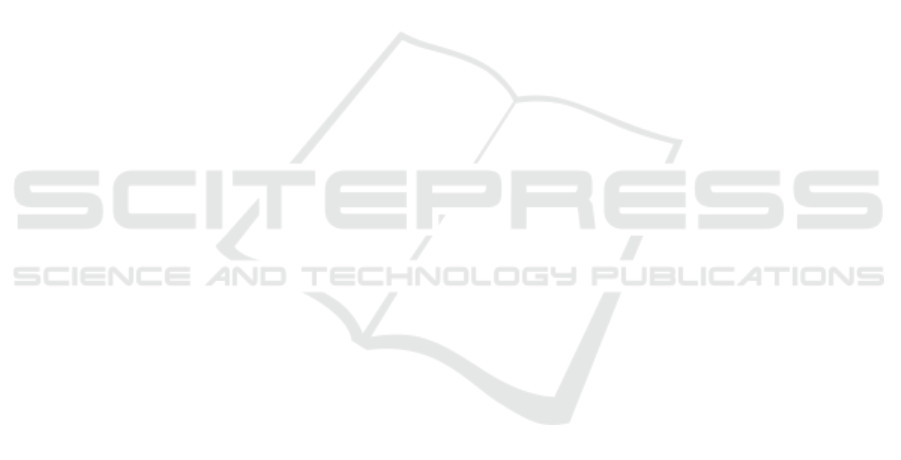
Du
ˇ
sek, O. and Kasner, Z. (2020). Evaluating semantic accu-
racy of data-to-text generation with natural language
inference. In Davis, B., Graham, Y., Kelleher, J., and
Sripada, Y., editors, Proceedings of the 13th Interna-
tional Conference on Natural Language Generation,
pages 131–137, Dublin, Ireland. Association for Com-
putational Linguistics.
Elhadad, M. (1991). Generating adjectives to express the
speaker’s argumentative intent. In Proceedings of the
Ninth National Conference on Artificial Intelligence -
Volume 1, AAAI’91, page 98–103. AAAI Press.
Goswamy, T., Singh, I., Barkati, A., and Modi, A. (2020).
Adapting a language model for controlled affective
text generation. In Scott, D., Bel, N., and Zong, C.,
editors, Proceedings of the 28th International Confer-
ence on Computational Linguistics, pages 2787–2801,
Barcelona, Spain (Online). International Committee
on Computational Linguistics.
Halliday, M. and Hasan, R. (1976). Cohesion in English.
Longman, London.
Ji, Z., Lee, N., Frieske, R., Yu, T., Su, D., Xu, Y., Ishii, E.,
Bang, Y. J., Madotto, A., and Fung, P. (2023). Survey
of hallucination in natural language generation. ACM
Computing Surveys, 55(12):1–38.
Kirchenbauer, J., Geiping, J., Wen, Y., Katz, J., Miers, I.,
and Goldstein, T. (2023). A watermark for large lan-
guage models.
Langner, M. and Klabunde, R. (2022). Realizing a denial
of expectation in pipelined neural data-to-text gener-
ation. In Confalonieri, R. and Porello, D., editors,
Proceedings of the 6th Workshop on Advances in Ar-
gumentation in Artificial Intelligence 2022 co-located
with the 21st International Conference of the Italian
Association for Artificial Intelligence (AIxIA 2022),
Udine, Italy, November 28, 2022, volume 3354 of
CEUR Workshop Proceedings. CEUR-WS.org.
Langner, M. and Klabunde, R. (2023). Validating predic-
tive models of evaluative language for controllable
Data2Text generation. In Keet, C. M., Lee, H.-
Y., and Zarrieß, S., editors, Proceedings of the 16th
International Natural Language Generation Confer-
ence, pages 313–322, Prague, Czechia. Association
for Computational Linguistics.
Mahamood, S., Reiter, E., and Mellish, C. (2007). A com-
parison of hedged and non-hedged nlg texts. In Pro-
ceedings of the Eleventh European Workshop on Natu-
ral Language Generation, ENLG ’07, page 155–158,
USA. Association for Computational Linguistics.
Mitchell, E., Lee, Y., Khazatsky, A., Manning, C. D.,
and Finn, C. (2023). Detectgpt: Zero-shot machine-
generated text detection using probability curvature.
In Proceedings of the 40th International Conference
on Machine Learning, ICML’23, page 24950–24962.
JMLR.org.
Sadasivan, V. S., Kumar, A., Balasubramanian, S., Wang,
W., and Feizi, S. (2023). Can ai-generated text be re-
liably detected?
Santhanam, S. and Shaikh, S. (2019). Emotional neural lan-
guage generation grounded in situational contexts. In
Burtenshaw, B. and Manjavacas, E., editors, Proceed-
ings of the 4th Workshop on Computational Creativ-
ity in Language Generation, pages 22–27. Association
for Computational Linguistics, Tokyo, Japan.
Shen, X., Chang, E., Su, H., Niu, C., and Klakow, D.
(2020). Neural data-to-text generation via jointly
learning the segmentation and correspondence. In Ju-
rafsky, D., Chai, J., Schluter, N., and Tetreault, J.,
editors, Proceedings of the 58th Annual Meeting of
the Association for Computational Linguistics, pages
7155–7165, Online. Association for Computational
Linguistics.
Solaiman, I., Brundage, M., Clark, J., Askell, A., Herbert-
Voss, A., Wu, J., Radford, A., Krueger, G., Kim, J. W.,
Kreps, S., McCain, M., Newhouse, A., Blazakis, J.,
McGuffie, K., and Wang, J. (2019). Release strategies
and the social impacts of language models.
Thomson, C., Reiter, E., and Sripada, S. (2020). Sport-
Sett:basketball - a robust and maintainable data-set for
natural language generation. In S
´
anchez, D., Herv
´
as,
R., and Gatt, A., editors, Proceedings of the Work-
shop on Intelligent Information Processing and Nat-
ural Language Generation, pages 32–40, Santiago
de Compostela, Spain. Association for Computational
Lingustics.
Trnavac, R. and Taboada, M. (2012). The contribution of
nonveridical rhetorical relations to evaluation in dis-
course. Language Sciences, 3(34):301–318.
Wang, P., Li, L., Ren, K., Jiang, B., Zhang, D., and Qiu,
X. (2023). SeqXGPT: Sentence-level AI-generated
text detection. In Bouamor, H., Pino, J., and Bali,
K., editors, Proceedings of the 2023 Conference on
Empirical Methods in Natural Language Processing,
pages 1144–1156, Singapore. Association for Compu-
tational Linguistics.
Wiseman, S., Shieber, S., and Rush, A. (2017). Challenges
in data-to-document generation. In Proceedings of
the 2017 Conference on Empirical Methods in Nat-
ural Language Processing, pages 2253–2263, Copen-
hagen, Denmark. Association for Computational Lin-
guistics.
ICAART 2024 - 16th International Conference on Agents and Artificial Intelligence
654