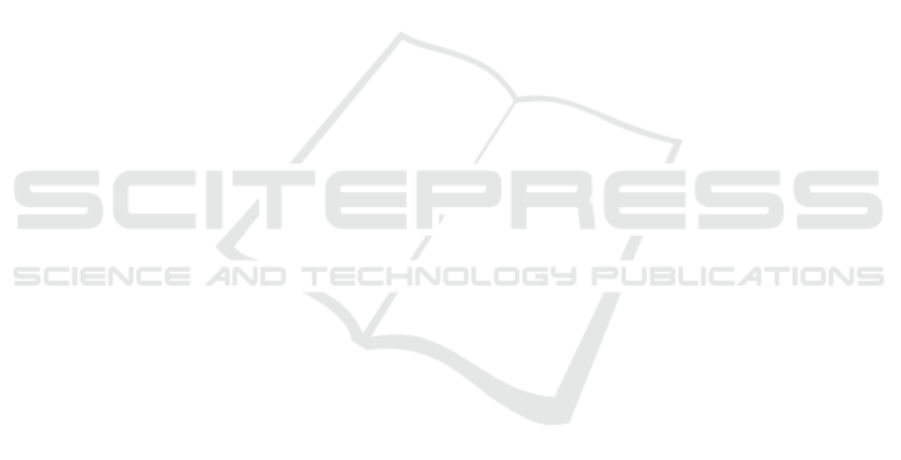
(maas) system. Technical report, MaaS Global Ram-
boll.
Hemminki, S., Nurmi, P., and Tarkoma, S. (2013).
Accelerometer-based transportation mode detection
on smartphones. In Proceedings of the 11th ACM
Conference on Embedded Networked Sensor Systems.
Association for Computing Machinery.
Hensher, D. A., Ho, C. Q., Mulley, C., Nelson, J. D., Smith,
G., and Wong, Y. Z. (2020). Understanding Mobility
as a Service (MaaS): Past, Present and Future. Else-
vier.
Hietanen, S. (2014). Mobility as a service - the new trans-
port model? Eurotransport-ITS & Transport Manage-
ment Supplement, 12(2):2–4.
House of Commons Transport Committee (2018). Mobil-
ity as a service : Eighth report of session 2017–19.
Technical report, U.K. Parliament.
Jittrapirom, P., Caiati, V., Feneri, A.-M., Ebrahimighare-
hbaghi, S., Gonz
´
alez, M., and Narayan, J. (2017). Mo-
bility as a service: A critical review of definitions,
assessments of schemes, and key challenges. Urban
Planning, 2(2):13–25.
Kamargianni, M., Matyas, M., Li, W., Muscat, J., and Yfan-
tis, L. (2018). The maas dictionary. Technical report,
MaaSLab, Energy Institute, University College Lon-
don.
Klinkm
¨
uller, C. and Weber, I. (2021). Every apprentice
needs a master: Feedback-based effectiveness im-
provements for process model matching. Information
Systems, 95:101612.
Krempl, G.,
ˇ
Zliobaite, I., Brzezi
´
nski, D., H
¨
ullermeier, E.,
Last, M., Lemaire, V., Noack, T., Shaker, A., Sievi, S.,
Spiliopoulou, M., and Stefanowski, J. (2014). Open
challenges for data stream mining research. SIGKDD
Explor. Newsl., 16(1):1–10.
Lyons, G., Hammond, P., and Mackay, K. (2019). The im-
portance of user perspective in the evolution of maas.
Transportation Research Part A: Policy and Practice,
121:22–36.
Lyu, L., Yu, H., and Yang, Q. (2020). Threats to federated
learning: A survey. arXiv preprint arXiv:2003.02133.
Maas, B. (2022). Literature review of mobility as a service.
Sustainability, 14(14).
MaaS4EU (2020). Horizon2020 MaaS4EU Project.
https://cordis.europa.eu/project/id/723176. Accessed
January 2024.
Mach, P. and Becvar, Z. (2017). Mobile edge comput-
ing: A survey on architecture and computation of-
floading. IEEE Communications Surveys & Tutorials,
19(3):1628–1656.
McNee, S. M., Riedl, J., and Konstan, J. A. (2006). Be-
ing accurate is not enough: How accuracy metrics
have hurt recommender systems. In CHI ’06 Extended
Abstracts on Human Factors in Computing Systems,
page 1097–1101.
Melis, A., Mirri, S., Prandi, C., Prandini, M., Salomoni,
P., and Callegati, F. (2018). Integrating personalized
and accessible itineraries in maas ecosystems through
microservices. Mobile Networks and Applications,
23(1):167–176.
Mulvenna, M., Annual, S., and Buchner, A. (2000). Per-
sonalization on the net using web mining. Communi-
cation of the ACM, 43(8):122–125.
Opiola, J. (2018). Defining levels of maas. Traffic Technol-
ogy International, pages 70–71.
Perugini, S. and Ramakrishnan, N. (2003). Personalizing
web sites with mixed-initiative interaction. IT Profes-
sional, 5(2):9–15.
Polydoropoulou, A., Pagoni, I., and Tsirimpa, A. (2020).
Ready for mobility as a service? insights from stake-
holders and end-users. Travel Behaviour and Society,
21:295–306.
Prost, S., Schrammel, J., R
¨
oderer, K., and Tscheligi, M.
(2013). Contextualise! personalise! persuade! a mo-
bile hci framework for behaviour change support sys-
tems. In Proceedings of the 15th International Con-
ference on Human-Computer Interaction with Mobile
Devices and Services, page 510–515. Association for
Computing Machinery.
Ranaweera, P., Jurcut, A. D., and Liyanage, M. (2021).
Survey on multi-access edge computing security and
privacy. IEEE Communications Surveys & Tutorials,
23(2):1078–1124.
Signor, L., Karjalainen, P., Kamargianni, M., Matyas, M.,
et al. (2019). Mobility as a Service (MaaS) and Sus-
tainable Urban Mobility Planning. Technical report,
ERTICO – ITS Europe.
Smith, D. (2019). Navigogo: Scotland’s first maas pilot.
Technical report, ESP Group.
Sochor, J., Arby, H., Karlsson, I. M., and Sarasini, S.
(2018). A topological approach to mobility as a ser-
vice: A proposed tool for understanding requirements
and effects, and for aiding the integration of societal
goals. Research in Transportation Business & Man-
agement, 27:3–14.
Xie, H., Song, X., and Zhang, H. (2022). Maas and iot:
Concepts, methodologies, and applications. In Zhang,
H., Song, X., and Shibasaki, R., editors, Big Data and
Mobility as a Service, pages 229–243. Elsevier.
Zafar, F., Khattak, H. A., Aloqaily, M., and Hussain, R.
(2022). Carpooling in connected and autonomous ve-
hicles: Current solutions and future directions. ACM
Computing Surveys, 54(10s).
Zehlike, M., Yang, K., and Stoyanovich, J. (2022). Fairness
in ranking, part ii: Learning-to-rank and recommender
systems. ACM Computing Surveys, 55(6).
Zhang, C., Xie, Y., Bai, H., Yu, B., Li, W., and Gao, Y.
(2021). A survey on federated learning. Knowledge-
Based Systems, 216:106775.
Zhang, D., Chang, Z., Yang, D., Li, D., Tan, K.-L., Chen,
K., and Chen, G. (2023). Squid: subtrajectory query
in trillion-scale GPS database. The VLDB Journal.
Zheng, Y. (2015). Trajectory data mining: An overview.
ACM Transactions on Intelligent Systems and Tech-
nology, 6(3).
Ziarani, R. J. and Ravanmehr, R. (2021). Serendipity
in recommender systems: A systematic literature re-
view. Journal of Computer Science and Technology,
36(2):375–396.
VEHITS 2024 - 10th International Conference on Vehicle Technology and Intelligent Transport Systems
360