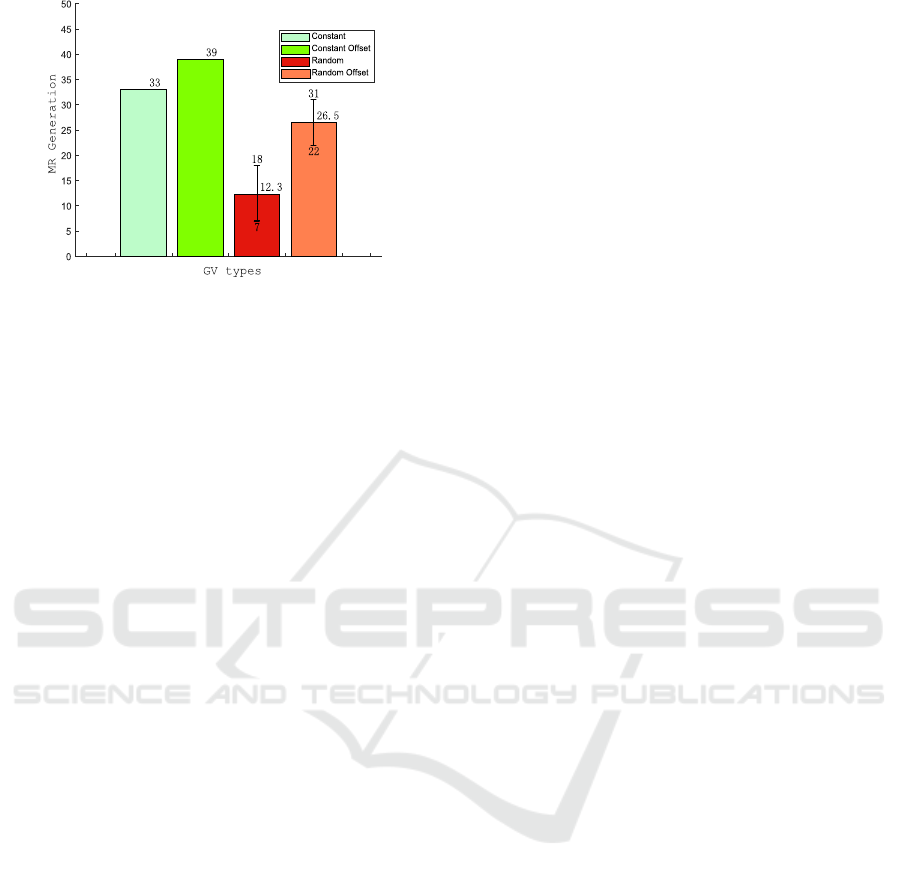
Figure 14: Comparison of detection rate of four CPM-based
GV types.
except for the Random GV, which remains at a rela-
tively low detection accuracy. Besides, as can be seen
in Fig. 14, it can be noticed that there are no error bars
for the former two types of CPM-based, namely Con-
stant and Constant Offset. On the other hand, the gaps
in MR generation numbers of each simulation remain
considerably different for the latter two types, namely
Random and Random Offset CPM-based GV types.
This is because simply the ’Random’ GV’s position
changes over time, and the probability that they stay
out of detector vehicles’ perception range becomes
larger. Furthermore, this figure also shows the ’Off-
set’ GV, either Constant Offset or Random Offset, re-
mains more detectable than their original GV versions
(Constant and Random). As the ’Offset’ GV moves
in a manner that follows one of the evaluator vehi-
cles, it will be more likely to be in the detection range.
The detection accuracy results are obtained by simu-
lation of only two detector vehicles (honest CPS vehi-
cles), and in this sense, we believe that as the number
of CPS detectors increases, the detection success rate
will grow significantly.
4 CONCLUSIVE REMARKS
As CPS is rarely considered in existing works and
there was no implementation of CPM in the popular
Veins simulator, in this work, we integrated CPS in
Veins, enabling inter-vehicle CPM communications.
Furthermore, we proposed a trust framework address-
ing two CAM-based GV attacks, namely OOA and
NCA, and four CPM-based GV attacks, namely Con-
stant, Constant Offset, Random, and Random Off-
set. A three-vehicle scenario simulation has been con-
ducted to provide a preliminary analysis of the feasi-
bility of the proposed model and show the effective-
ness in terms of assessing V2X messages’ trustwor-
thiness.
With this proposed trust model integrating the
CPS component in hand, our future work will be sim-
ulating larger-scale IoV scenarios involving more en-
tities. On the other hand, more complicated strategic
misbehavior models can also be considered in our fu-
ture work to analyze the resilience of the countermea-
sures proposed.
REFERENCES
Ambrosin, M., Yang, L. L., Liu, X., Sastry, M. R., and Al-
varez, I. J. (2019). Design of a misbehavior detection
system for objects based shared perception v2x appli-
cations. In 2019 IEEE ITSC, pages 1165–1172.
ETSI (2019). 302 637-2 v1.4.1 -intelligent transport sys-
tems; vehicular communications; basic set of applica-
tions; part 2: Specification of cooperative awareness
basic service. ETSI, Apr.
ETSI (2020). 103 415 v1. 1.1 -intelligent transport systems;
security; pre-standardization study on misbehaviour
detection; release 2. ETSI, Oct.
ETSI (2023). 103 324 v2.1.1 -intelligent transport system;
vehicular communications; basic set of applications;
collective perception service; release 2. ETSI, Jun.
Farran, H. and Khoury, D. (2023). Performance improve-
ments of vehicular pki protocol for the security of v2x
communications. In 2023 46th TSP, pages 177–182.
Gai, F., Zhang, J., Zhu, P., and Jiang, X. (2017). Trust on the
ratee: a trust management system for social internet of
vehicles. WCMC.
Gyawali, S. and Qian, Y. (2019). Misbehavior detection
using machine learning in vehicular communication
networks. In 2019-2019 IEEE ICC, pages 1–6.
Hasrouny, H., Samhat, A. E., Bassil, C., and Laouiti,
A. (2019). Trust model for secure group leader-
based communications in vanet. Wireless Networks,
25:4639–4661.
Kerrache, C. A., Lagraa, N., Calafate, C. T., Cano, J.-C.,
and Manzoni, P. (2016). T-vnets: A novel trust archi-
tecture for vehicular networks using the standardized
messaging services of etsi its. Computer Communica-
tions, 93:68–83.
Li, W. and Song, H. (2015). Art: An attack-resistant trust
management scheme for securing vehicular ad hoc
networks. IEEE transactions on ITS, 17(4):960–969.
Su, R., Sfar, A. R., Natalizio, E., Moyal, P., and Song, Y.-Q.
(2022). Ensuring trustworthiness in ioit/aiot: A phase-
based approach. IEEE IoT Magazine, 5(2):84–88.
Tsukada, M., Arii, S., Ochiai, H., and Esaki, H. (2022).
Misbehavior detection using collective perception un-
der privacy considerations. In 2022 19th CCNC, pages
808–814. IEEE.
Van Der Heijden, R. W., Lukaseder, T., and Kargl, F. (2018).
Veremi: A dataset for comparable evaluation of mis-
behavior detection in vanets. In 14th SecureComm
2018, Proceedings, Part I, pages 318–337. Springer.
Veins. Veins. https://veins.car2x.org/. Accessed:
12.24.2023.
Assessing Trustworthiness of V2X Messages: A Cooperative Trust Model Against CAM- and CPM-Based Ghost Vehicles in IoV
283