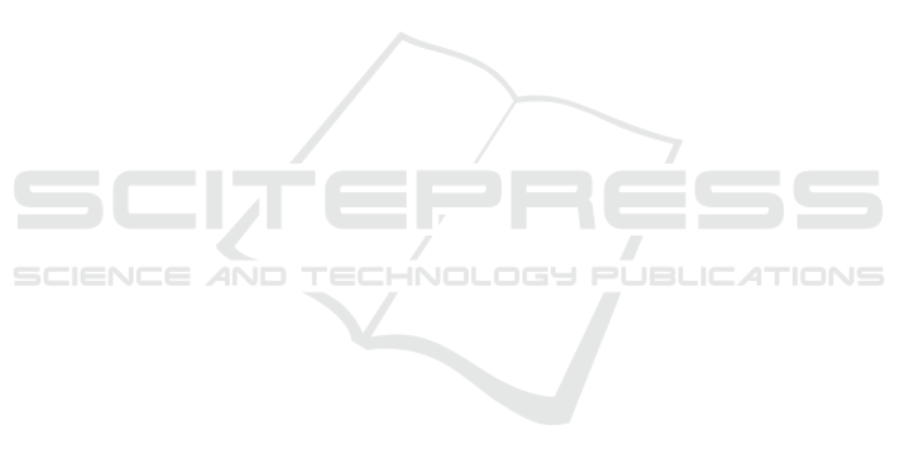
Curation System for Museums. TECHART: Journal
of Arts and Imaging Science, 9(3):6–10.
Kulesz, O. (2020). Supporting culture in the dig-
ital age. Surry Hills, Australia: International
federation of Arts Councils and Culture Agencies.
https://ifacca. org/en/news/202 0/04/23/supporting-
culture-digital-age/accessed April, 25:2020.
Laato, S., Vilppu, H., Heimonen, J., Hakkala, A., Bj
¨
orne, J.,
Farooq, A., Salakoski, T., and Airola, A. (2020). Prop-
agating AI Knowledge Across University Disciplines-
The Design of A Multidisciplinary AI Study Mod-
ule. In 2020 IEEE Frontiers in Education Conference
(FIE), pages 1–9. IEEE.
Laupichler, M. C., Aster, A., Haverkamp, N., and Raupach,
T. (2023). Development of the “Scale for the assess-
ment of non-experts’ AI literacy”–An exploratory fac-
tor analysis. Computers in Human Behavior Reports,
12:100338.
Long, D. and Magerko, B. (2020). What is AI literacy?
Competencies and design considerations. In Proceed-
ings of the 2020 CHI conference on human factors in
computing systems, pages 1–16.
Lowagie, H. (2023). From Bias to Transparency: Ethical
Imperatives in AI-Based Library Cataloging.
Macr
`
ı, E. and Cristofaro, C. L. (2021). The digitalisation
of cultural heritage for sustainable development: The
impact of Europeana. Cultural Initiatives for Sustain-
able Development: Management, Participation and
Entrepreneurship in the Cultural and Creative Sector,
pages 373–400.
Marchello, G., Giovanelli, R., Fontana, E., Cannella,
F., and Traviglia, A. (2023). CULTURAL HER-
ITAGE DIGITAL PRESERVATION THROUGH AI-
DRIVEN ROBOTICS. The International Archives of
the Photogrammetry, Remote Sensing and Spatial In-
formation Sciences, 48:995–1000.
Mehrabi, N., Morstatter, F., Saxena, N., Lerman, K., and
Galstyan, A. (2021). A survey on bias and fairness in
machine learning. ACM computing surveys (CSUR),
54(6):1–35.
Moral-Andr
´
es, F., Merino-G
´
omez, E., Reviriego, P., and
Lombardi, F. (2023). Can artificial intelligence re-
construct ancient mosaics? Studies in Conservation,
pages 1–14.
Ng, D. T. K., Leung, J. K. L., Chu, S. K. W., and Qiao, M. S.
(2021). Conceptualizing AI literacy: An exploratory
review. Computers and Education: Artificial Intelli-
gence, 2:100041.
Ntoutsi, E., Fafalios, P., Gadiraju, U., Iosifidis, V., Ne-
jdl, W., Vidal, M.-E., Ruggieri, S., Turini, F., Pa-
padopoulos, S., Krasanakis, E., et al. (2020). Bias in
data-driven artificial intelligence systems—An intro-
ductory survey. Wiley Interdisciplinary Reviews: Data
Mining and Knowledge Discovery, 10(3):e1356.
Oksanen, A., Cvetkovic, A., Akin, N., Latikka, R.,
Bergdahl, J., Chen, Y., and Savela, N. (2023). Arti-
ficial intelligence in fine arts: A systematic review of
empirical research. Computers in Human Behavior:
Artificial Humans, page 100004.
Pansoni, S., Tiribelli, S., Paolanti, M., Di Stefano, F.,
Malinverni, E., Frontoni, E., Giovanola, B., et al.
(2023). Artificial Intelligence and Cultural Heritage:
Design and Assessment of an Ethical Framework. In
29th CIPA Symposium “Documenting, Understand-
ing, Preserving Cultural Heritage. Humanities and
Digital Technologies for Shaping the Future”, vol-
ume 48, pages 1149–1155. The International Archives
of the Photogrammetry, Remote Sensing and Spa-
tial . . . .
Pavlidis, G. (2022). AI trends in digital humanities research.
Trends Comput Sci Inf Technol, 7(2):026–034.
Piotrowski, M. (2012). Natural language processing for
historical texts. Morgan & Claypool Publishers.
Raina, V., Krishnamurthy, S., Raina, V., and Krishna-
murthy, S. (2022). Natural language processing.
Building an Effective Data Science Practice: A
Framework to Bootstrap and Manage a Successful
Data Science Practice, pages 63–73.
Rani, S., Jining, D., Shah, D., Xaba, S., and Singh, P. R.
(2023). Exploring the Potential of Artificial Intelli-
gence and Computing Technologies in Art Museums.
In ITM Web of Conferences, volume 53. EDP Sci-
ences.
Sang
¨
uesa, R. and Guersenzvaig, A. (2019). AI as a Design
Material: Dealing with new agencies. Temes de Dis-
seny, (35):6–25.
Schumann, C.-A., Reuther, K., Tittmann, C., Clauß, A.-M.,
and Kauper, J. (2020). Impact of ai application on
digital education focused on ste (a) m. In EDEN Con-
ference Proceedings, number 1, pages 153–161.
Sporleder, C. (2010). Natural language processing for cul-
tural heritage domains. Language and Linguistics
Compass, 4(9):750–768.
Terras, M., Coleman, S., Drost, S., Elsden, C., Helgason,
I., Lechelt, S., Osborne, N., Panneels, I., Pegado, B.,
Schafer, B., et al. (2021). The value of mass-digitised
cultural heritage content in creative contexts. Big Data
& Society, 8(1):20539517211006165.
Tholander, J. and Jonsson, M. (2023). Design ideation
with ai-sketching, thinking and talking with Genera-
tive Machine Learning Models. In Proceedings of the
2023 ACM Designing Interactive Systems Conference,
pages 1930–1940.
Van Wynsberghe, A. (2021). Sustainable AI: AI for sus-
tainability and the sustainability of AI. AI and Ethics,
1(3):213–218.
Verheijden, M. P. and Funk, M. (2023). Collaborative Dif-
fusion: Boosting Designerly Co-Creation with Gen-
erative AI. In Extended Abstracts of the 2023 CHI
Conference on Human Factors in Computing Systems,
pages 1–8.
Wang, B., Rau, P.-L. P., and Yuan, T. (2023). Measur-
ing user competence in using artificial intelligence:
validity and reliability of artificial intelligence lit-
eracy scale. Behaviour & information technology,
42(9):1324–1337.
Wang, Q. and Li, L. (2022). Museum Relic Image Detection
and Recognition Based on Deep Learning. Computa-
tional Intelligence and Neuroscience, 2022:1–11.
AI Literacy for Cultural and Design Studies
49