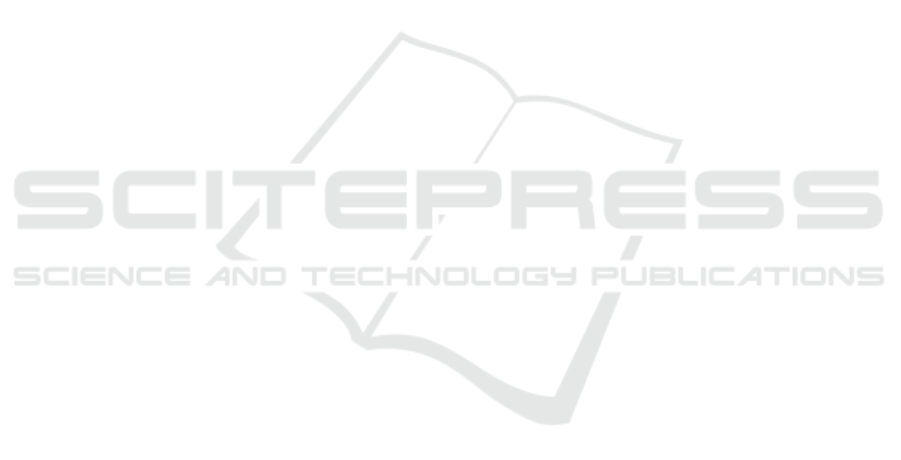
X., and Gao, W. (2010). Wld: A robust local image
descriptor. In IEEE Transactions on Pattern Analysis
and Machine Intelligence, pages 1705–1720.
Cho, Y., Lee, G., Kim, K., and Zawadzki, I. (2006). Identi-
fication and removal of ground echoes and anomalous
propagation using the characteristics of radar echoes.
pages 1206–1222.
Cortes, C. and Vapnik, V. (1995). Support-vector networks.
pages 273–297.
Fathi, M., Kashani, M., Jameii, S., and Mahdipour, E.
(2022). Big data analytics in weather forecasting: A
systematic review. pages 1247–1275.
Haddad, B., Adane, A., Mesnard, F., and Sauvageot, H.
(2000). Modeling anomalous radar propagation using
first-order two-state markov chains. pages 283–292.
Haddad, B., Adane, A., Sauvageot, H., Sadouki, L., and
Naili, R. (2004). Identification and filtering of rainfall
and ground radar echoes using textural features. pages
4641–4656.
Hedir, M., Demim, F., and Haddad, B. (2018). Radar echoes
classification based on local descriptor. In IEEE In-
ternational Conference on Signal, Image, Vision and
their Applications, pages 1–6.
Hedir, M., Demim, F., and Haddad, B. (2019). A compara-
tive analysis of the ground clutter suppression on me-
teorological images. In IEEE 6th International Con-
ference on Image and Signal Processing and their Ap-
plications, Mostaganem, Algeria, pages 1–7.
Hedir, M. and Haddad, B. (2016). Automatic system for
radar echoes filtering based on textural features and
artificial intelligence.
Islam, T., Rico-Ramirez, M., Han, D., and Srivastava, P.
(2012). Artificial intelligence techniques for clut-
ter identification with polarimetric radar signatures.
pages 95–113.
Kaissassou, S., Lenouo, A., Tchawoua, C., Lopez, P., and
Gaye, A. (2015). Climatology of radar anomalous
propagation over west africa. pages 1–12.
Liu, F., Tang, Z., and Tang, J. (2013). Weber local binary
pattern for local image description. pages 325–335.
Malki, Z., Atlam, E., Hassanien, A., Dagnew, G., Elhos-
seini, M., and Gad, I. (2020). Association between
weather data and covid-19 pandemic predicting mor-
tality rate: Machine learning approaches. pages 110–
137.
Ojala, T., Pietikainen, M., and Maenpaa, T. (2002). Mul-
tiresolution grey-scale and rotation invariant texture
classification with local binary patterns. pages 971–
987.
Paixao, E., Mirza, M., Shephard, M., Auld, H., Klaassen,
J., and Smith, G. (2015). An integrated approach
for identifying homogeneous regions of extreme rain-
fall events and estimating IDF curves in Southern On-
tario, Canada: Incorporating radar observations.
Rouigueb, A., Demim, F., Belaidi, H., Messaoui, A., Bena-
tia, M., and Djamaa, B. (2023). Improving the license
plate character segmentation using na
˜
A¯ve bayesian
network. In Proceedings of the 20th International
Conference on Informatics in Control, Automation
and Robotics (ICINCO 2023), Rome, Italy, pages 61–
68. Giuseppina Gini; Henk Nijmeijer and Dimitar
Filev.
Rouigueb, A., Demim, F., Nemra, A., and Djamaa, B.
(2022). Gearbox fault diagnosis using the short-time
cepstral features. In Proceedings of the 2nd IEEE In-
ternational Conference on Advanced Electrical Engi-
neering (ICAEE), pages 1–8. IEEE.
SIMULTECH 2024 - 14th International Conference on Simulation and Modeling Methodologies, Technologies and Applications
26