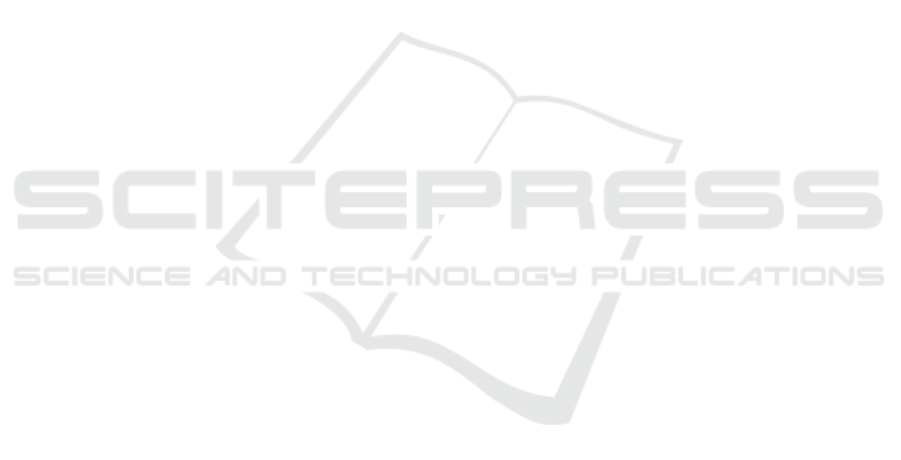
with and without cleft lip and/or palate: A cross-
sectional study. The Cleft palate-craniofacial jour-
nal : official publication of the American Cleft Palate-
Craniofacial Association, 58(9):1150—1159.
Chen, S., Atapour-Abarghouei, A., Kerby, J., Ho, E. S. L.,
Sainsbury, D. C. G., Butterworth, S., and Shum, H.
P. H. (2022). A feasibility study on image inpaint-
ing for non-cleft lip generation from patients with cleft
lip. In 2022 IEEE-EMBS International Conference on
Biomedical and Health Informatics (BHI), pages 01–
04.
Cobourne, M. T. (2004). The complex genetics of cleft
lip and palate. European Journal of Orthodontics,
26(1):7–16.
Daher, R., Vasconcelos, F., and Stoyanov, D. (2022). A
temporal learning approach to inpainting endoscopic
specularities and its effect on image correspondence.
Deng, Y., Hui, S., Meng, R., Zhou, S., and Wang, J. (2022).
Hourglass attention network for image inpainting. In
Computer Vision – ECCV 2022: 17th European Con-
ference, Tel Aviv, Israel, October 23–27, 2022, Pro-
ceedings, Part XVIII, page 483–501, Berlin, Heidel-
berg. Springer-Verlag.
Duggal, I., Talwar, A., Duggal, R., Chaudhari, P. K., and
Samrit, V. (2023). Comparative evaluation of na-
solabial appearance of unilateral cleft lip and palate
patients by professional, patient and layperson using
2 aesthetic scoring systems: A cross sectional study.
Orthodontics & Craniofacial Research, 26(4):660–
666.
He, K., Zhang, X., Ren, S., and Sun, J. (2015). Deep resid-
ual learning for image recognition.
Kammoun, A., Slama, R., Tabia, H., Ouni, T., and Abid,
M. (2022). Generative Adversarial Networks for Face
Generation: A Survey. ACM Computing Surveys,
55(5):1–37.
Karras, T., Laine, S., and Aila, T. (2018). A style-based
generator architecture for generative adversarial net-
works. CoRR, abs/1812.04948.
Karras, T., Laine, S., Aittala, M., Hellsten, J., Lehtinen, J.,
and Aila, T. (2020). Analyzing and improving the im-
age quality of stylegan.
King, D. E. (2009). Dlib-ml: A machine learning toolkit.
The Journal of Machine Learning Research, 10:1755–
1758.
Koch, G., Zemel, R., and Salakhutdinov, R. (2015).
Siamese neural networks for one-shot image recogni-
tion.
Li, Y., Cheng, J., Mei, H., Ma, H., Chen, Z., and Li, Y.
(2019). Clpnet: Cleft lip and palate surgery support
with deep learning. In 2019 41st Annual International
Conference of the IEEE Engineering in Medicine and
Biology Society (EMBC), pages 3666–3672.
Lin, G., Kim, P.-J., Baek, S.-H., Kim, H.-G., Kim, S.-W.,
and Chung, J.-H. (2021). Early prediction of the need
for orthognathic surgery in patients with repaired uni-
lateral cleft lip and palate using machine learning and
longitudinal lateral cephalometric analysis data. Jour-
nal of Craniofacial Surgery, 32(2):616–620.
Liu, Y., Shi, H., Shen, H., Si, Y., Wang, X., and Mei, T.
(2020). A new dataset and boundary-attention se-
mantic segmentation for face parsing. In AAAI, pages
11637–11644.
Liu, Z., Luo, P., Wang, X., and Tang, X. (2015). Deep learn-
ing face attributes in the wild. In Proceedings of In-
ternational Conference on Computer Vision (ICCV).
Medvedev, I., Shadmand, F., and Gonc¸alves, N. (2023).
Young labeled faces in the wild (ylfw): A dataset for
children faces recognition.
Mosmuller, D. G., Mennes, L. M., Prahl, C., Kramer, G. J.,
Disse, M. A., Couwelaar, G. M. V., Frank, B. N., and
Griot, J. D. (2016). The Development of the Cleft
Aesthetic Rating Scale: A New Rating Scale for the
Assessment of Nasolabial Appearance in Complete
Unilateral Cleft Lip and Palate Patients. The Cleft
Palate-Craniofacial Journal, 54(5):555–561.
Paszke, A., Gross, S., Chintala, S., Chanan, G., Yang, E.,
DeVito, Z., Lin, Z., Desmaison, A., Antiga, L., and
Lerer, A. (2017). Automatic differentiation in Py-
Torch. NIPS.
Pei, M., Yan, B., Hao, H., and Zhao, M. (2023). Person-
specific face spoofing detection based on a siamese
network. Pattern Recognition, 135:109148.
Ronneberger, O., P.Fischer, and Brox, T. (2015). U-
net: Convolutional networks for biomedical image
segmentation. In Medical Image Computing and
Computer-Assisted Intervention (MICCAI), volume
9351 of LNCS, pages 234–241. Springer. (available
on arXiv:1505.04597 [cs.CV]).
Shkoukani, M., Chen, M., and Vong, A. (2013). Cleft lip –
a comprehensive review. Frontiers in pediatrics, 1:53.
Shorfuzzaman, M. and Hossain, M. S. (2021). Metacovid:
A siamese neural network framework with contrastive
loss for n-shot diagnosis of covid-19 patients. Pattern
recognition, 113:107700.
Tran, M.-T., Kim, S.-H., Yang, H.-J., and Lee, G.-S.
(2021). Multi-task learning for medical image inpaint-
ing based on organ boundary awareness. Applied Sci-
ences, 11(9).
Vyas, T., Gupta, P., Kumar, S., Gupta, R., Gupta, T., and
Singh, H. (2020). Cleft of lip and palate: A re-
view. Journal of Family Medicine and Primary Care,
9(6):2621.
Wadde, D., Chowdhar, D., Venkatakrishnan, D., Ghodake,
D., Sachdev, S., and Chhapane, D. (2022). Protocols
in the management of cleft lip and palate: A system-
atic review. Journal of Stomatology, Oral and Max-
illofacial Surgery, 124.
Wang, X., Yu, Z., Wang, L., and Zheng, P. (2022). An
enhanced priori knowledge gan for ct images gener-
ation of early lung nodules with small-size labelled
samples. Oxid Med Cell Longev, 2022:2129303.
Yilmaz, H. N., Ozbilen, E. O., and and, T. U. (2019). The
prevalence of cleft lip and palate patients: A single-
center experience for 17 years. Turkish Journal of Or-
thodontics, 32(3):139–144.
Enhancing Surgical Visualization: Feasibility Study on GAN-Based Image Generation for Post Operative Cleft Palate Images
945