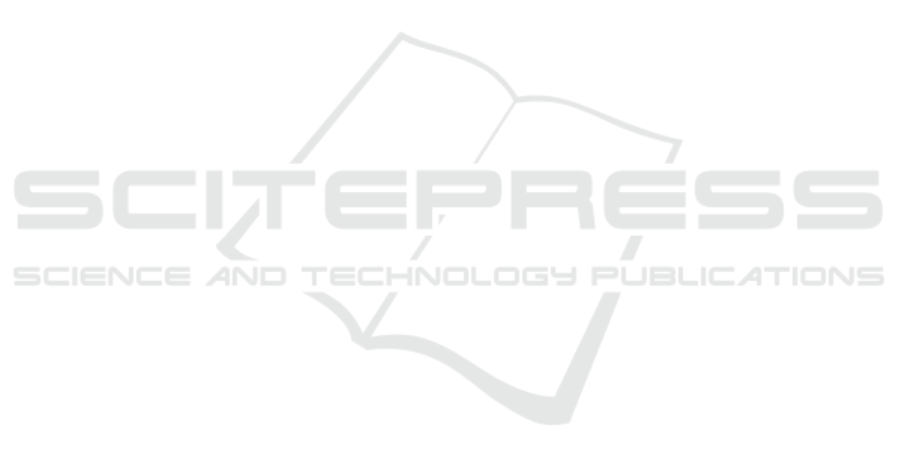
Li, J., Lin, X., Che, H., Li, H., and Qian, X. (2021a). Pan-
creas segmentation with probabilistic map guided bi-
directional recurrent unet. Physics in Medicine and
Biology, 66(11):115010.
Li, M., Lian, F., and Guo, S. (2021b). Automatic pancreas
segmentation using double adversarial networks with
pyramidal pooling module. IEEE Access, 9:140965–
140974.
Lin, T.-Y., Doll
´
ar, P., Girshick, R., He, K., Hariharan, B.,
and Belongie, S. (2017a). Feature pyramid networks
for object detection. In Proceedings of the IEEE con-
ference on computer vision and pattern recognition,
pages 2117–2125.
Lin, T.-Y., Goyal, P., Girshick, R., He, K., and Doll
´
ar, P.
(2017b). Focal loss for dense object detection. In
Proceedings of the IEEE international conference on
computer vision, pages 2980–2988.
Liu, S., Yuan, X., Hu, R., Liang, S., Feng, S., Ai, Y., and
Zhang, Y. (2019). Automatic pancreas segmentation
via coarse location and ensemble learning. IEEE Ac-
cess, 8:2906–2914.
Ma, H., Zou, Y., and Liu, P. X. (2021). Mhsu-net:
A more versatile neural network for medical image
segmentation. Computer Methods and Programs in
Biomedicine, 208:106230.
Mo, J., Zhang, L., Wang, Y., and Huang, H. (2020). It-
erative 3d feature enhancement network for pancreas
segmentation from ct images. Neural Computing and
Applications, 32:12535–12546.
Oktay, O., Schlemper, J., Folgoc, L. L., Lee, M., Heinrich,
M., Misawa, K., Mori, K., McDonagh, S., Hammerla,
N. Y., Kainz, B., et al. (2018). Attention u-net: Learn-
ing where to look for the pancreas. arXiv preprint
arXiv:1804.03999.
Ronneberger, O., Fischer, P., and Brox, T. (2015). U-
net: Convolutional networks for biomedical image
segmentation. In Medical Image Computing and
Computer-Assisted Intervention–MICCAI 2015: 18th
International Conference, Munich, Germany, October
5-9, 2015, Proceedings, Part III 18, pages 234–241.
Springer.
Roth, H. R., Lu, L., Farag, A., Shin, H.-C., Liu, J., Turkbey,
E. B., and Summers, R. M. (2015). Deeporgan: Multi-
level deep convolutional networks for automated pan-
creas segmentation. In Medical Image Computing and
Computer-Assisted Intervention–MICCAI 2015: 18th
International Conference, Munich, Germany, October
5-9, 2015, Proceedings, Part I 18, pages 556–564.
Springer.
Seeram, E. (2015). Computed Tomography-E-Book: Phys-
ical Principles, Clinical Applications, and Quality
Control. Elsevier Health Sciences.
Simonyan, K. and Zisserman, A. (2014). Very deep con-
volutional networks for large-scale image recognition.
arXiv preprint arXiv:1409.1556.
Simpson, A. L., Antonelli, M., Bakas, S., Bilello, M.,
Farahani, K., Van Ginneken, B., Kopp-Schneider, A.,
Landman, B. A., Litjens, G., Menze, B., et al. (2019).
A large annotated medical image dataset for the de-
velopment and evaluation of segmentation algorithms.
arXiv.
Sudre, C. H., Li, W., Vercauteren, T., Ourselin, S., and
Jorge Cardoso, M. (2017). Generalised dice over-
lap as a deep learning loss function for highly unbal-
anced segmentations. In Deep Learning in Medical
Image Analysis and Multimodal Learning for Clini-
cal Decision Support: Third International Workshop,
DLMIA 2017, and 7th International Workshop, ML-
CDS 2017, Held in Conjunction with MICCAI 2017,
Qu
´
ebec City, QC, Canada, September 14, Proceed-
ings 3, pages 240–248. Springer.
Szegedy, C., Vanhoucke, V., Ioffe, S., Shlens, J., and Wo-
jna, Z. (2016). Rethinking the inception architecture
for computer vision. In Proceedings of the IEEE con-
ference on computer vision and pattern recognition,
pages 2818–2826.
Yang, Z., Zhang, L., Zhang, M., Feng, J., Wu, Z., Ren, F.,
and Lv, Y. (2019). Pancreas segmentation in abdomi-
nal ct scans using inter-/intra-slice contextual informa-
tion with a cascade neural network. In 2019 41st An-
nual International Conference of the IEEE Engineer-
ing in Medicine and Biology Society (EMBC), pages
5937–5940. IEEE.
Yu, W., Chen, H., and Wang, L. (2019). Dense attentional
network for pancreas segmentation in abdominal ct
scans. In Proceedings of the 2nd International Con-
ference on Artificial Intelligence and Pattern Recogni-
tion, pages 83–87.
Zhang, D., Zhang, J., Zhang, Q., Han, J., Zhang, S., and
Han, J. (2021a). Automatic pancreas segmentation
based on lightweight dcnn modules and spatial prior
propagation. Pattern Recognition, 114:107762.
Zhang, Y., Wu, J., Liu, Y., Chen, Y., Chen, W., Wu, E. X.,
Li, C., and Tang, X. (2021b). A deep learning frame-
work for pancreas segmentation with multi-atlas reg-
istration and 3d level-set. Medical Image Analysis,
68:101884.
Zhao, H., Shi, J., Qi, X., Wang, X., and Jia, J. (2017).
Pyramid scene parsing network. In Proceedings of
the IEEE conference on computer vision and pattern
recognition, pages 2881–2890.
Zhao, N., Tong, N., Ruan, D., and Sheng, K. (2019). Fully
automated pancreas segmentation with two-stage 3d
convolutional neural networks. In Medical Im-
age Computing and Computer Assisted Intervention–
MICCAI 2019: 22nd International Conference, Shen-
zhen, China, October 13–17, 2019, Proceedings, Part
II 22, pages 201–209. Springer.
Zheng, H., Chen, Y., Yue, X., Ma, C., Liu, X., Yang, P.,
and Lu, J. (2020). Deep pancreas segmentation with
uncertain regions of shadowed sets. Magnetic Reso-
nance Imaging, 68:45–52.
Zhu, Z., Liu, C., Yang, D., Yuille, A., and Xu, D. (2019). V-
nas: Neural architecture search for volumetric medical
image segmentation. In 2019 International conference
on 3d vision (3DV), pages 240–248. IEEE.
Selection of Backbone for Feature Extraction with U-Net in Pancreas Segmentation
829