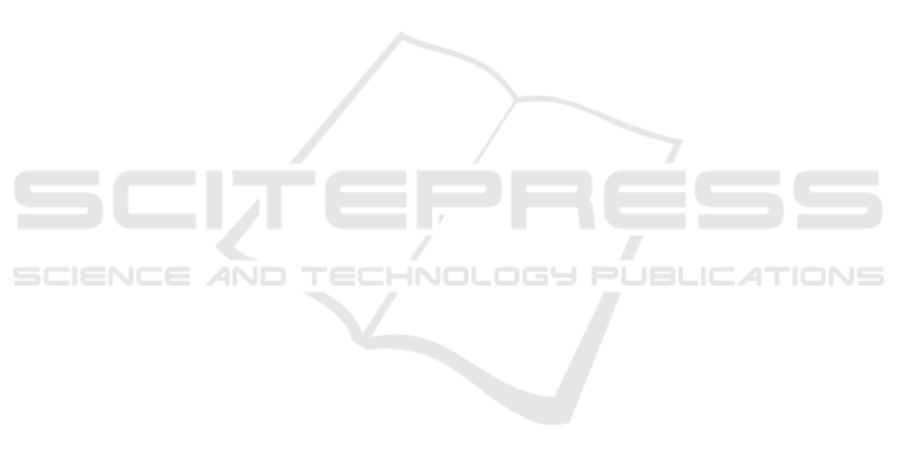
cycle mode. Finally, a carried-out paired t-test with a
resulting p-value of 2.2e-16 ensured the statistical sig-
nificance of the observed differences, affirming that
it cannot be attributed to random fluctuations. How-
ever, it is essential to evaluate each routing service
separately to ensure that routing results based on cal-
ibrated parametrizations improve travel satisfaction.
5 CONCLUSIONS
Individual mobility is pivotal for societal well-being,
and multimodal transportation offers an efficient al-
ternative to exclusive car use in urban areas. The
process of personalizing travel suggestions based on
diverse preferences can enhance the attractiveness of
multimodal transportation. The proposed multi-stage
personalization approach exhibits the capability to ef-
ficiently integrate a broad range of mobility prefer-
ences and routing services. Leveraging the adapter
pattern makes it highly adaptable for different regions
worldwide. New operational regions can be inte-
grated by including additional routing services and
data sources in the system. The proposed routing cal-
ibration approach helps establish utility-maximizing
mapping rules for each preference profile and routing
service, which is particularly important for efficiently
exploiting the personalization capabilities of highly
customizable routing services. Additionally, a utility-
based comparison of routing options tailored to indi-
vidual users promises an enhanced user experience.
However, challenges persist, including managing ex-
tensive preferences, estimating their significance, and
ensuring data availability. An additional critical issue
is the quality of data, which can be addressed through
crowdsensing. The platform’s route recommendation
quality will improve with more users, mitigating the
need for a physical route assessment. Despite these
complexities, our proposed approach has the poten-
tial to enable the transition towards more personalized
and efficient itinerary recommendations in the realm
of mobility services.
ACKNOWLEDGEMENTS
The content of this paper is the result of the project
“MobAPlan - Mobility and Activity-based Planning
Assistant”. This research and development project is
funded by the Vector Stiftung (Vector Foundation).
REFERENCES
Arentze, T. A. (2013). Adaptive personalized travel infor-
mation systems: A bayesian method to learn users’
personal preferences in multimodal transport net-
works. IEEE Transactions on intelligent transporta-
tion systems, 14(4):1957–1966.
Arentze, T. A. and Molin, E. J. E. (2013). Travelers’
preferences in multimodal networks: Design and re-
sults of a comprehensive series of choice experiments.
Transportation Research Part A: Policy and Practice,
58:15–28.
Campigotto, P., Rudloff, C., Leodolter, M., and Bauer, D.
(2016). Personalized and situation-aware multimodal
route recommendations: the favour algorithm. IEEE
Transactions on Intelligent Transportation Systems,
18(1):92–102.
Eszterg
´
ar-Kiss, D., Ansariyar, A., and Katona, G. (2022).
Interconnecting separate transportation systems by in-
troducing exchange points. In 2022 IEEE Interna-
tional Smart Cities Conference (ISC2), pages 1–6.
IEEE.
Gamma, E. (1995). Design patterns. Pearson Education
India.
Lathia, N., Capra, L., Magliocchetti, D., De Vigili, F.,
Conti, G., De Amicis, R., Arentze, T., Zhang, J., Cal
`
ı,
D., and Alexa, V. (2012). Personalizing mobile travel
information services. Procedia-Social and Behavioral
Sciences, 48:1195–1204.
Louviere, J. J., Street, D., Burgess, L., Wasi, N., Islam, T.,
and Marley, A. A. J. (2008). Modeling the choices
of individual decision-makers by combining efficient
choice experiment designs with extra preference infor-
mation. Journal of Choice Modelling, 1(1):128–164.
Morris, M. D. (1991). Factorial sampling plans for pre-
liminary computational experiments. Technometrics,
33(2):161–174.
Nuzzolo, A. and Comi, A. (2016). Individual utility-based
path suggestions in transit trip planners. IET Intelli-
gent Transport Systems, 10(4):219–226.
OpenTripPlanner (2024). Multimodal trip planning. https:
//www.opentripplanner.org/. Accessed: Jan. 3, 2024.
Spitadakis, V. and Fostieri, M. (2012). Wisetrip-
international multimodal journey planning and deliv-
ery of personalized trip information. Procedia-Social
and Behavioral Sciences, 48:1294–1303.
Teodoro, G., Kurc¸, T. M., Taveira, L. F., Melo, A. C., Gao,
Y., Kong, J., and Saltz, J. H. (2017). Algorithm sen-
sitivity analysis and parameter tuning for tissue image
segmentation pipelines. Bioinformatics, 33(7):1064–
1072.
Wins, A., Barthelmes, L., Alpers, S., Becker, C., Kager-
bauer, M., and Oberweis, A. (2024). Personalized
day-trip planning: A TSP-TW-based multimodal mul-
ticriteria optimisation approach. Procedia Computer
Science (In Press).
VEHITS 2024 - 10th International Conference on Vehicle Technology and Intelligent Transport Systems
326