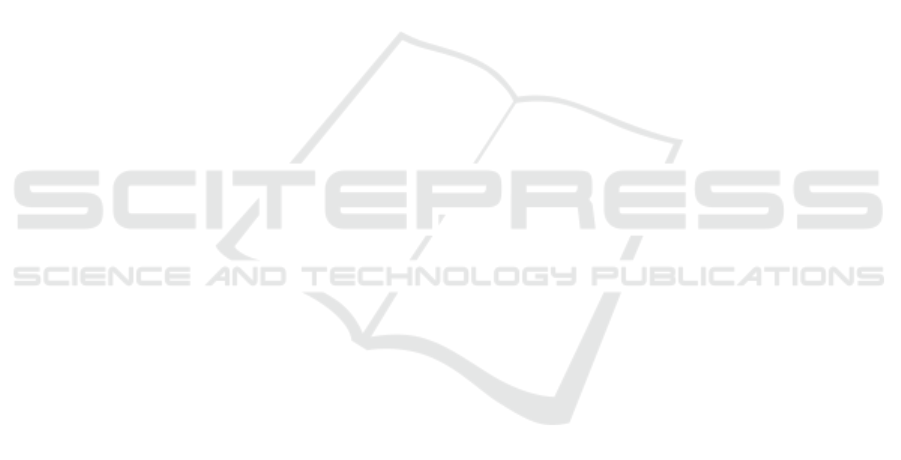
4 CONCLUSIONS
In this paper, we proposed a new method for sign
language recognition that processes time-domain in-
formation on the frequency-domain by representing
videos as 3D amplitude tensors using the 3D Fast
Fourier Transform (3D-FFT) and effectively compar-
ing them in the Product Grassmann Manifold (PGM).
Focusing only on the amplitude spectrum, we ob-
tain features robust to time deviations. Furthermore,
PGM can effectively represent and compare the ten-
sor structures as subspaces generated from each ten-
sor mode while preserving the temporal information
due to the unfolding operation. Therefore, we estab-
lished a simple yet powerful subspace representation
that considers temporal information. Experimental
results showed that our method can significantly im-
prove performance over other subspace-based meth-
ods. In the future, we are interested in verifying the
efficacy of our method in other action recognition
tasks.
ACKNOWLEDGMENTS
This work was supported by JSPS KAKENHI Grant
Number 21K18481. The authors thank the Tsukuba
University of Technology students for their help in
collecting our TNSD data set.
REFERENCES
Batalo, B., Souza, L. S., Gatto, B. B., Sogi, N., and Fukui,
K. (2022). Temporal-stochastic tensor features for
action recognition. Machine Learning with Applica-
tions, 10:100407.
Beleza, S. R. and Fukui, K. (2021). Slow feature sub-
space for action recognition. In Pattern Recognition.
ICPR International Workshops and Challenges: Vir-
tual Event, January 10–15, 2021, Proceedings, Part
III, pages 702–716.
Beleza, S. R. A., Shimomoto, E. K., Souza, L. S., and
Fukui, K. (2023). Slow feature subspace: A video
representation based on slow feature analysis for ac-
tion recognition. Machine Learning with Applica-
tions, 14:100493.
Fukui, K. (2014). Subspace methods. In Computer Vision,
A Reference Guide, pages 777–781.
Fukui, K. and Maki, A. (2015). Difference subspace and
its generalization for subspace-based methods. IEEE
Transactions on Pattern Analysis and Machine Intel-
ligence, 37(11):2164–2177.
Iwashita, Y., Kakeshita, M., Sakano, H., and Kurazume,
R. (2017). Making gait recognition robust to speed
changes using mutual subspace method. In 2017 IEEE
International Conference on Robotics and Automation
(ICRA), pages 2273–2278.
Iwashita, Y., Sakano, H., and Kurazume, R. (2015). Gait
recognition robust to speed transition using mutual
subspace method. In International Conference on Im-
age Analysis and Processing, pages 141–149.
Jaouedi, N., Boujnah, N., and Bouhlel, M. S. (2020). A new
hybrid deep learning model for human action recogni-
tion. Journal of King Saud University-Computer and
Information Sciences, 32(4):447–453.
Liu, T., Zhou, W., and Li, H. (2016). Sign language recog-
nition with long short-term memory. In 2016 IEEE
international conference on image processing (ICIP),
pages 2871–2875.
Lui, Man, Y., Beveridge, Ross, J., Kirby, and Michael
(2010). Action classification on product manifolds.
In 2010 IEEE Computer Society Conference on Com-
puter Vision and Pattern Recognition, pages 833–839.
Lui, Y. M. (2012). Human gesture recognition on prod-
uct manifolds. The Journal of Machine Learning Re-
search, 13(1):3297–3321.
Mahbub, U., Imtiaz, H., Roy, T., Rahman, M. S., and Ahad,
M. A. R. (2013). A template matching approach of
one-shot-learning gesture recognition. Pattern Recog-
nition Letters, 34(15):1780–1788.
Modler, P. and Myatt, T. (2007). Image features based on
two-dimensional fft for gesture analysis and recogni-
tion. SMC07, Leykada, Greece.
Peris, M. and Fukui, K. (2012). Both-hand gesture recogni-
tion based on komsm with volume subspaces for robot
teleoperation. In 2012 IEEE International Conference
on Cyber Technology in Automation, Control, and In-
telligent Systems (CYBER), pages 191–196.
Sakai, A., Sogi, N., and Fukui, K. (2019). Gait recognition
based on constrained mutual subspace method with
cnn features. In 2019 16th international conference
on machine vision applications (MVA), pages 1–6.
Simonyan, K. and Zisserman, A. (2014). Very deep con-
volutional networks for large-scale image recognition.
arXiv preprint arXiv:1409.1556.
Suryanto, C. H., Xue, J.-H., and Fukui, K. (2016). Random-
ized time warping for motion recognition. Image and
Vision Computing, 54:1–11.
Tanaka, S., Okazaki, A., Kato, N., Hino, H., and Fukui,
K. (2016). Spotting fingerspelled words from sign
language video by temporally regularized canonical
component analysis. In 2016 IEEE International Con-
ference on Identity, Security and Behavior Analysis
(ISBA), pages 1–7.
Tufek, N., Yalcin, M., Altintas, M., Kalaoglu, F., Li, Y.,
and Bahadir, S. K. (2019). Human action recognition
using deep learning methods on limited sensory data.
IEEE Sensors Journal, 20(6):3101–3112.
Wiskott, L. and Sejnowski, T. J. (2002). Slow feature anal-
ysis: Unsupervised learning of invariances. Neural
computation, 14(4):715–770.
Wong, Y.-C. (1967). Differential geometry of grassmann
manifolds. Proceedings of the National Academy of
Sciences, 57(3):589–594.
Yamaguchi, O., Fukui, K., and Maeda, K. (1998). Face
recognition using temporal image sequence. In Pro-
ceedings third IEEE international conference on au-
tomatic face and gesture recognition, pages 318–323.
Sign Language Recognition Based on Subspace Representations in the Spatio-Temporal Frequency Domain
159