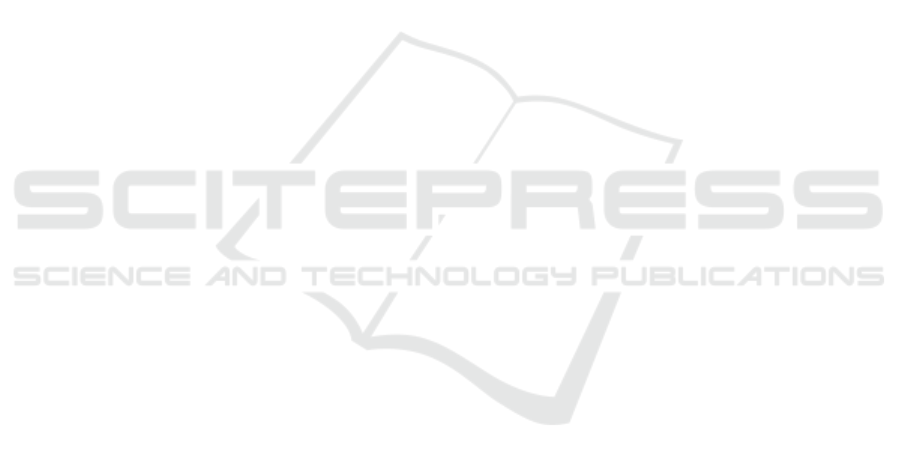
Choi, J., Parker, S. M., Knarr, B. A., Gwon, Y., and Youn, J.-
H. (2021). Wearable Sensor-Based Prediction Model
of Timed up and Go Test in Older Adults. Sen-
sors, 21(20):6831. Number: 20 Publisher: Multidis-
ciplinary Digital Publishing Institute.
Diao, Y., Lou, N., Liang, S., Zhang, Y., Ning, Y., Li, G.,
and Zhao, G. (2021). A Novel Environment-Adaptive
Timed Up and Go Test System for Fall Risk Assess-
ment With Wearable Inertial Sensors. IEEE Sensors
Journal, 21(16):18287–18297.
Dierick, F., Stoffel, P., Sch
¨
utz, G., and Buisseret, F.
(2022). High Specificity of Single Inertial Sensor-
Supplemented Timed Up and Go Test for Assessing
Fall Risk in Elderly Nursing Home Residents. Sen-
sors, 22(6).
Frenken, T., Brell, M., G
¨
overcin, M., Wegel, S., and Hein,
A. (2013). aTUG: technical apparatus for gait and bal-
ance analysis within component-based Timed Up &
Go using mutual ambient sensors. Journal of Ambient
Intelligence and Humanized Computing, 4(6):759–
778.
Fudickar, S., Hellmers, S., Lau, S., Diekmann, R., Bauer,
J., and Hein, A. (2020). Measurement System for
Unsupervised Standardized Assessment of Timed ”Up
& Go” and Five Times Sit to Stand Test in the
Community-A Validity Study. Sensors, 20(10).
Gambi, E., Ciattaglia, G., Santis, A. D., and Senigagliesi, L.
(2020). Millimeter wave radar data of people walking.
Data in Brief, 31(105996).
Greene, B., Doheny, E., O’Halloran, A., and Kenny, R.
(2014). Frailty status can be accurately assessed using
inertial sensors and the TUG test. Age and Ageing,
43(3):406–411.
Kataoka, Y., Saito, Y., Takeda, R., Ishida, T., Tadano, S.,
Suzuki, T., Nakamura, K., Nakata, A., Osuka, S., Ya-
mada, S., Samukawa, M., and Tohyama, H. (2023).
Evaluation of Lower-Limb Kinematics during Timed
Up and Go (TUG) Test in Subjects with Locomotive
Syndrome (LS) Using Wearable Gait Sensors (H-Gait
System). Sensors, 23(2).
Laved
´
an, A., Viladrosa, M., J
¨
urschik, P., Botigu
´
e, T., Nu
´
ın,
C., Masot, O., and Laved
´
an, R. (2018). Fear of falling
in community-dwelling older adults: A cause of falls,
a consequence, or both? PLoS one, 13(3):e0194967.
Li, T., Chen, J., Hu, C., Ma, Y., Wu, Z., Wan, W., Huang,
Y., Jia, F., Gong, C., Wan, S., and Li, L. (2018).
Automatic Timed Up-and-Go Sub-Task Segmenta-
tion for Parkinson’s Disease Patients Using Video-
Based Activity Classification. IEEE Transactions
on Neural Systems and Rehabilitation Engineering,
26(11):2189–2199.
Moncada, L. V. V. (2011). Management of Falls in Older
Persons: A Prescription for Prevention. American
Family Physician, 84(11):1267–1276.
NICE (2013). National Institute for Health and Care Ex-
cellence. Falls in older people: assessing risk and pre-
vention. Clinical guideline [CG161]. Technical report,
NICE.
NodeNs Medical Ltd. (2023). Millimetre Wave Radar.
https://nodens.eu.
Rajab, K. Z., Wu, B., Alizadeh, P., and Alomainy, A.
(2021). Multi-target tracking and activity classifica-
tion with millimeter-wave radar. Applied Physics Let-
ters, 119(3):034101.
Richards, M. A. (2014). Fundamentals of Radar Signal
Processing. McGraw-Hill Education, New York, 2nd
edition edition.
Savoie, P., Cameron, J. A. D., Kaye, M. E., and
Scheme, E. J. (2020). Automation of the Timed-
Up-and-Go Test Using a Conventional Video Camera.
IEEE Journal of Biomedical and Health Informatics,
24(4):1196–1205. Conference Name: IEEE Journal
of Biomedical and Health Informatics.
Soubra, R., Mourad-Chehade, F., and Chkeir, A. (2023).
Automation of the Timed Up and Go Test Using a
Doppler Radar System for Gait and Balance Analysis
in Elderly People. Journal of Healthcare Engineering,
2023:e2016262. Publisher: Hindawi.
Spina, S., Facciorusso, S., D’ascanio, M. C., Morone, G.,
Baricich, A., Fiore, P., and Sandamato, A. (2022).
Sensor based assessment of turning during instru-
mented Timed Up and Go Test for quantifying mo-
bility in chronic stroke patients. European Journal of
Physical and Rehabilitation Medicine, 59(1):6–13.
Sun, R. and Sosnoff, J. J. (2018). Novel sensing technology
in fall risk assessment in older adults: a systematic
review. BMC Geriatrics, 18(1):14.
Takayanagi, N., Sudo, M., Yamashiro, Y., Lee, S.,
Kobayashi, Y., Niki, Y., and Shimada, H. (2019).
Relationship between Daily and In-laboratory Gait
Speed among Healthy Community-dwelling Older
Adults. Scientific Reports, 9(1):3496. Number: 1 Pub-
lisher: Nature Publishing Group.
Taylor, K. I., Staunton, H., Lipsmeier, F., Nobbs, D., and
Lindemann, M. (2020). Outcome measures based
on digital health technology sensor data: data- and
patient-centric approaches. npj Digital Medicine,
3(1):1–8. Number: 1 Publisher: Nature Publishing
Group.
WHO (2021). World Health Organization fact sheet on
falls providing key facts and information on scope
of the problem, who is at risk, prevention, who
response. Falls, https://www.who.int/news-room/fact-
sheets/detail/falls.
Yu, Z., Taha, A., Taylor, W., Zahid, A., Rajab, K., Hei-
dari, H., Imran, M. A., and Abbasi, Q. H. (2022).
A Radar-Based Human Activity Recognition Using a
Novel 3-D Point Cloud Classifier. IEEE Sensors Jour-
nal, 22(19):18218–18227.
ICT4AWE 2024 - 10th International Conference on Information and Communication Technologies for Ageing Well and e-Health
168