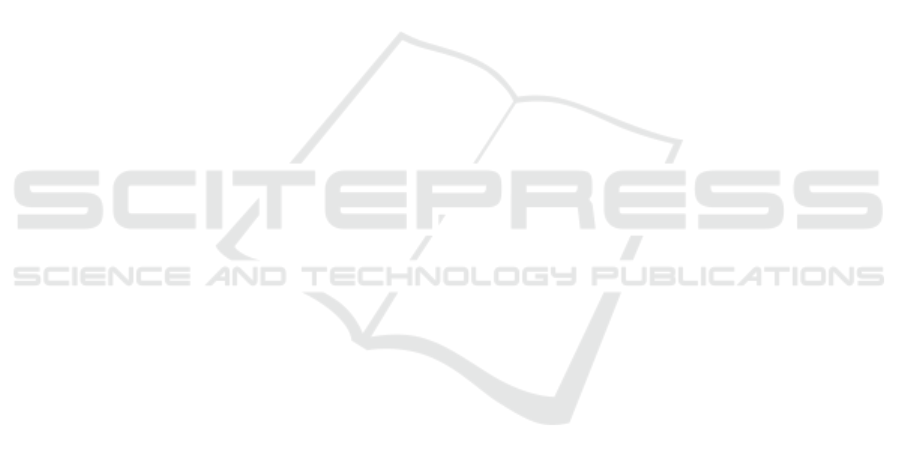
REFERENCES
Antwarg, L., Miller, R. M., Shapira, B., and Rokach, L.
(2021). Explaining anomalies detected by autoen-
coders using Shapley Additive Explanations[Formula
presented]. Expert Systems with Applications, 186.
Bahani, K., Moujabbir, M., and Ramdani, M. (2021). An
accurate fuzzy rule-based classification systems for
heart disease diagnosis. Scientific African, 14.
Chalabianloo, N., Can, Y. S., Umair, M., Sas, C., and Ersoy,
C. (2022). Application level performance evaluation
of wearable devices for stress classification with ex-
plainable AI. Pervasive and Mobile Computing, 87.
Chen, T. and Guestrin, C. (2016). XGBoost: A scalable tree
boosting system. In Proceedings of the ACM SIGKDD
International Conference on Knowledge Discovery
and Data Mining, volume 13-17-August-2016, pages
785–794. Association for Computing Machinery.
Dave, D., Naik, H., Singhal, S., and Patel, P. (2020). Ex-
plainable AI meets Healthcare: A Study on Heart Dis-
ease Dataset. Technical report.
Doran, D., Schulz, S., and Besold, T. R. (2017). What Does
Explainable AI Really Mean? A New Conceptualiza-
tion of Perspectives.
Greene, S., Thapliyal, H., and Caban-Holt, A. (2016). A
survey of affective computing for stress detection:
Evaluating technologies in stress detection for bet-
ter health. IEEE Consumer Electronics Magazine,
5(4):44–56.
Guidotti, R., Monreale, A., Ruggieri, S., Turini, F., Gian-
notti, F., and Pedreschi, D. (2018). A survey of meth-
ods for explaining black box models. ACM Computing
Surveys, 51(5).
Hosseini, S., Gottumukkala, R., Katragadda, S., Bhupati-
raju, R. T., Ashkar, Z., Borst, C. W., and Cochran,
K. (2022). A multimodal sensor dataset for continu-
ous stress detection of nurses in a hospital. Scientific
Data, 9(1).
Inam, R., Terra, A., Mujumdar, A., Fersman, E., and Feljan,
A. V. (2021). Explainable AI – how humans can trust
AI.
Linardatos, P., Papastefanopoulos, V., and Kotsiantis, S.
(2021). Explainable ai: A review of machine learn-
ing interpretability methods.
Lopez-Martinez, D., El-Haouij, N., and Picard, R. (2019).
Detection of Real-world Driving-induced Affective
State Using Physiological Signals and Multi-view
Multi-task Machine Learning.
Lundberg, S. and Lee, S.-I. (2017). A Unified Approach to
Interpreting Model Predictions.
Madanu, R., Abbod, M. F., Hsiao, F.-J., Chen, W.-T., and
Shieh, J.-S. (2022). Explainable AI (XAI) Applied
in Machine Learning for Pain Modeling: A Review.
Technologies, 10(3):74.
Mazzanti, S. (2020). SHAP Values Explained Exactly How
You Wished Someone Explained to You.
Miller, T. (2019). Explanation in artificial intelligence: In-
sights from the social sciences.
Montavon, G., Samek, W., and M
¨
uller, K. R. (2018). Meth-
ods for interpreting and understanding deep neural
networks.
Morales, A., Barbosa, M., Mor
´
as, L., Cazella, S. C., Sgobbi,
L. F., Sene, I., and Marques, G. (2022a). Occupational
stress monitoring using biomarkers and smartwatches:
A systematic review. Sensors, 22(17).
Morales, A. S., de Oliveira Ourique, F., Mor
´
as, L. D.,
Barbosa, M. L. K., and Cazella, S. C. (2022b). A
Biomarker-Based Model to Assist the Identification
of Stress in Health Workers Involved in Coping with
COVID-19, pages 485–500. Springer International
Publishing, Cham.
Morales, A. S., de Oliveira Ourique, F., Mor
´
as, L. D., and
Cazella, S. C. (2022c). Exploring Interpretable Ma-
chine Learning Methods and Biomarkers to Classify-
ing Occupational Stress of the Health Workers, pages
105–124. Springer International Publishing, Cham.
Pawar, U., O’shea, D., Rea, S., and O’reilly, R. (2020). Ex-
plainable AI in Healthcare. Technical report.
Picard, R. W. (2000). Affective computing. MIT press.
Potts, S. R., McCuddy, W. T., Jayan, D., and Porcelli, A. J.
(2019). To trust, or not to trust? individual differ-
ences in physiological reactivity predict trust under
acute stress. Psychoneuroendocrinology, 100:75–84.
Singh, A., Thakur, N., and Sharma, A. (2016). A Review of
Supervised Machine Learning Algorithms.
Tseng, P. Y., Chen, Y. T., Wang, C. H., Chiu, K. M., Peng,
Y. S., Hsu, S. P., Chen, K. L., Yang, C. Y., and Lee, O.
K. S. (2020). Prediction of the development of acute
kidney injury following cardiac surgery by machine
learning. Critical Care, 24(1).
Vos, G., Trinh, K., Sarnyai, Z., and Azghadi, M. R. (2022).
Machine Learning for Stress Monitoring from Wear-
able Devices: A Systematic Literature Review.
EAA 2024 - Special Session on Emotions and Affective Agents
506