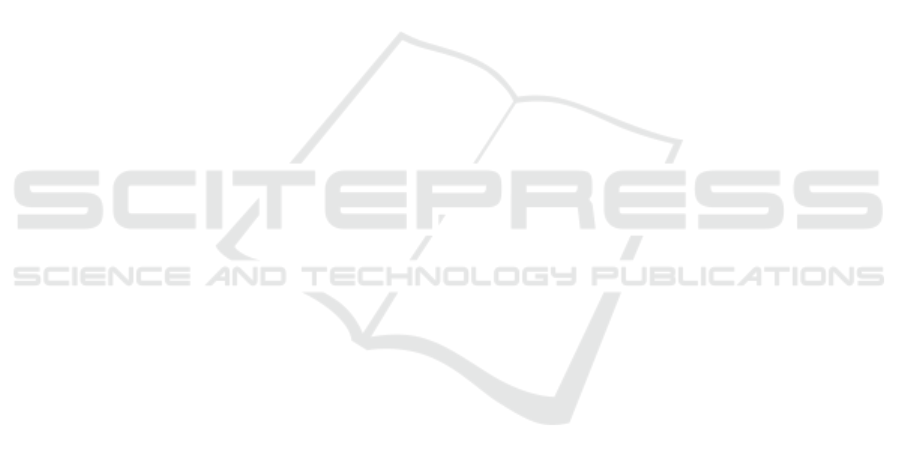
closely aligned with their learning interests and re-
quirements, thereby increasing their engagement and
comprehension. In summary, this research not only
advances the field of STC but also offers a practical
solution to enhance video-based learning.
Future work can consider increasing the size of
text which includes: increasing the number of video
segments and the duration of each segment to include
more transcript text lines. This to allow us observe
how it affects the performance of various models
within the given short text size constraints. Once the
video segments are characterised, we have developed
a framework to link video segments to support learn-
ing of specific domain concepts(Mohammed, 2024).
We envisage applications of the work in other do-
mains related to using videos for life-wide learning
based on other people’s experiences, e.g. communica-
tion, project management, empathy, where automated
video characterization can enable efficient video link-
ing to support informal learning.
REFERENCES
Barrett, M. D. (2018). Reference framework of competences
for democratic culture. Council of Europe.
Bywater, J. P., Floryan, M., and Chiu, J. L. (2021). Discs:
A new sequence segmentation method for open-ended
learning environments. Springer, pages 88–100.
Commission of the European Communities (2000). A mem-
orandum on lifelong learning. European Commission.
Denny, P., Sarsa, S., Hellas, A., and Leinonen, J. (2022).
Robosourcing educational resources – leveraging
large language models for learnersourcing. arXiv.
Dodson, S., Roll, I., Harandi, N. M., Fels, S., and Yoon,
D. (2019). Weaving together media, technologies and
people. Inf. and Learning Sciences, 120:519–540.
Gallagher, R. J., Reing, K., Kale, D. C., and Steeg, G. V.
(2017). Anchored correlation explanation: Topic
modeling with minimal domain knowledge. Trans-
actions of the ACL, 5:529–542.
Grootendorst, M. (2022). Bertopic: Neural topic modeling
with a class-based tf-idf procedure. arXiv.
Jagarlamudi, J., Daum
´
e, H., and Udupa, R. (2012). Incor-
porating lexical priors into topic models. Conference
of the European Chapter of the Association for Com-
putational Linguistics, pages 204–213.
Lee, J. Y. and Dernoncourt, F. (2016). Sequential short-text
classification with recurrent and convolutional neural
networks. In Knight, K., Nenkova, A., and Rambow,
O., editors, Proceedings of the 2016 Conference of the
North American Chapter of the Association for Com-
putational Linguistics: Human Language Technolo-
gies, pages 515–520, San Diego, California. Associa-
tion for Computational Linguistics.
Li, C., Duan, Y., Wang, H., Zhang, Z., Sun, A., and Ma,
Z. (2017). Enhancing topic modeling for short texts
with auxiliary word embeddings. ACM Transactions
on Information Systems, 36:1–30.
Luca, C., Canale, L., and Farinetti, L. (2019). Visa: A
supervised approach to indexing video lectures with
semantic annotations. 2019 IEEE 43rd Annual Com-
puter Software and Applications Conference.
Meng, Y., Shen, J., Zhang, C., and Han, J. (2019). Weakly-
supervised hierarchical text classification. Proceed-
ings of the 33rd AAAI Conference, 33:6826–6833.
Mitrovic, A., Dimitrova, V., and Weerasinghe, A. (2016).
Reflective experiential learning: Using active video
watching for soft skills training. Proceedings of the
24th Int. Conference on Computers in Education.
Mohammed, A. (2022). Generating narratives of video seg-
ments to support learning. In 23rd International Con-
ference on Artificial Intelligence in Education, pages
22–28. Springer.
Mohammed, A. (2024). Generating Video Narratives to
Support Learning. PhD thesis, University of Leeds.
OpenAI (2023). Chat completions api. https://platform.
openai.com/docs/guides/text-generation/chat-
completions-api [Accessed: (June 2023)].
Qiang, J., Qian, Z., Li, Y., Yuan, Y., and Wu, X. (2020).
Short text topic modeling techniques, applications,
and performance: A survey. IEEE Transactions on
Knowledge and Data Engineering, pages 1–1.
Redeker, C., Leis, M., Leendertse, M., Punie, Y., Gijsbers,
G., Kirschner, P. A., Stoyanov, S., and Hoogveld, B.
(2012). The future of learning: Preparing for change.
Sabli
´
c, M., Mirosavljevi
´
c, A., and
ˇ
Skugor, A. (2020).
Video-based learning (vbl)—past, present and future:
an overview of the research published from 2008 to
2019. Technology, Knowledge and Learning.
Savelka, J., Agarwal, A., Bogart, C., and Sakr, M.
(2023). Large language models (gpt) struggle to an-
swer multiple-choice questions about code. arXiv.
Schlotterbeck, D., Uribe, P., Jim
´
enez, A., Araya, R., , v.,
and Caballero, D. (2021). Tarta: Teacher activity rec-
ognizer from transcriptions and audio. Lecture Notes
in Computer Science, pages 369–380.
Shu, K., Mukherjee, S., Zheng, G., Awadallah, A. H., Shok-
ouhi, M., and Dumais, S. T. (2020). Learning with
weak supervision for email intent detection. arXiv.
Song, G., Ye, Y., Du, X., Huang, X., and Bie, S. (2014).
Short text classification: A survey. Journal of Multi-
media, 9.
Tseng, S.-S. (2021). The influence of teacher annotations
on student learning engagement and video watching
behaviors. International Journal of Educational Tech-
nology in Higher Education, 18.
T
¨
urker, R., Zhang, L., Alam, M., and Sack, H. (2020).
Weakly supervised short text categorization using
world knowledge. Springer eBooks, pages 584–600.
Xie, S. M., Raghunathan, A., Liang, P., and Ma, T.
(2021). An explanation of in-context learning as im-
plicit bayesian inference. arXiv.
Zhou, Z.-H. (2017). A brief introduction to weakly super-
vised learning. National Science Review, 5:44–53.
CSEDU 2024 - 16th International Conference on Computer Supported Education
204