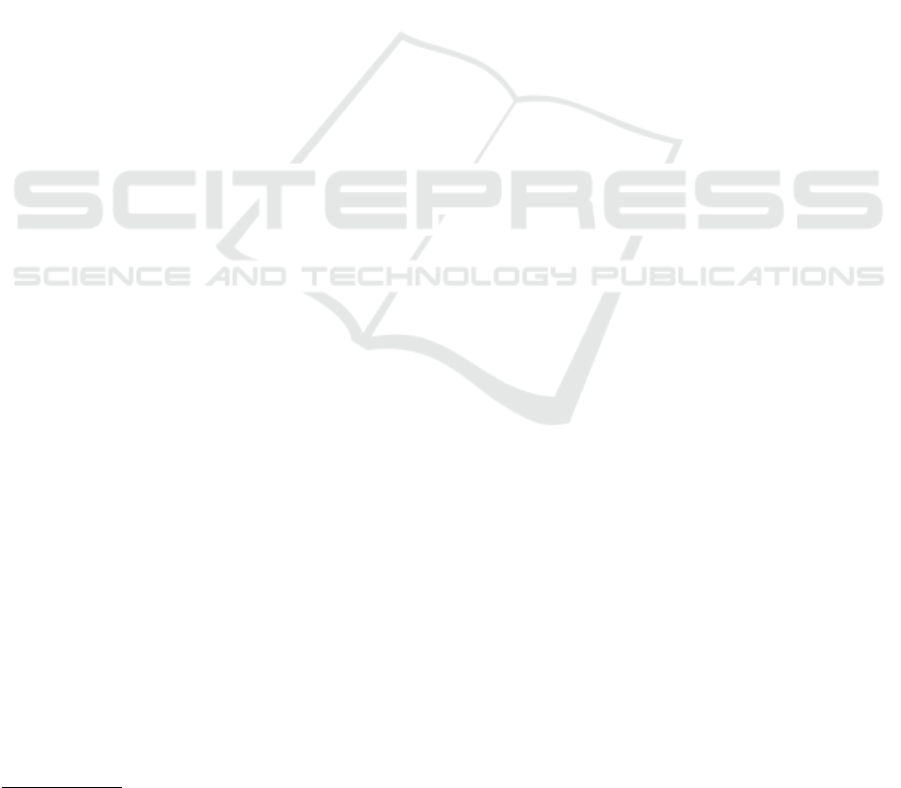
It is important to not lift these results out of pro-
portion, particularly because we used simplified and
over-realistic models for communication and percep-
tion. Nonetheless, the potential upsides of enrich-
ing FCD with data from perception sensors have been
proven and should further be examined.
5 CONCLUSION & OUTLOOK
In this paper, we investigated the research question
of whether a good level of Traffic State Estimation
(TSE) based on Floating Car Data (FCD) can be real-
ized with sufficient precision, even at low equipment
rates, yet with high data quality from perception sen-
sors.
In previous work(Schweppenh
¨
auser et al., 2023),
we offered an introduction to established speed met-
rics for TSE and respective sensor modalities as well
as their upsides and shortcomings. The main aspects
of this work have been reiterated in the first part of
this paper. Floating Car Data approaches have been
identified to be the most common source for current-
day navigation applications. The Temporal Mean
Speed(Yoon et al., 2007) was found to be one of the
core measures to be estimated from FCD traces us-
ing curve-fitted traversals. In contrast to conventional
methods, we inspected how perception sensors could
be used to improve the data quality for the sake of
mean speed estimation. We differentiated three traffic
scenarios, Flowing, Oncoming, and Intersecting Traf-
fic that show different levels of potential gain. Based
on this, we utilized Eclipse MOSAIC’s(Schrab et al.,
2023) perception module and showed how data gath-
ered from surrounding vehicles can be used as addi-
tional input for mean speed estimation in the form of
the Sample-Based Mean Speed.
To evaluate the established measures, we set up
a simulation-based test, leveraging the strengths of
MOSAIC. Building on top of previously published
applications
1
, we developed a new processor for cal-
culating the sample-based mean speed. By coupling
state-of-the-art simulators and utilizing the calibrated
large-scale BeST scenario(Schrab et al., 2022), we
can generate sensible and transferable results.
Focusing on the area of Charlottenburg, we
first conducted a study comparing the temporal and
sample-based mean speed with the ground truth gen-
erated by the traffic simulator Eclipse SUMO. We
furthermore identified that estimation quality strongly
depends on the road type for both the conventional
1
https://github.com/mosaic-addons/traffic-state-
estimation
and the perception-enriched FCD as well as the mar-
ket penetration of connected vehicles. Consequently,
we looked at time-series plots for the speed estima-
tions and the amount of data received for three ex-
emplary road segments in dependence on penetration
rates. We were able to validate the proposed ap-
proach of the Sample-Based Mean Speed as a sensible
method to estimate the mean speed. This analysis fur-
ther showed that regardless of the targeted road type
the perception increases the amount of received data,
and thereby improves estimation quality. As a conse-
quence, this indicates that penetration rates of 1 % and
lower can be sufficient to provide reliable mean speed
estimates for larger road segments. Nonetheless, we
also identified shortcomings and less promising re-
sults for smaller road segments, which we intend to
further investigate in future work.
Evaluating the estimation quality on a global scale
is a non-trivial task. Therefore, we defined the result-
ing travel time estimations as a proxy for the overall
TSE quality. Our results indicated that using percep-
tion data from surrounding vehicles improves average
travel time estimates over conventional FCD, partic-
ularly at lower penetration rates, thereby indicating a
globally improved estimation quality.
Concerning a real-world deployment of the pro-
posed system, some challenges arise. For one, we
heavily simplified the vehicle perception and sen-
sor fusion. Real sensors suffer from occlusion and
noise, which reduce sample size and quality. Sec-
ondly, we disregarded the constraints of the cellular
network. Cell coverage, timing issues, losses, and
package sizes are not realistically modeled. Nonethe-
less, this study still holds meaning as the listed limita-
tions can either be overcome by improved technology
or only have a minor impact on final results.
In conclusion, our results indicate that data from
vehicular perception sensors can be a valuable addi-
tion to the input of Intelligent Transportation Systems
such as TSE and should be further researched. In the
future, we aim to make our results more quantifiable
by improving the realism of the applied perception
model, including occlusion and noise. We further aim
to investigate how the deployed estimation techniques
react to more disruptive traffic patterns like conges-
tion, construction sides, and second-row parking.
ACKNOWLEDGEMENTS
This work was supported by the KIS’M project
through the German Federal Ministry for Economic
Affairs and Climate Action under grant 45AVF3001E.
VEHITS 2024 - 10th International Conference on Vehicle Technology and Intelligent Transport Systems
110