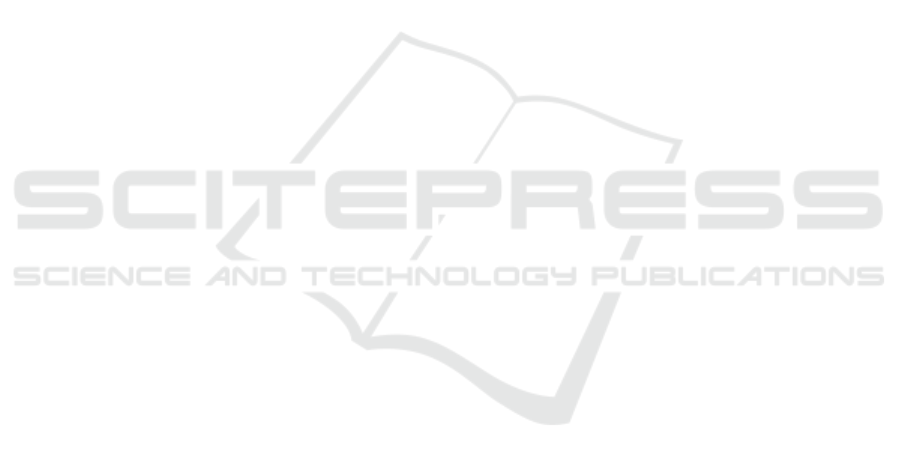
bersecurity) and SERICS (PE00000014) under the
MUR National Recovery and Resilience Plan funded
by the EU - NextGenerationEU projects.
REFERENCES
Goodfellow, I., Pouget-Abadie, J., Mirza, M., Xu, B.,
Warde-Farley, D., Ozair, S., Courville, A., and Ben-
gio, Y. (2020). Generative adversarial networks. Com-
munications of the ACM, 63(11):139–144.
Huang, P., Li, C., He, P., Xiao, H., Ping, Y., Feng, P., Tian,
S., Chen, H., Mercaldo, F., Santone, A., et al. (2024a).
Mamlformer: Priori-experience guiding transformer
network via manifold adversarial multi-modal learn-
ing for laryngeal histopathological grading. Informa-
tion Fusion, page 102333.
Huang, P., Tan, X., Zhou, X., Liu, S., Mercaldo, F., and
Santone, A. (2021). Fabnet: fusion attention block
and transfer learning for laryngeal cancer tumor grad-
ing in p63 ihc histopathology images. IEEE Journal
of Biomedical and Health Informatics, 26(4):1696–
1707.
Huang, P., Xiao, H., He, P., Li, C., Guo, X., Tian, S.,
Feng, P., Chen, H., Sun, Y., Mercaldo, F., et al.
(2024b). La-vit: A network with transformers con-
strained by learned-parameter-free attention for in-
terpretable grading in a new laryngeal histopathol-
ogy image dataset. IEEE Journal of Biomedical and
Health Informatics.
Huang, P., Zhou, X., He, P., Feng, P., Tian, S., Sun, Y., Mer-
caldo, F., Santone, A., Qin, J., and Xiao, H. (2023).
Interpretable laryngeal tumor grading of histopatho-
logical images via depth domain adaptive network
with integration gradient cam and priori experience-
guided attention. Computers in Biology and Medicine,
154:106447.
Iadarola, G., Martinelli, F., Mercaldo, F., and Santone, A.
(2021). Towards an interpretable deep learning model
for mobile malware detection and family identifica-
tion. Computers & Security, 105:102198.
Li, Y., Jang, J., Hu, X., and Ou, X. (2017). Android mal-
ware clustering through malicious payload mining. In
Research in Attacks, Intrusions, and Defenses: 20th
International Symposium, RAID 2017, Atlanta, GA,
USA, September 18–20, 2017, Proceedings, pages
192–214. Springer.
Mercaldo, F., Martinelli, F., and Santone, A. (2021). A pro-
posal to ensure social distancing with deep learning-
based object detection. In 2021 International Joint
Conference on Neural Networks (IJCNN), pages 1–5.
IEEE.
Mercaldo, F. and Santone, A. (2020). Deep learning
for image-based mobile malware detection. Jour-
nal of Computer Virology and Hacking Techniques,
16(2):157–171.
Mercaldo, F., Zhou, X., Huang, P., Martinelli, F., and San-
tone, A. (2022). Machine learning for uterine cervix
screening. In 2022 IEEE 22nd International Confer-
ence on Bioinformatics and Bioengineering (BIBE),
pages 71–74. IEEE.
Nguyen, H., Di Troia, F., Ishigaki, G., and Stamp, M.
(2023). Generative adversarial networks and image-
based malware classification. Journal of Computer
Virology and Hacking Techniques, pages 1–17.
Radford, A., Metz, L., and Chintala, S. (2015). Unsu-
pervised representation learning with deep convolu-
tional generative adversarial networks. arXiv preprint
arXiv:1511.06434.
Sun, X., Li, L., Mercaldo, F., Yang, Y., Santone, A., and
Martinelli, F. (2021). Automated intention mining
with comparatively fine-tuning bert. In Proceedings
of the 2021 5th International Conference on Natu-
ral Language Processing and Information Retrieval,
pages 157–162.
Zhou, X., Tang, C., Huang, P., Tian, S., Mercaldo, F., and
Santone, A. (2023). Asi-dbnet: an adaptive sparse
interactive resnet-vision transformer dual-branch net-
work for the grading of brain cancer histopathological
images. Interdisciplinary Sciences: Computational
Life Sciences, 15(1):15–31.
Evaluating the Impact of Generative Adversarial Network in Android Malware Detection
597