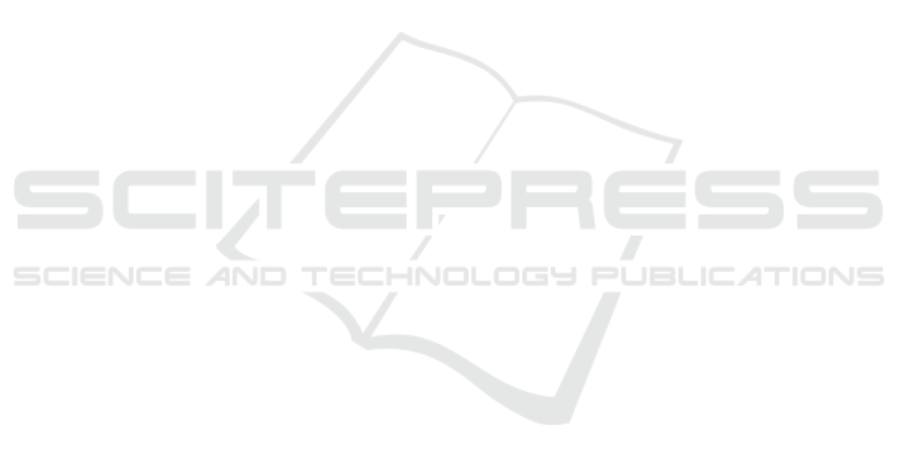
Focusing on the banking ’getLoanDecision’ algo-
rithm, we’ve unveiled the complexities of algorith-
mic decisions and different stakeholders in finance.
This model handles diverse scenarios, from loan ap-
plications to ethical dilemmas, exemplifying its abil-
ity to streamline customer service and operational ef-
ficiency. However, it also raises ethical issues, like
the use of ZIP codes in decision-making, highlighting
the importance of ethics in algorithm development.
Addressing such concerns proactively, including re-
ferring them to an ethical committee, demonstrates
RAGADA’s potential in managing sensitive matters,
crucial for trust and transparency in banking. Over-
all, RAGADA’s nuanced decision-making approach
offers promising prospects for evolving financial ser-
vices.
6 CONCLUSION AND FUTURE
WORK
This research introduces and validates the RAGADA
model, a novel AI-based software architecture en-
hancing corporate decision-making. Successfully im-
plemented in the banking sector, RAGADA leverages
natural language interfaces to facilitate complex deci-
sions, demonstrating significant advancements in AI
and corporate governance. It has shown potential in
improving decision accuracy, user satisfaction, and
strategic adaptability. Future exploration includes ex-
tending RAGADA’s applicability to various sectors
and enhancing its capabilities for more complex sce-
narios. Emphasizing ethical AI and user-centric de-
sign, the model presents a substantial progression in
AI-integrated corporate decision-making, offering a
blueprint for future advancements in AI-augmented
governance.
REFERENCES
Bender, E. M., Gebru, T., McMillan-Major, A., and
Shmitchell, S. (2021). On the dangers of stochastic
parrots: Can language models be too big? In Pro-
ceedings of the 2021 ACM conference on fairness, ac-
countability, and transparency, pages 610–623.
Borgeaud, S., Mensch, A., Hoffmann, J., Cai, T., Ruther-
ford, E., Millican, K., Van Den Driessche, G. B.,
Lespiau, J.-B., Damoc, B., Clark, A., et al. (2022).
Improving language models by retrieving from tril-
lions of tokens. In International conference on ma-
chine learning, pages 2206–2240. PMLR.
Brown, T., Mann, B., Ryder, N., Subbiah, M., Kaplan, J. D.,
Dhariwal, P., Neelakantan, A., Shyam, P., Sastry, G.,
Askell, A., et al. (2020). Language models are few-
shot learners. Advances in neural information pro-
cessing systems, 33:1877–1901.
Christian, B. (2020). The alignment problem: Machine
learning and human values. WW Norton & Company.
ISBN: 9781786494306.
Dash, R., Kremer, A., and Petrov, A. (2021). Designing
next-generation credit-decisioning models. McKinsey
& Company, White paper, Dec.
Devlin, J., Chang, M.-W., Lee, K., and Toutanova, K.
(2018). Bert: Pre-training of deep bidirectional trans-
formers for language understanding. arXiv preprint
arXiv:1810.04805.
Guu, K., Lee, K., Tung, Z., Pasupat, P., and Chang, M.
(2020). Retrieval augmented language model pre-
training. In International conference on machine
learning, pages 3929–3938. PMLR.
Kaplan, R. S. (1992). The balanced scorecard measures
that drive performance. Harvard business review.
Karpukhin, V., O
˘
guz, B., Min, S., Lewis, P., Wu, L.,
Edunov, S., Chen, D., and Yih, W.-t. (2020). Dense
passage retrieval for open-domain question answer-
ing. arXiv preprint arXiv:2004.04906.
Lewis, P., Perez, E., Piktus, A., Petroni, F., Karpukhin,
V., Goyal, N., K
¨
uttler, H., Lewis, M., Yih, W.-t.,
Rockt
¨
aschel, T., et al. (2020). Retrieval-augmented
generation for knowledge-intensive nlp tasks. Ad-
vances in Neural Information Processing Systems,
33:9459–9474.
Olesen-Bagneux, O. (2023). Enterprise Data Catalog.
O’Reilly Media. ISBN: 149209871X.
Petroni, F., Rockt
¨
aschel, T., Lewis, P., Bakhtin, A., Wu,
Y., Miller, A. H., and Riedel, S. (2019). Lan-
guage models as knowledge bases? arXiv preprint
arXiv:1909.01066.
Rosenschein, J. S. and Zlotkin, G. (1994). Rules of en-
counter: designing conventions for automated nego-
tiation among computers. MIT press.
Stone, P. and Veloso, M. (2000). Multiagent systems: A sur-
vey from a machine learning perspective. Autonomous
Robots, 8:345–383.
Vaswani, A., Shazeer, N., Parmar, N., Uszkoreit, J., Jones,
L., Gomez, A. N., Kaiser, Ł., and Polosukhin, I.
(2017). Attention is all you need. Advances in neural
information processing systems, 30.
Wilson, E. O. (1999). Consilience: The unity of knowledge.
Vintage; Reprint edition. ISBN: 978-0679768678.
Wooldridge, M. (2009). An introduction to multiagent sys-
tems. John wiley & sons.
Bridging Human and AI Decision-Making with LLMs: The RAGADA Approach
819