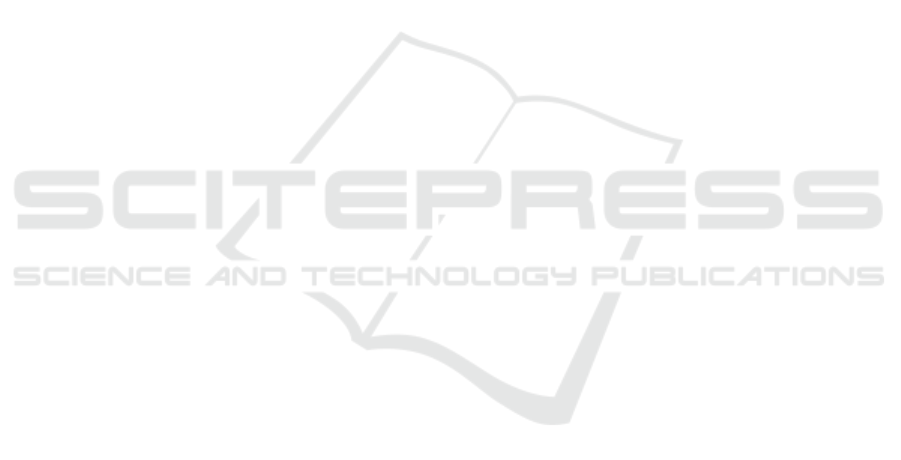
Dunbar, K. (1995). How scientists really reason: Scientific
reasoning in real-world laboratories. The nature of in-
sight, 18:365–395.
Eraut, M. (1994). Developing professional knowledge and
competence. Psychology Press.
Eraut, M. (2000). Non-formal learning and tacit knowledge
in professional work. British journal of educational
psychology, 70(1):113–136.
Guid, M., Mo
ˇ
zina, M., Bohak, C., Sadikov, A., and Bratko,
I. (2013). Building an intelligent tutoring system
for chess endgames. In International Conference
on Computer Supported Education, volume 2, pages
263–266. SCITEPRESS.
Ito, T. (2004). The thought and cognition on the verbal data
of shogi experts. Technical report, IPSJ SIG-GI-12-2.
Ito, T. (2018). Game learning support system based on fu-
ture position. ICGA Journal, 40(4):450–459.
Ito, T., Matsubara, H., and Grimbergen, R. (2005). Chunk-
ing in shogi: new findings. In Advances in Computer
Games, pages 140–154. Springer.
Ito, T. and Takano, D. (2015). Changes in cognitive pro-
cesses and brain activity. ICGA Journal, 38(4):209–
223.
Malaise, Y. and Signer, B. (2022). Personalised learning en-
vironments based on knowledge graphs and the zone
of proximal development. In CSEDU (1), pages 199–
206.
Miyake, N. (1986). Constructive interaction and the it-
erative process of understanding. Cognitive science,
10(2):151–177.
Nakatani, H. and Yamaguchi, Y. (2014). Quick concur-
rent responses to global and local cognitive informa-
tion underlie intuitive understanding in board-game
experts. Scientific Reports, 4(1):5894.
Nikkei Inc. (2022). The real of Shogi AI that gave birth
to Sota Fujii. https://bizgate.nikkei.com/article/
DGXZQOLM1405Q014042022000000 (accessed
2024-2-22).
Nishihara, Y., Takayama, R., Hishida, K., and Yamanishi,
R. (2018). Support method for beginners understand-
ing shogi games by evaluating battlefields and a king’s
danger degree by using an arrangement of komas.
Journal of Japan Society for Fuzzy Theory and Intel-
ligent Informatics, 30:796–803.
P
´
alsson, A. and Bj
¨
ornsson, Y. (2023). Unveiling con-
cepts learned by a world-class chess-playing agent. In
Proceedings of the Thirty-Second International Joint
Conference on Artificial Intelligence, pages 4864–
4872.
Plutchik, R. (1980). A general psychoevolutionary theory of
emotion. In Theories of emotion, pages 3–33. Elsevier.
Rooein, D., Paolini, P., Pernici, B., et al. (2022). Ed-
ucational chatbots: A sustainable approach for cus-
tomizable conversations for education. In CSEDU (1),
pages 314–321.
Russell, J. A. (1980). A circumplex model of affect. Journal
of personality and social psychology, 39(6):1161.
Sadiq., A. and Marentakis., G. (2023). Communicating
emotions during lectures. In Proceedings of the 15th
International Conference on Computer Supported Ed-
ucation - Volume 2: CSEDU, pages 558–565. IN-
STICC, SciTePress.
Scherer, K. R. and Wallbott, H. G. (1994). Evidence for
universality and cultural variation of differential emo-
tion response patterning. Journal of personality and
social psychology, 66(2):310.
Schut, L., Tomasev, N., McGrath, T., Hassabis, D., Pa-
quet, U., and Kim, B. (2023). Bridging the human-
ai knowledge gap: Concept discovery and transfer in
alphazero. arXiv preprint arXiv:2310.16410.
Shen, L., Wang, M., and Shen, R. (2009). Affective e-
learning: Using “emotional” data to improve learning
in pervasive learning environment. Journal of Educa-
tional Technology & Society, 12(2):176–189.
ShogiGUI (2022). http://shogigui.siganus.com/ (accessed
2024-2-22).
Siddiqui, S., Maher, M. L., Najjar, N., Mohseni, M.,
and Grace, K. (2022). Personalized curiosity engine
(pique): A curiosity inspiring cognitive system for stu-
dent directed learning. In CSEDU (1), pages 17–28.
Silver, D., Hubert, T., Schrittwieser, J., Antonoglou, I., Lai,
M., Guez, A., Lanctot, M., Sifre, L., Kumaran, D.,
Graepel, T., et al. (2018). A general reinforcement
learning algorithm that masters chess, shogi, and go
through self-play. Science, 362(6419):1140–1144.
Skinner, B. F. (1954). The science of learning and the art of
teaching. Cambridge, Mass, USA, 99:113.
Tanaka, K. (2018). Brain mechanisms of intuition in shogi
experts. Brain and Nerve= Shinkei Kenkyu no Shinpo,
70(6):607–615.
Tyng, C. M., Amin, H. U., Saad, M. N., and Malik, A. S.
(2017). The influences of emotion on learning and
memory. Frontiers in psychology, page 1454.
Vygotsky, L. et al. (2011). Interaction between learning
and development. Link
¨
opings universitet.
Wan, X., Nakatani, H., Ueno, K., Asamizuya, T., Cheng,
K., and Tanaka, K. (2011). The neural basis of intu-
itive best next-move generation in board game experts.
Science, 331(6015):341–346.
Yelle, L. E. (1979). The learning curve: Historical re-
view and comprehensive survey. Decision sciences,
10(2):302–328.
Zou, X., Ma, W., Ma, Z., and Baker, R. S. (2019). To-
wards helping teachers select optimal content for stu-
dents. In Artificial Intelligence in Education: 20th
International Conference, AIED 2019, Chicago, IL,
USA, June 25-29, 2019, Proceedings, Part II 20, pages
413–417. Springer.
CSEDU 2024 - 16th International Conference on Computer Supported Education
494