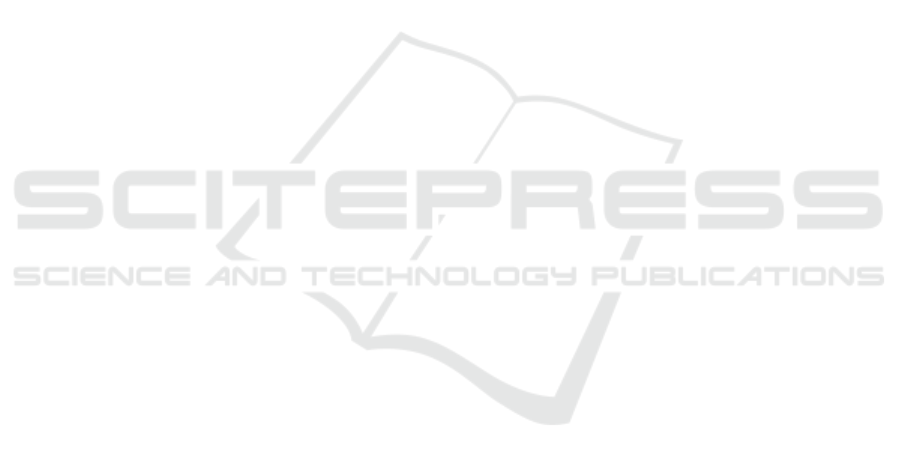
Neal, J. E., Sesterhenn, I. A., Troncoso, P., and
Wheeler, T. M. (1995). Interobserver reproducibil-
ity in the diagnosis of prostatic intraepithelial neo-
plasia. The American Journal of Surgical Pathology,
19(8):873–886.
Gildenblat, J. and contributors (2021). Pytorch li-
brary for cam methods. https://github.com/jacobgil/
pytorch-grad-cam.
Hor
´
ak, J., Furmanov
´
a, K., Kozl
´
ıkov
´
a, B., Br
´
azdil, T.,
Holub, P., Ka
ˇ
cenga, M., Gallo, M., Nenutil, R., By
ˇ
ska,
J., and Rus
ˇ
n
´
ak, V. (2023). xopat: explainable open
pathology analysis tool. Computer Graphics Forum,
42(3):63–73.
Kartasalo, K., Bulten, W., Delahunt, B., et al. (2021). Ar-
tificial intelligence for diagnosis and gleason grading
of prostate cancer in biopsies—current status and next
steps. European Urology Focus, 7(4):687–691.
K
¨
ollermann, J., Hoeh, B., Ruppel, D., Smith, K., Reis,
H., Wenzel, M., Preisser, F., Kosiba, M., Mandel, P.,
Karakiewicz, P. I., Becker, A., Chun, F. K. H., Wild,
P., and Kluth, L. A. (2022). The significance of the ex-
tent of tissue embedding for the detection of inciden-
tal prostate carcinoma on transurethral prostate resec-
tion material: the more, the better? Virchows Archiv,
481(3):387–396.
Lu, M. Y., Williamson, D. F., Chen, T. Y., Chen, R. J., Bar-
bieri, M., and Mahmood, F. (2021). Data-efficient
and weakly supervised computational pathology on
whole-slide images. Nature Biomedical Engineering,
5(6):555–570.
Matsakis, N. D. and Klock II, F. S. (2014). The rust lan-
guage. In ACM SIGAda Ada Letters, volume 34, pages
103–104. ACM.
Nagpal, K., Foote, D., Liu, Y., Chen, P.-H. C., Wulczyn, E.,
Tan, F., Olson, N., Smith, J. L., Mohtashamian, A.,
Wren, J. H., Corrado, G. S., MacDonald, R., Peng,
L. H., Amin, M. B., Evans, A. J., Sangoi, A. R., Mer-
mel, C. H., Hipp, J. D., and Stumpe, M. C. (2019). De-
velopment and validation of a deep learning algorithm
for improving gleason scoring of prostate cancer. npj
Digital Medicine, 2(1).
Oquab, M., Darcet, T., Moutakanni, T., et al. (2023). Di-
nov2: Learning robust visual features without super-
vision. CoRR, abs/2304.07193.
Park, T., Efros, A. A., Zhang, R., and Zhu, J.-Y. (2020).
Contrastive learning for unpaired image-to-image
translation. CoRR, abs/2007.15651.
Perincheri, S., Levi, A. W., Celli, R., Gershkovich, P.,
Rimm, D., Morrow, J. S., Rothrock, B., Raciti, P.,
Klimstra, D., and Sinard, J. (2021). An indepen-
dent assessment of an artificial intelligence system for
prostate cancer detection shows strong diagnostic ac-
curacy. Modern Pathology, 34(8):1588–1595.
Radboud Computational Pathology Group and ASAP con-
tributors (2009). Automated slide analysis platform.
https://github.com/computationalpathologygroup/
ASAP.
Sch
¨
uffler, P. J., Stamelos, E., Ahmed, I., Yarlagadda, D.
V. K., Ardon, O., Hanna, M. G., Reuter, V. E., Klim-
stra, D. S., and Hameed, M. (2022). Efficient visu-
alization of whole slide images in web-based viewers
for digital pathology. Archives of Pathology and Lab-
oratory Medicine, 146(10):1273–1280.
Singhal, N., Soni, S., Bonthu, S., Chattopadhyay, N.,
Samanta, P., Joshi, U., Jojera, A., Chharchhodawala,
T., Agarwal, A., Desai, M., and Ganpule, A. (2022). A
deep learning system for prostate cancer diagnosis and
grading in whole slide images of core needle biopsies.
Scientific Reports, 12(1).
Tolkach, Y., Dohmg
¨
orgen, T., Toma, M., and Kristiansen,
G. (2020). High-accuracy prostate cancer pathology
using deep learning. Nature Machine Intelligence,
2(7):411–418.
Vaswani, A., Shazeer, N., Parmar, N., et al. (2017). Atten-
tion is all you need. In Guyon, I., Luxburg, U. V.,
Bengio, S., Wallach, H., Fergus, R., Vishwanathan,
S., and Garnett, R., editors, Advances in Neural Infor-
mation Processing Systems, volume 30. Curran Asso-
ciates, Inc.
Yagi, Y., Yoshioka, S., Kyusojin, H., Onozato, M., Mizu-
tani, Y., Osato, K., Yada, H., Mark, E. J., Frosch,
M. P., and Louis, D. N. (2012). An ultra-high speed
whole slide image viewing system. Analytical Cellu-
lar Pathology, 35(1):65–73.
Yang, Z., Wang, X., Xiang, J., Zhang, J., Yang, S.,
Wang, X., Yang, W., Li, Z., Han, X., and Liu, Y.
(2023). The devil is in the details: a small-lesion
sensitive weakly supervised learning framework for
prostate cancer detection and grading. Virchows
Archiv, 482(3):525–538.
Ye, H.-L. and Wang, D.-H. (2022). Stain-adaptive self-
supervised learning for histopathology image analy-
sis. CoRR, abs/2208.04017.
Zuley, M., Jarosz, R., Drake, B., et al. (2016). The can-
cer genome atlas prostate adenocarcinoma collection
(tcga-prad). The Cancer Imaging Archive, last ac-
cessed 15.01.23.
ICEIS 2024 - 26th International Conference on Enterprise Information Systems
738