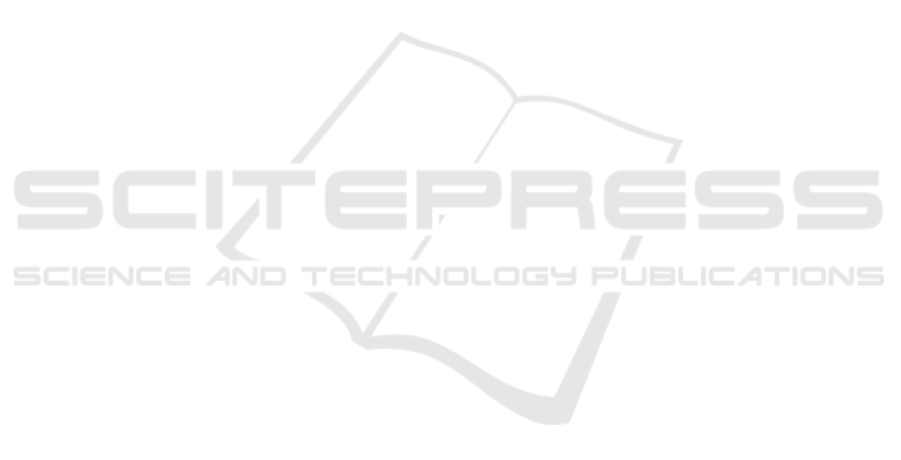
de S
´
a, J. R., da Silva Junior, J. C., Fagundes, K. M.,
Damaceno, L. F., Zajdenverg, L., Calliari, L. E. P.,
Rodacki, M., Montenegro Junior, R. M., Lamounier,
R. N., and Vencio, S. A. C. (2021). Diretriz da So-
ciedade Brasileira de Diabetes. Sociedade Brasileira
de Diabetes.
Brisimi, T. S., Xu, T., Wang, T., Dai, W., and Paschalidis,
I. C. (2019). Predicting diabetes-related hospitaliza-
tions based on electronic health records. Statistical
Methods in Medical Research, 28(12):3667–3682.
Cenitta, D., Arjunan, R. V., and V, P. K. (2022). Ischemic
heart disease multiple imputation technique using ma-
chine learning algorithm. ”Engineered Science, 19
(September 2022):262–272.
Chen, Y., Zhou, Z., Liang, Y., Tan, X., Li, Y., Qin, C., Feng,
Y., Ma, X., Mo, Z., Xia, J., Zhang, H., Qiu, S., and
Shen, D. (2021). Classification of type 2 diabetes
mellitus with or without cognitive impairment from
healthy controls using high-order functional connec-
tivity. Human Brain Mapping, 42(14):4671–4684.
Clarke, W. L., Cox, D., Gonder-Frederick, L. A., Carter, W.,
and Pohl, S. L. (1987). Evaluating Clinical Accuracy
of Systems for Self-Monitoring of Blood Glucose. Di-
abetes Care, 10(5):622–628.
da Costa, C. A., Pasluosta, C. F., Eskofier, B., da Silva,
D. B., and da Rosa Righi, R. (2018). Internet of Health
Things: Toward intelligent vital signs monitoring in
hospital wards. Artificial Intelligence in Medicine,
89(March 2017):61–69.
Dey, A. K., Abowd, G. D., and Salber, D. (2001). A con-
ceptual framework and a toolkit for supporting the
rapid prototyping of context-aware applications. Hu-
man–Computer Interaction, 16(2-4):97–166.
Dworzynski, P., Aasbrenn, M., Rostgaard, K., Melbye, M.,
Gerds, T. A., Hjalgrim, H., and Pers, T. H. (2019). Na-
tionwide prediction of type 2 diabetes comorbidities.
bioRxiv, page 664722.
Ellahham, S. (2020). Artificial intelligence: the future for
diabetes care. The American journal of medicine,
133(8):895–900.
Faruqui, S. H. A., Du, Y., Meka, R., Alaeddini, A., Li,
C., Shirinkam, S., and Wang, J. (2019). Develop-
ment of a deep learning model for dynamic forecast-
ing of blood glucose level for type 2 diabetes mellitus:
Secondary analysis of a randomized controlled trial.
JMIR mHealth and uHealth, 7(11):1–14.
Gao, J., Yi, P., Chi, Z., and Zhu, T. (2017). A smart medical
system for dynamic closed-loop blood glucose-insulin
control. Smart Health, 1-2(February):18–33.
Guariguata, L., Whiting, D. R., Linnenkamp, U., Bea-
gley, J., and Shaw, J. (2014). Global estimates of
the prevalence of impaired glucose tolerance for 2013
and projections to 2035. In Diabetes, volume 63,
pages A391–A391. AMER DIABETES ASSOC 1701
N BEAUREGARD ST, ALEXANDRIA, VA 22311-
1717 USA.
Hudson, L. (1985). Design of the intensive care unit
from a monitoring point of view. Respiratory care,
30(7):549—559.
Javidi, H., Mariam, A., Khademi, G., Zabor, E. C., Zhao,
R., Radivoyevitch, T., and Rotroff, D. M. (2022).
Identification of robust deep neural network models
of longitudinal clinical measurements. npj Digital
Medicine, 5(1):1–11.
Joseph, V. R. (2022). Optimal ratio for data splitting. Sta-
tistical Analysis and Data Mining, 15(4):531–538.
Joseph, V. R. and Vakayil, A. (2022). SPlit: An Optimal
Method for Data Splitting. Technometrics, 64(2):166–
176.
Lee, J.-Y., Won, D., and Lee, K. (2023). Machine learning-
based identification and related features of depression
in patients with diabetes mellitus based on the korea
national health and nutrition examination survey: A
cross-sectional study. PLOS ONE, 18(7):1–11.
Massaro, A., Maritati, V., Giannone, D., Convertini, D.,
and Galiano, A. (2019). LSTM DSS automatism and
dataset optimization for diabetes prediction. Applied
Sciences (Switzerland), 9(17):3532.
Midroni, C., Leimbigler, P. J., Baruah, G., Kolla, M., White-
head, A. J., and Fossat, Y. (2018). Predicting glycemia
in type 1 diabetes patients: Experiments with XG-
Boost. CEUR Workshop Proceedings, 2148:79–84.
Montori, F., Bedogni, L., Felice, M. D., and Bononi, L.
(2018). Machine-to-machine wireless communication
technologies for the Internet of Things: Taxonomy,
comparison and open issues. Pervasive and Mobile
Computing, 50:56–81.
Moura, E. C., Pacheco-Santos, L. M., Peters, L. R., Ser-
ruya, S. J., and Guimar
˜
aes, R. (2012). Research on
chronic noncommunicable diseases in Brazil: meet-
ing the challenges of epidemiologic transition. Revista
Panamericana de Salud P
´
ublica, 31(3):240–245.
Mshali, H., Lemlouma, T., and Magoni, D. (2018). Adap-
tive monitoring system for e-health smart homes. Per-
vasive and Mobile Computing, 43:1–19.
Nadimi-Shahraki, M. H., Mohammadi, S., Zamani, H.,
Gandomi, M., and Gandomi, A. H. (2021). A hybrid
imputation method for multi-pattern missing data: A
case study on type ii diabetes diagnosis. Electronics
(Switzerland), 10(24):3167.
Nanda, R., Nath, A., Patel, S., and Mohapatra, E. (2022).
Machine learning algorithm to evaluate risk factors
of diabetic foot ulcers and its severity. Medical and
Biological Engineering and Computing, 60(8):2349–
2357.
Ogurtsova, K., da Rocha Fernandes, J. D., Huang, Y., Lin-
nenkamp, U., Guariguata, L., Cho, N. H., Cavan, D.,
Shaw, J. E., and Makaroff, L. E. (2017). IDF Diabetes
Atlas: Global estimates for the prevalence of diabetes
for 2015 and 2040. Diabetes Research and Clinical
Practice, 128:40–50.
Pagiatakis, C., Rivest-H
´
enault, D., Roy, D., Thibault, F.,
and Jiang, D. (2020). Intelligent interaction interface
for medical emergencies: Application to mobile hypo-
glycemia management. Smart Health, 15(November
2019).
Pan, H., Sun, J., Luo, X., Ai, H., Zeng, J., Shi, R., and
Zhang, A. (2023). A risk prediction model for type 2
diabetes mellitus complicated with retinopathy based
IoTBDS 2024 - 9th International Conference on Internet of Things, Big Data and Security
282