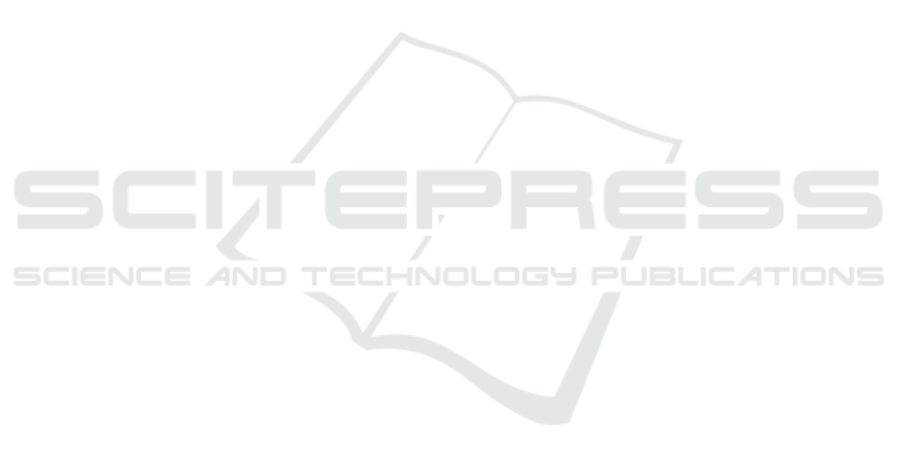
Hanhirova, J., K
¨
am
¨
ar
¨
ainen, T., Sepp
¨
al
¨
a, S., Siekkinen, M.,
Hirvisalo, V., and Yl
¨
a-J
¨
a
¨
aski, A. (2018). Latency
and throughput characterization of convolutional neu-
ral networks for mobile computer vision. In Proceed-
ings of the 9th ACM Multimedia Systems Conference,
pages 204–215.
Hou, Q., Zhou, D., and Feng, J. (2021). Coordinate atten-
tion for efficient mobile network design. In Proceed-
ings of the IEEE/CVF conference on computer vision
and pattern recognition, pages 13713–13722.
Howard, A., Sandler, M., Chu, G., Chen, L.-C., Chen, B.,
Tan, M., Wang, W., Zhu, Y., Pang, R., Vasudevan, V.,
et al. (2019). Searching for mobilenetv3. In Pro-
ceedings of the IEEE/CVF international conference
on computer vision, pages 1314–1324.
Hu, J., Shen, L., and Sun, G. (2018). Squeeze-and-
excitation networks. In Proceedings of the IEEE con-
ference on computer vision and pattern recognition,
pages 7132–7141.
Lee, M., Lee, J., Lee, D., Kim, W., Hwang, S., and Lee, S.
(2022). Robust lane detection via expanded self atten-
tion. In Proceedings of the IEEE/CVF winter confer-
ence on applications of computer vision, pages 533–
542.
Li, J., Jiang, F., Yang, J., Kong, B., Gogate, M., Dashtipour,
K., and Hussain, A. (2021). Lane-deeplab: Lane se-
mantic segmentation in automatic driving scenarios
for high-definition maps. Neurocomputing, 465:15–
25.
Li, X., Hu, X., and Yang, J. (2019). Spatial group-wise en-
hance: Improving semantic feature learning in convo-
lutional networks. arXiv preprint arXiv:1905.09646.
Lin, T.-Y., Goyal, P., Girshick, R., He, K., and Doll
´
ar, P.
(2017). Focal loss for dense object detection. In
Proceedings of the IEEE international conference on
computer vision, pages 2980–2988.
Pan, X., Shi, J., Luo, P., Wang, X., and Tang, X. (2018).
Spatial as deep: Spatial cnn for traffic scene under-
standing. In Proceedings of the AAAI Conference on
Artificial Intelligence, volume 32.
Sanchez-Iborra, R. and Skarmeta, A. F. (2020). Tinyml-
enabled frugal smart objects: Challenges and opportu-
nities. IEEE Circuits and Systems Magazine, 20(3):4–
18.
Sandler, M., Howard, A., Zhu, M., Zhmoginov, A., and
Chen, L.-C. (2018). Mobilenetv2: Inverted residu-
als and linear bottlenecks. In Proceedings of the IEEE
conference on computer vision and pattern recogni-
tion, pages 4510–4520.
Selvaraju, R. R., Cogswell, M., Das, A., Vedantam, R.,
Parikh, D., and Batra, D. (2017). Grad-cam: Visual
explanations from deep networks via gradient-based
localization. In Proceedings of the IEEE international
conference on computer vision, pages 618–626.
Wang, Q., Wu, B., Zhu, P., Li, P., Zuo, W., and Hu, Q.
(2020). Eca-net: Efficient channel attention for deep
convolutional neural networks. In Proceedings of the
IEEE/CVF conference on computer vision and pattern
recognition, pages 11534–11542.
Woo, S., Park, J., Lee, J.-Y., and Kweon, I. S. (2018). Cbam:
Convolutional block attention module. In Proceed-
ings of the European conference on computer vision
(ECCV), pages 3–19.
Xing, Y., Lv, C., Chen, L., Wang, H., Wang, H., Cao,
D., Velenis, E., and Wang, F.-Y. (2018). Advances
in vision-based lane detection: Algorithms, integra-
tion, assessment, and perspectives on acp-based par-
allel vision. IEEE/CAA Journal of Automatica Sinica,
5(3):645–661.
Xu, H., Wang, S., Cai, X., Zhang, W., Liang, X., and Li,
Z. (2020). Curvelane-nas: Unifying lane-sensitive
architecture search and adaptive point blending. In
Computer Vision–ECCV 2020: 16th European Con-
ference, Glasgow, UK, August 23–28, 2020, Proceed-
ings, Part XV 16, pages 689–704. Springer.
Yao, Z., Chen, X., et al. (2022). Efficient lane detection
technique based on lightweight attention deep neural
network. Journal of Advanced Transportation, 2022.
Yu, F., Chen, H., Wang, X., Xian, W., Chen, Y., Liu, F.,
Madhavan, V., and Darrell, T. (2020). Bdd100k: A
diverse driving dataset for heterogeneous multitask
learning. In Proceedings of the IEEE/CVF conference
on computer vision and pattern recognition, pages
2636–2645.
Zakaria, N. J., Shapiai, M. I., Ghani, R. A., Yasin, M.,
Ibrahim, M. Z., and Wahid, N. (2023). Lane detection
in autonomous vehicles: A systematic review. IEEE
Access.
Zhang, J., Zhou, Y., and Saab, R. (2023). Post-training
quantization for neural networks with provable guar-
antees. SIAM Journal on Mathematics of Data Sci-
ence, 5(2):373–399.
Zhang, L., Jiang, F., Kong, B., Yang, J., and Wang, C.
(2021). Real-time lane detection by using biologically
inspired attention mechanism to learn contextual in-
formation. Cognitive Computation, 13:1333–1344.
Zhang, Q.-L. and Yang, Y.-B. (2021). Sa-net: Shuf-
fle attention for deep convolutional neural networks.
In ICASSP 2021-2021 IEEE International Confer-
ence on Acoustics, Speech and Signal Processing
(ICASSP), pages 2235–2239. IEEE.
Zhu, X., Cheng, D., Zhang, Z., Lin, S., and Dai, J. (2019).
An empirical study of spatial attention mechanisms in
deep networks. In Proceedings of the IEEE/CVF inter-
national conference on computer vision, pages 6688–
6697.
VEHITS 2024 - 10th International Conference on Vehicle Technology and Intelligent Transport Systems
98