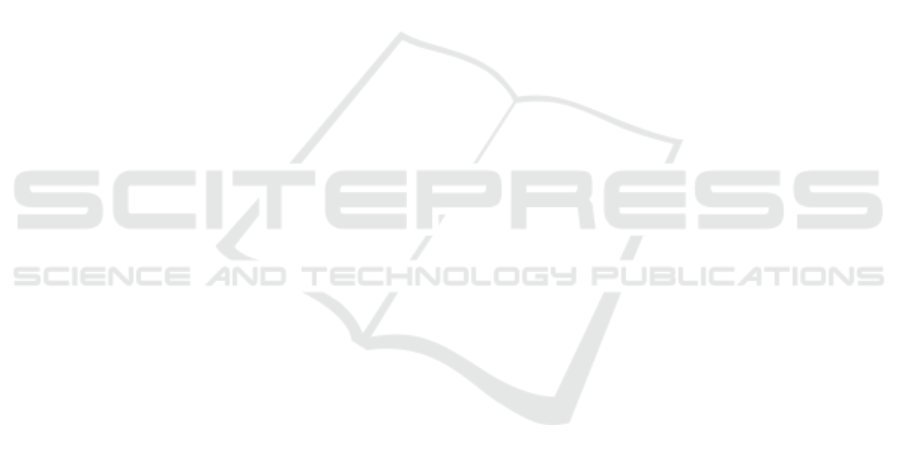
Conf. Knowledge Discovery and Data Mining, KDD,
pages 226–231. AAAI Press.
Gooch, A. A., Long, J., Ji, L., Estey, A., and Gooch, B.
(2010). Viewing progress in non-photorealistic ren-
dering through heinlein’s lens. In Proc. 8th Int. Symp.
Non-Photorealistic Animation and Rendering, NPAR,
pages 165–171. ACM.
Hao, W., Zuo, Z., and Liang, W. (2022). Structure-based
street tree extraction from mobile laser scanning point
clouds. In Proc. 5th Int. Conf. Image and Graphics
Processing,ICIGP, pages 373–379. ACM.
Hertzmann, A. (1998). Painterly rendering with curved
brush strokes of multiple sizes. In Proc. 25th Annu.
Conf. Computer Graphics and Interactive Techniques,
SIGGRAPH, pages 453–460. ACM.
H
¨
ollein, L., Johnson, J., and Nießner, M. (2022).
StyleMesh: Style transfer for indoor 3D scene recon-
structions. In Proc. IEEE/CVF Conf. Computer Vision
and Pattern Recognition, CVPR, pages 6198–6208.
Horaud, R., Hansard, M. E., Evangelidis, G. D., and
M
´
enier, C. (2016). An overview of depth cameras and
range scanners based on time-of-flight technologies.
Mach. Vis. Appl., 27(7):1005–1020.
Jing, Y., Yang, Y., Feng, Z., Ye, J., Yu, Y., and Song, M.
(2020). Neural style transfer: A review. IEEE Trans.
Vis. Comput. Graph., 26(11):3365–3385.
Kornilov, A. S. and Safonov, I. V. (2018). An overview of
watershed algorithm implementations in open source
libraries. J. Imaging, 4(10):123.
Kyprianidis, J. E., Collomosse, J., Wang, T., and Isenberg,
T. (2013). State of the ”art”: A taxonomy of artis-
tic stylization techniques for images and video. IEEE
Trans. Vis. Comput. Graph., 19(5):866–885.
K
¨
olle, M., Laupheimer, D., Schmohl, S., Haala, N., Rot-
tensteiner, F., Wegner, J. D., and Ledoux, H. (2021).
The Hessigheim 3D (H3D) benchmark on semantic
segmentation of high-resolution 3D point clouds and
textured meshes from UAV LiDAR and multi-view-
stereo. ISPRS J. Photogramm. Remote Sens., 1:11.
Li, Y., Ma, L., Zhong, Z., Liu, F., Chapman, M. A., Cao, D.,
and Li, J. (2021). Deep learning for lidar point clouds
in autonomous driving: A review. IEEE Trans. Neural
Networks Learn. Syst., 32(8):3412–3432.
Liu, X.-C., Cheng, M.-M., Lai, Y.-K., and Rosin, P. L.
(2017). Depth-aware neural style transfer. In Proc.
15th Int. Symp. Non-Photorealistic Animation and
Rendering, NPAR, pages 4:1–4:10. ACM.
Luo, H., Khoshelham, K., Chen, C., and He, H. (2021).
Individual tree extraction from urban mobile laser
scanning point clouds using deep pointwise direction
embedding. ISPRS J. Photogramm. Remote Sens.,
175:326–339.
Mirzaei, K., Arashpour, M., Asadi, E., Masoumi, H., Bai,
Y., and Behnood, A. (2022). 3D point cloud data pro-
cessing with machine learning for construction and
infrastructure applications: A comprehensive review.
Adv. Eng. Informatics, 51:101501.
Mu, F., Wang, J., Wu, Y., and Li, Y. (2022). 3D photo
stylization: Learning to generate stylized novel views
from a single image. In Proc. IEEE/CVF Conf. Com-
puter Vision and Pattern Recognition, CVPR, pages
16273–16282.
Rabbani, T., van den Heuvel, F., and Vosselman, G. (2006).
Segmentation of point clouds using smoothness con-
straints. In Proc. ISPRS Commission V Symposium,
pages 248–253.
Ribes, A. and Boucheny, C. (2011). Eye-dome lighting: A
non-photorealistic shading technique. Kitware Source
Quarterly Magazine, 7.
Richter, R., Discher, S., and D
¨
ollner, J. (2015). Out-of-
core visualization of classified 3D point clouds. In 3D
Geoinformation Science: Selected Papers of the 3D
GeoInfo 2014, pages 227–242. Springer.
Scheiblauer, C. (2014). Interactions with gigantic point
clouds. PhD thesis, Institute of Computer Graphics
and Algorithms, Vienna University of Technology.
Semmo, A., Limberger, D., Kyprianidis, J. E., and D
¨
ollner,
J. (2016). Image stylization by interactive oil paint
filtering. Comput. Graph., 55:157–171.
Shekhar, S., Reimann, M., Mayer, M., Semmo, A., Pase-
waldt, S., D
¨
ollner, J., and Trapp, M. (2021). Interac-
tive photo editing on smartphones via intrinsic decom-
position. Comput. Graph. Forum, 40(2):497–510.
Thomas, H., Qi, C. R., Deschaud, J.-E., Marcotegui, B.,
Goulette, F., and Guibas, L. J. (2019). KPConv: Flex-
ible and deformable convolution for point clouds. In
Proc. IEEE/CVF Int. Conf. Computer Vision ICCV,
pages 6410–6419.
Wagner, R., Wegen, O., Limberger, D., D
¨
ollner, J., and
Trapp, M. (2022). A non-photorealistic rendering
technique for art-directed hatching of 3D point clouds.
In Proc. 17th Int. Joint Conf. Computer Vision, Imag-
ing and Computer Graphics Theory and Applications,
VISIGRAPP, pages 220–227. SCITEPRESS.
Wang, W., Yu, R., Huang, Q., and Neumann, U.
(2018). SGPN: Similarity group proposal network
for 3D point cloud instance segmentation. In Proc.
IEEE/CVF Conf. Computer Vision and Pattern Recog-
nition, CVPR, pages 2569–2578.
Wegen, O., D
¨
ollner, J., Wagner, R., Limberger, D., Richter,
R., and Trapp, M. (2022). Non-photorealistic render-
ing of 3D point clouds for cartographic visualization.
Abstr. Int. Cartogr. Assoc., 5:161.
Weinmann, M., Jutzi, B., Hinz, S., and Mallet, C. (2015).
Semantic point cloud interpretation based on optimal
neighborhoods, relevant features and efficient classi-
fiers. ISPRS J. Photogramm. Remote Sens., 105:286–
304.
Westoby, M. J., Brasington, J., Glasser, N. F., Hambrey,
M. J., and Reynolds, J. M. (2012). ‘Structure-
from-Motion’ photogrammetry: A low-cost, effective
tool for geoscience applications. Geomorphology,
179:300–314.
Winnem
¨
oller, H., Olsen, S. C., and Gooch, B. (2006).
Real-time video abstraction. ACM Trans. Graph.,
25(3):1221–1226.
Xie, Y., Tian, J., and Zhu, X. X. (2020). Linking points
with labels in 3D: A review of point cloud seman-
tic segmentation. IEEE Geosci. Remote Sens. Mag.,
8(4):38–59.
Xu, H., Gossett, N., and Chen, B. (2004). PointWorks: Ab-
straction and rendering of sparsely scanned outdoor
environments. In Proc. 15th EG Workshop on Ren-
dering Techniques, EGWR, pages 45–52. Eurograph-
ics Association.
Zwicker, M., Pfister, H., van Baar, J., and Gross, M. H.
(2001). Surface splatting. In Proc. 28th Annu. Conf.
Computer Graphics and Interactive Techniques, SIG-
GRAPH, pages 371–378. ACM.
GRAPP 2024 - 19th International Conference on Computer Graphics Theory and Applications
200