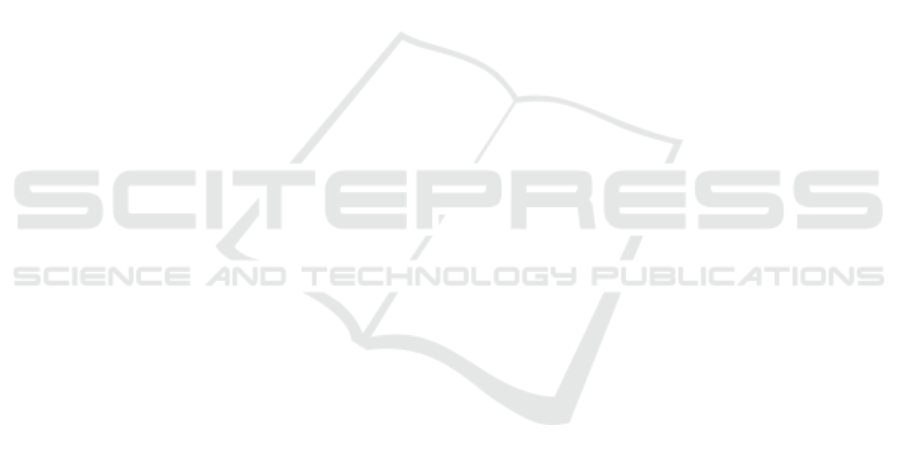
their Italian versions: CBA-VE, PQ-16, ERIraos-CL,
GAF, and SOFAS. These cover various psychological
and psychiatric aspects and serve as essential tools in
assessing and treating patients.
An issue encountered is certainly the lack of a
higher quantity of data to perform a consistent fine-
tuning of the convolutional networks used. For this
reason, during the process, the choice of using a
Transfer Learning technique, without fine-tuning
the CNNs (GoogleNet, VGG16 and Resnet18), has
been taken. Additionally, due to the highly sensi-
tive nature of the information contained in this type of
questionnaire, a labelled dataset serving as a ground
truth for accurate assessment was not found. There-
fore, the validation of the extraction phase lacked a
measurable metric.
Currently, the tool operates only on structured
questionnaires, exclusively recognising manually
filled check boxes. Nonetheless, we intend to include
a handwriting recognition component in the future,
allowing for the digitisation of less structured ques-
tionnaires containing handwritten sections. Thanks to
the collaboration with CPS “Giovani di Niguarda” we
will test the effectiveness of this solution on a wider
number of administrations, further expanding its ap-
plicability and assessing its performance.
This solution will be integrated into a proprietary
digital platform designed to oversee the comprehen-
sive management of psychiatric and psychological pa-
tient treatment courses. Although the digital platform
won’t be open-source, the PANTHER tool is publicly
available for further research and to be freely inte-
grated into other solutions.
REFERENCES
Alzubaidi, L., Zhang, J., Humaidi, A. J., Al-Dujaili, A.,
Duan, Y., Al-Shamma, O., Santamar
´
ıa, J., Fadhel,
M. A., Al-Amidie, M., and Farhan, L. (2021). Re-
view of deep learning: Concepts, cnn architectures,
challenges, applications, future directions. Journal of
big Data, 8:1–74.
American Psychiatric Association (2000). Manuale diag-
nostico e statistico dei disturbi mentali, chapter Valu-
tazione Multiassiale. Masson, 4th edition. text rev.
Basha, S. S., Dubey, S. R., Pulabaigari, V., and Mukherjee,
S. (2020). Impact of fully connected layers on per-
formance of convolutional neural networks for image
classification. Neurocomputing, 378:112–119.
Biau, G. and Scornet, E. (2016). A random forest guided
tour. Test, 25:197–227.
Browne, M. W. (2000). Cross-validation methods. Journal
of mathematical psychology, 44(1):108–132.
Butcher, J. N., Perry, J. N., and Atlis, M. M. (2000). Valid-
ity and utility of computer-based test interpretation.
Psychological assessment, 12(1):6.
Cao, H. and Govindaraju, V. (2007). Vector model based
indexing and retrieval of handwritten medical forms.
In Ninth International Conference on Document Anal-
ysis and Recognition (ICDAR 2007), volume 1, pages
88–92. IEEE.
Chabert, C., Collado, A., Cheval, B., and Hue, O. (2021).
and-pencil questionnaires analysis: a new automated
technique to reduce analysis time and errors. bioRxiv,
pages 2021–03.
Coons, S. J., Gwaltney, C. J., Hays, R. D., Lundy, J. J.,
Sloan, J. A., Revicki, D. A., Lenderking, W. R.,
Cella, D., and Basch, E. (2009). Recommendations
on evidence needed to support measurement equiv-
alence between electronic and paper-based patient-
reported outcome (pro) measures: Ispor epro good re-
search practices task force report. Value in Health,
12(4):419–429.
Deng, J., Dong, W., Socher, R., Li, L.-J., Li, K., and Fei-
Fei, L. (2009). Imagenet: A large-scale hierarchical
image database. In 2009 IEEE conference on com-
puter vision and pattern recognition, pages 248–255.
Ieee.
Du, X., Sun, Y., Song, Y., Sun, H., and Yang, L. (2023). A
comparative study of different cnn models and transfer
learning effect for underwater object classification in
side-scan sonar images. Remote Sensing, 15(3):593.
Engan, H., Hilmarsen, C., Sittlinger, S., Sandmæl, J. A.,
Skanke, F., and Oldervoll, L. M. (2016). Are web-
based questionnaires accepted in patients attending re-
habilitation? Disability and Rehabilitation, 38:2406–
2412.
Franke, G. H. (1997). ”the whole is more than the sum of its
parts”: the effects of grouping and randomizing items
on the reliability and validity of questionnaires. Euro-
pean Journal of Psychological Assessment, 13:67–74.
Groth-Marnat, G. (1999). Current status and future direc-
tions of psychological assessment: Introduction. Jour-
nal of Clinical Psychology, 55(7):781–785.
He, K., Zhang, X., Ren, S., and Sun, J. (2016). Deep resid-
ual learning for image recognition. In Proceedings of
the IEEE conference on computer vision and pattern
recognition, pages 770–778.
Holl
¨
andare, F., Andersson, G., Engstr
¨
om, I., et al. (2010). A
comparison of psychometric properties between inter-
net and paper versions of two depression instruments
(bdi-ii and madrs-s) administered to clinic patients.
Journal of medical Internet research, 12(5):e1392.
Lanyon, R. I. (2011). Handbook of psychological assess-
ment, gary groth-marnat, wiley, new york (2009), 752
pp.
Lorenzo, P., Silvia, A., Federica, P., Sara, G., Ilaria, S.,
Pupo, S., and Raballo, A. (2018). The italian ver-
sion of the 16-item prodromal questionnaire (ipq-16):
Field-test and psychometric features. Schizophrenia
Research, 199:353–360.
Maniar, S., Parmani, J., Bodke, M., and Saxena, K. (2021).
Generation and grading of arduous mcqs using nlp
ICT4AWE 2024 - 10th International Conference on Information and Communication Technologies for Ageing Well and e-Health
150