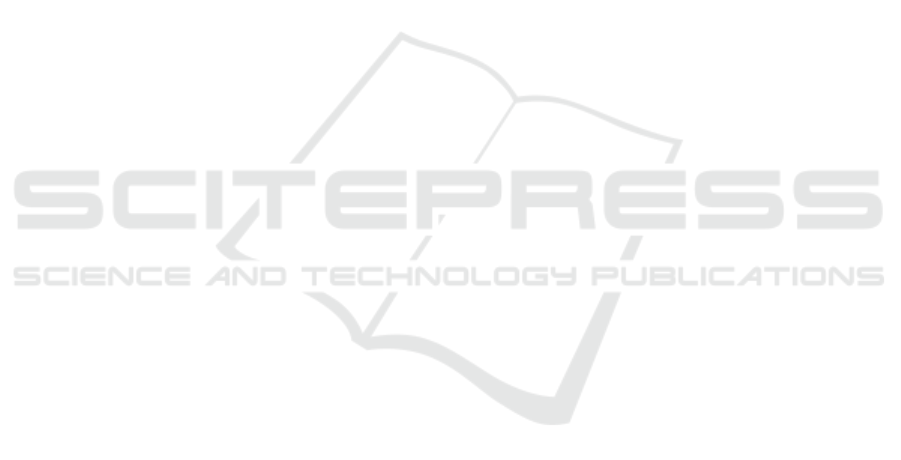
proaches and empirical comparison of traditional al-
gorithms. Applied Energy, 221:386–405.
Luo, C., Wu, D., and Wu, D. (2017). A deep learn-
ing approach for credit scoring using credit default
swaps. Engineering Applications of Artificial Intel-
ligence, 65:465–470.
L’heureux, A., Grolinger, K., Elyamany, H. F., and Capretz,
M. A. (2017). Machine learning with big data: Chal-
lenges and approaches. Ieee Access, 5:7776–7797.
Moula, F. E., Guotai, C., and Abedin, M. Z. (2017). Credit
default prediction modeling: an application of support
vector machine. Risk Management, 19:158–187.
Mukhoti, J., Kulharia, V., Sanyal, A., Golodetz, S., Torr,
P., and Dokania, P. (2020). Calibrating deep neural
networks using focal loss. Advances in Neural Infor-
mation Processing Systems, 33:15288–15299.
Navas-Palencia, G. (2020a). Github optbinning
repository, https://github.com/guillermo-navas-
palencia/optbinning.
Navas-Palencia, G. (2020b). Optimal binning: mathemati-
cal programming formulation. abs/2001.08025.
´
Oskarsd
´
ottir, M., Bravo, C., Sarraute, C., Vanthienen, J.,
and Baesens, B. (2019). The value of big data for
credit scoring: Enhancing financial inclusion using
mobile phone data and social network analytics. Ap-
plied Soft Computing, 74:26–39.
Parola., M., Dirrhami., H., Cimino., M., and Squeglia.,
N. (2023). Effects of environmental conditions on
historic buildings: Interpretable versus accurate ex-
ploratory data analysis. In Proceedings of the 12th In-
ternational Conference on Data Science, Technology
and Applications - DATA, pages 429–435. INSTICC,
SciTePress.
Parola, M., Galatolo, F. A., Torzoni, M., and Cimino, M.
G. C. A. (2023a). Convolutional neural networks
for structural damage localization on digital twins.
In Fred, A., Sansone, C., Gusikhin, O., and Madani,
K., editors, Deep Learning Theory and Applications,
pages 78–97, Cham. Springer Nature Switzerland.
Parola., M., Galatolo., F. A., Torzoni., M., Cimino., M. G.
C. A., and Vaglini., G. (2022). Structural damage
localization via deep learning and iot enabled digital
twin. In Proceedings of the 3rd International Con-
ference on Deep Learning Theory and Applications -
DeLTA, pages 199–206. INSTICC, SciTePress.
Parola, M., Mantia, G. L., Galatolo, F., Cimino, M. G.,
Campisi, G., and Di Fede, O. (2023b). Image-based
screening of oral cancer via deep ensemble architec-
ture. In 2023 IEEE Symposium Series on Computa-
tional Intelligence (SSCI), pages 1572–1578.
Raymaekers, J., Verbeke, W., and Verdonck, T. (2022).
Weight-of-evidence through shrinkage and spline bin-
ning for interpretable nonlinear classification. Applied
Soft Computing, 115:108160.
Sudjianto, A., Nair, S., Yuan, M., Zhang, A., Kern, D., and
Cela-D
´
ıaz, F. (2010). Statistical methods for fighting
financial crimes. Technometrics, 52(1):5–19.
Teles, G., Rodrigues, J. J., Saleem, K., Kozlov, S., and
Rab
ˆ
elo, R. A. (2020). Machine learning and decision
support system on credit scoring. Neural Computing
and Applications, 32:9809–9826.
Thomas, L., Crook, J., and Edelman, D. (2017). Credit
scoring and its applications. SIAM.
Verbraken, T., Bravo, C., Weber, R., and Baesens, B.
(2014a). Development and application of consumer
credit scoring models using profit-based classifica-
tion measures. European Journal of Operational Re-
search, 238(2):505–513.
Verbraken, T., Bravo, C., Weber, R., and Baesens, B.
(2014b). Development and application of consumer
credit scoring models using profit-based classifica-
tion measures. European Journal of Operational Re-
search, 238(2):505–513.
Verma, S., Pant, M., and Snasel, V. (2021). A comprehen-
sive review on nsga-ii for multi-objective combina-
torial optimization problems. Ieee Access, 9:57757–
57791.
Zeng, G. (2014). A necessary condition for a good bin-
ning algorithm in credit scoring. Applied Mathemati-
cal Sciences, 8(65):3229–3242.
ICEIS 2024 - 26th International Conference on Enterprise Information Systems
720