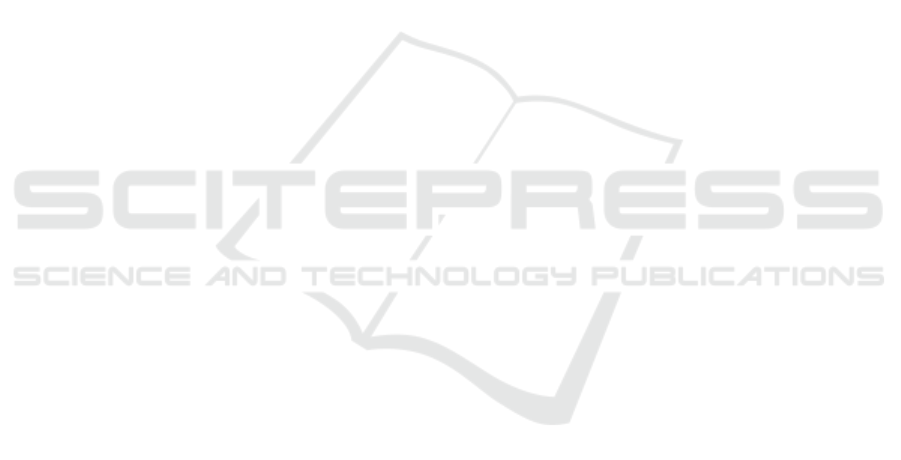
This confirm that transfer learning is useful, even
with networks trained for a very different problem,
opening a new strategy to overcome the problem of
the lack of samples for training in heath related
applications.
Detecting PD in its early stages is one of the
challenges facing the world, as it is essential for
successful treatment and improving patients' quality
of life, and we must race against time to find ways to
treat this dangerous disease. The YAMNet-based
method offers an affordable and non-invasive
solution for the detection of Parkinson's disease, thus
reducing examination time and workload in hospitals
and healthcare centres. In addition, it can be used in
real time, allowing PD patients to be continuously
monitored using wearable technology, and to
diagnose the patient earlier to offer tailored treatment
options. It should be noted that the study had
limitations, including the small sample size, which
made training and testing more complicated and
required the use of the k-fold technique, and the fact
that the database belonged to a restricted age group.
Moreover, in order to enhance diagnostic
precision and formulate a model for identifying and
categorizing other diseases that are also related to
analysing brain signals, it is crucial to verify the
efficacy of our technique with a wider range of
population samples and a larger dataset and variety.
Additionally, the implementation of this approach on
wearable devices to enable continuous monitoring of
PD patients poses several challenges that call for
further research.
ACKNOWLEDGEMENTS
This paper is part of the project with reference
PID2021-129043OB-I00, funded by
MCIN/AEI/10.13039/501100011033/FEDER, EU.
REFERENCES
Balestrino, R., & Schapira, A. H. V. (2020). Parkinson
disease. European Journal of Neurology, 27(1), 27–42.
https://doi.org/10.1111/ene.14108
Dentamaro, V., Giglio, P., Impedovo, D., & Pirlo, G.
(2021). Benchmarking of shallow learning and deep
learning techniques with transfer learning for
neurodegenerative disease assessment through
handwriting. International Conference on Document
Analysis and Recognition, 7–20.
Dentamaro, V., Impedovo, D., & Pirlo, G. (2020). Gait
Analysis for Early Neurodegenerative Diseases
Classification through the Kinematic Theory of Rapid
Human Movements. IEEE Access, 8, 193966–193980.
https://doi.org/10.1109/ACCESS.2020.3032202
Dentamaro, V., Impedovo, D., & Pirlo, G. (2021). An
analysis of tasks and features for neuro-degenerative
disease assessment by handwriting. International
Conference on Pattern Recognition, 536–545.
Gattulli, V., Impedovo, D., Pirlo, G., & Semeraro, G.
(2022). Early Dementia Identification: On the Use of
Random Handwriting Strokes. International
Graphonomics Conference, 285–300.
Impedovo, D., & Pirlo, G. (2018). Dynamic Handwriting
Analysis for the Assessment of Neurodegenerative
Diseases: A Pattern Recognition Perspective. IEEE
Reviews in Biomedical Engineering, 12, 209–220.
https://doi.org/10.1109/RBME.2018.2840679
Khan, P., Kader, M. F., Islam, S. M. R., Rahman, A. B.,
Kamal, M. S., Toha, M. U., & Kwak, K. S. (2021).
Machine Learning and Deep Learning Approaches for
Brain Disease Diagnosis: Principles and Recent
Advances. IEEE Access, 9, 37622–37655.
https://doi.org/10.1109/ACCESS.2021.3062484
Khosla, A., Khandnor, P., & Chand, T. (2020). A
comparative analysis of signal processing and
classification methods for different applications based
on EEG signals. Biocybernetics and Biomedical
Engineering, 40(2), 649–690.
https://doi.org/10.1016/j.bbe.2020.02.002
Lang, A. E., & Espay, A. J. (2018). Disease Modification
in Parkinson’s Disease: Current Approaches,
Challenges, and Future Considerations. Movement
Disorders, 33(5), 660–677. https://doi.org/10.1002/
mds.27360
Lee, S., Hussein, R., & McKeown, M. J. (2019). A deep
convolutional-recurrent neural network architecture for
Parkinson’s disease EEG classification. GlobalSIP
2019 - 7th IEEE Global Conference on Signal and
Information Processing, Proceedings, 14–17.
https://doi.org/10.1109/GlobalSIP45357.2019.896930
9
Lee, S., Hussein, R., Ward, R., Jane Wang, Z., &
McKeown, M. J. (2021). A convolutional-recurrent
neural network approach to resting-state EEG
classification in Parkinson’s disease. Journal of
Neuroscience Methods, 361, 109282.
https://doi.org/10.1016/j.jneumeth.2021.109282
Loh, H. W., Ooi, C. P., Palmer, E., Barua, P. D., Dogan, S.,
Tuncer, T., Baygin, M., & Rajendra Acharya, U.
(2021). Gaborpdnet: Gabor transformation and deep
neural network for parkinson’s disease detection using
eeg signals. Electronics (Switzerland), 10(14).
https://doi.org/10.3390/electronics10141740
Maitín, A. M., García-Tejedor, A. J., & Muñoz, J. P. R.
(2020). Machine learning approaches for detecting
parkinson’s disease from eeg analysis: A systematic
review. Applied Sciences (Switzerland), 10(23), 1–21.
https://doi.org/10.3390/app10238662
Maitin, A. M., Pablo, J., & Muñoz, R. (2022). applied
sciences Survey of Machine Learning Techniques in the
Analysis of EEG Signals for Parkinson ’ s Disease : A
Systematic Review. Applied Sciences (Switzerland),
Unveiling the Power of EEG Signals: Parkinson’s Disease Identification via Yet Another Mobile Network (YAMNet)
983