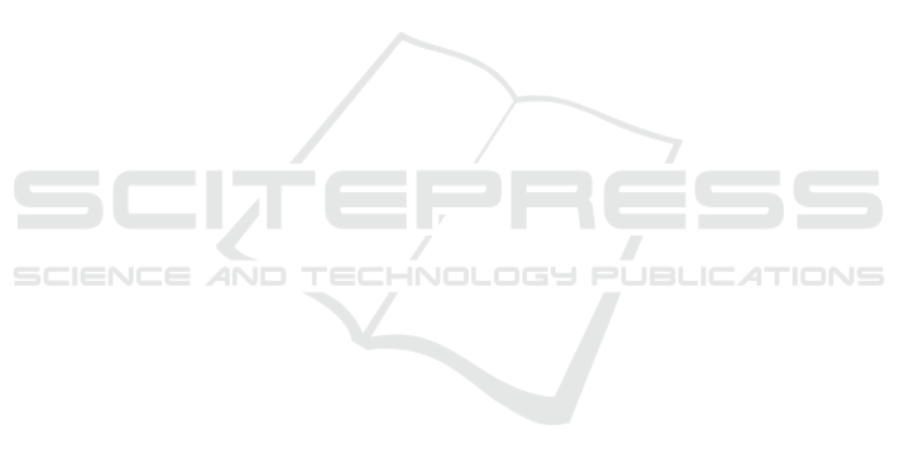
Eighteenth Annual ACM-SIAM Symposium on Dis-
crete Algorithms, SODA ’07, page 1027–1035, USA.
Society for Industrial and Applied Mathematics.
Dyoub, A., Costantini, S., and Letteri, I. (2022). Care robots
learning rules of ethical behavior under the supervi-
sion of an ethical teacher (short paper). In Joint Pro-
ceedings of the 1st International Workshop on HYbrid
Models for Coupling Deductive and Inductive ReA-
soning (HYDRA 2022) and the 29th RCRA Workshop
on Experimental Evaluation of Algorithms for Solv-
ing Problems with Combinatorial Explosion (RCRA
2022) co-located with the 16th International Confer-
ence on Logic Programming and Non-monotonic Rea-
soning (LPNMR 2022), Genova Nervi, Italy, Septem-
ber 5, 2022, volume 3281 of CEUR Workshop Pro-
ceedings, pages 1–8. CEUR-WS.org.
Dyoub, A., Costantini, S., Letteri, I., and Lisi, F. A.
(2021). A logic-based multi-agent system for ethi-
cal monitoring and evaluation of dialogues. In Pro-
ceedings 37th International Conference on Logic Pro-
gramming (Technical Communications), ICLP Tech-
nical Communications 2021, Porto (virtual event), 20-
27th September 2021, volume 345 of EPTCS, pages
182–188.
Dyoub, A., Costantini, S., Lisi, F. A., and Letteri, I. (2020).
Logic-based machine learning for transparent ethical
agents. In Proceedings of the 35th Italian Conference
on Computational Logic - CILC 2020, Rende, Italy,
October 13-15, 2020, volume 2710 of CEUR Work-
shop Proceedings, pages 169–183. CEUR-WS.org.
Engle, R. F. and Granger, C. W. J. (1987). Co-integration
and error correction: Representation, estimation, and
testing. Econometrica, 55(2):251–276.
Gasperis, G. D., Costantini, S., Rafanelli, A., Migliarini,
P., Letteri, I., and Dyoub, A. (2023). Exten-
sion of constraint-procedural logic-generated environ-
ments for deep q-learning agent training and bench-
marking. J. Log. Comput., 33(8):1712–1733.
Kirchg
¨
assner, G. and Wolters, J. (2007). Granger Causal-
ity, pages 93–123. Springer Berlin Heidelberg, Berlin,
Heidelberg.
Letteri, I. (2023a). AITA: A new framework for trading
forward testing with an artificial intelligence engine.
In Proceedings of the Italia Intelligenza Artificiale -
Thematic Workshops co-located with the 3rd CINI Na-
tional Lab AIIS Conference on Artificial Intelligence
(Ital IA 2023), Pisa, Italy, May 29-30, 2023, volume
3486 of CEUR Workshop Proceedings, pages 506–
511. CEUR-WS.org.
Letteri, I. (2023b). Volts: A volatility-based trading system
to forecast stock markets trend using statistics and ma-
chine learning.
Letteri, I., Cecco, A. D., Dyoub, A., and Penna, G. D.
(2020a). A novel resampling technique for imbal-
anced dataset optimization. CoRR, abs/2012.15231.
Letteri, I., Cecco, A. D., Dyoub, A., and Penna, G. D.
(2021a). Imbalanced dataset optimization with new
resampling techniques. In Arai, K., editor, Intelligent
Systems and Applications - Proceedings of the 2021
Intelligent Systems Conference, IntelliSys 2021, Am-
sterdam, The Netherlands, 2-3 September, 2021, Vol-
ume 2, volume 295 of Lecture Notes in Networks and
Systems, pages 199–215. Springer.
Letteri, I., Cecco, A. D., and Penna, G. D. (2020b). Dataset
optimization strategies for malwaretraffic detection.
CoRR, abs/2009.11347.
Letteri, I., Cecco, A. D., and Penna, G. D. (2021b). New op-
timization approaches in malware traffic analysis. In
Machine Learning, Optimization, and Data Science -
7th International Conference, LOD 2021, Grasmere,
UK, October 4-8, 2021, Revised Selected Papers, Part
I, volume 13163 of Lecture Notes in Computer Sci-
ence, pages 57–68. Springer.
Letteri, I., Penna, G. D., and Caianiello, P. (2019a). Fea-
ture selection strategies for HTTP botnet traffic de-
tection. In 2019 IEEE European Symposium on Se-
curity and Privacy Workshops, EuroS&P Workshops
2019, Stockholm, Sweden, June 17-19, 2019, pages
202–210. IEEE.
Letteri, I., Penna, G. D., and Gasperis, G. D. (2019b).
Security in the internet of things: botnet detec-
tion in software-defined networks by deep learning
techniques. Int. J. High Perform. Comput. Netw.,
15(3/4):170–182.
Letteri, I., Penna, G. D., Gasperis, G. D., and Dyoub, A.
(2022). Dnn-forwardtesting: A new trading strat-
egy validation using statistical timeseries analysis and
deep neural networks.
Letteri, I., Penna, G. D., Gasperis, G. D., and Dyoub,
A. (2023). Trading strategy validation using for-
wardtesting with deep neural networks. In Arami,
M., Baudier, P., and Chang, V., editors, Proceedings
of the 5th International Conference on Finance, Eco-
nomics, Management and IT Business, FEMIB 2023,
Prague, Czech Republic, April 23-24, 2023, pages 15–
25. SCITEPRESS.
Niennattrakul, V. and Ratanamahatana, C. A. (2007). On
clustering multimedia time series data using k-means
and dynamic time warping. In 2007 International
Conference on Multimedia and Ubiquitous Engineer-
ing (MUE’07), pages 733–738.
Wahid, A. and Chandra Sekhara Rao, A. (2020). An out-
lier detection algorithm based on knn-kernel density
estimation. In 2020 International Joint Conference on
Neural Networks (IJCNN), pages 1–8.
Yang, D. and Zhang, Q. (2000). Drift-independent volatility
estimation based on high, low, open, and close prices.
The Journal of Business, 73(3):477–492.
Stock Market Forecasting Using Machine Learning Models Through Volatility-Driven Trading Strategies
103