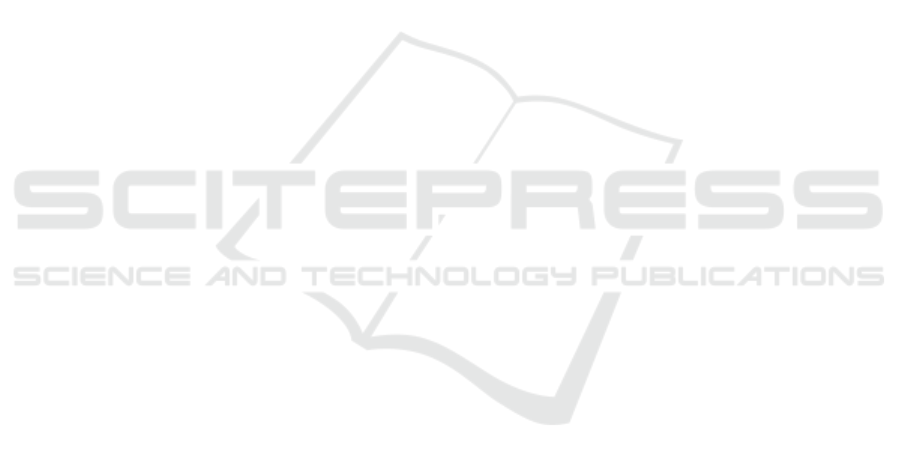
two variants in future work: the list variant and the
set variant. A/B testing could be performed to see if
one system is preferred or is more successful. Fu-
ture user surveys have the potential to delve into spe-
cific subpopulations that exhibited noteworthy results
with statistical significance. Additionally, the contra-
dictory results found comparing the indirect rating of
the recommendation quality of list and set and the se-
lected preferred variant should be investigated further.
ACKNOWLEDGEMENTS
During the preparation of this work, the au-
thors used https://www.deepl.com and https://www.
writefull.com in all sections to achieve better trans-
lations and more fluent texts. After using these ser-
vices, the authors reviewed and edited the content as
needed and take full responsibility for the content of
the publication.
REFERENCES
Berthold, C., Leichsenring, H., Brandenburg, U., G
¨
uttner,
A., Kreft, A.-K., Morzick, B., Noe, S., Reumsch
¨
ussel,
E., Schmalreck, U., and Willert, M. (2012). CHE Di-
versity Report B1: Studierende mit Migrationshinter-
grund [CHE Diversity Report B1: Students with a mi-
gration background]. Technical report.
Bollen, D., Knijnenburg, B. P., Willemsen, M. C., and
Graus, M. (2010). Understanding choice overload in
recommender systems. In Proceedings of the fourth
ACM conference on Recommender systems, RecSys
’10, pages 63–70, New York, NY, USA. Association
for Computing Machinery.
Elbadrawy, A. and Karypis, G. (2016). Domain-Aware
Grade Prediction and Top-n Course Recommendation.
In Proceedings of the 10th ACM Conference on Rec-
ommender Systems, RecSys ’16, pages 183–190, New
York, NY, USA. Association for Computing Machin-
ery.
Hernandez-Bocanegra, D. C. and Ziegler, J. (2023). Ex-
plaining Recommendations through Conversations:
Dialog Model and the Effects of Interface Type and
Degree of Interactivity. ACM Transactions on Inter-
active Intelligent Systems, 13(2):1–47.
Khan, M. A. Z. and Polyzou, A. (2023). Session-Based
Course Recommendation Frameworks Using Deep
Learning. In Proceedings of the 16th International
Conference on Educational Data Mining (EDM),
Bengaluru, India. International Educational Data Min-
ing Society.
Ma, B., Taniguchi, Y., and Konomi, S. (2020). Course Rec-
ommendation for University Environments. In Pro-
ceedings of the 13th International Conference on Edu-
cational Data Mining (EDM), pages 460–466, Online.
International Educational Data Mining Society.
Matayoshi, J. and Karumbaiah, S. (2021). Investigating the
Validity of Methods Used to Adjust for Multiple Com-
parisons in Educational Data Mining. In Proceedings
of the 14th International Conference on Educational
Data Mining, pages 33–45, Online. International Ed-
ucational Data Mining Society.
Miethe, I., Boysen, W., Grabowsky, S., and Kludt, R.
(2014). First Generation Students an deutschen
Hochschulen: Selbstorganisation und Studiensitua-
tion am Beispiel der Initiative www.ArbeiterKind.de
[First Generation Students at German universities:
Self-organization and study situation using the exam-
ple of the initiative www.ArbeiterKind.de]. edition
sigma.
Morsy, S. and Karypis, G. (2019). Will This Course In-
crease or Decrease Your GPA? Towards Grade-Aware
Course Recommendation. Journal of Educational
Data Mining, 11(2):20–46.
Pardos, Z. A., Fan, Z., and Jiang, W. (2019). Connec-
tionist recommendation in the wild: on the utility and
scrutability of neural networks for personalized course
guidance. User Modeling and User-Adapted Interac-
tion, 29(2):487–525.
Pardos, Z. A. and Jiang, W. (2020). Designing for serendip-
ity in a university course recommendation system. In
Proceedings of the 10th International Conference on
Learning Analytics & Knowledge (LAK), pages 350–
359, New York, NY, USA. Association for Computing
Machinery.
Polyzou, A., Nikolakopoulos, A. N., and Karypis, G.
(2019). Scholars Walk: A Markov Chain Framework
for Course Recommendation. In Proceedings of the
12th International Conference on Educational Data
Mining (EDM), pages 396–401, Montreal, Canada.
International Educational Data Mining Society.
Statistisches Bundesamt (2023). Pr
¨
ufungen an
Hochschulen: Deutschland, Jahre, Nationalit
¨
at,
Geschlecht, Pr
¨
ufungsergebnis [Examinations at
universities: Germany, years, nationality, gender,
examination result].
Urdaneta-Ponte, M. C., Mendez-Zorrilla, A., and
Oleagordia-Ruiz, I. (2021). Recommendation
Systems for Education: Systematic Review. Electron-
ics, 10(14):1611.
Wagner, K., Merceron, A., Sauer, P., and Pinkwart, N.
(2023). Can the Paths of Successful Students Help
Other Students With Their Course Enrollments? In
Proceedings of the 16th International Conference on
Educational Data Mining (EDM), pages 171–182,
Bengaluru, India. International Educational Data Min-
ing Society.
CSEDU 2024 - 16th International Conference on Computer Supported Education
246