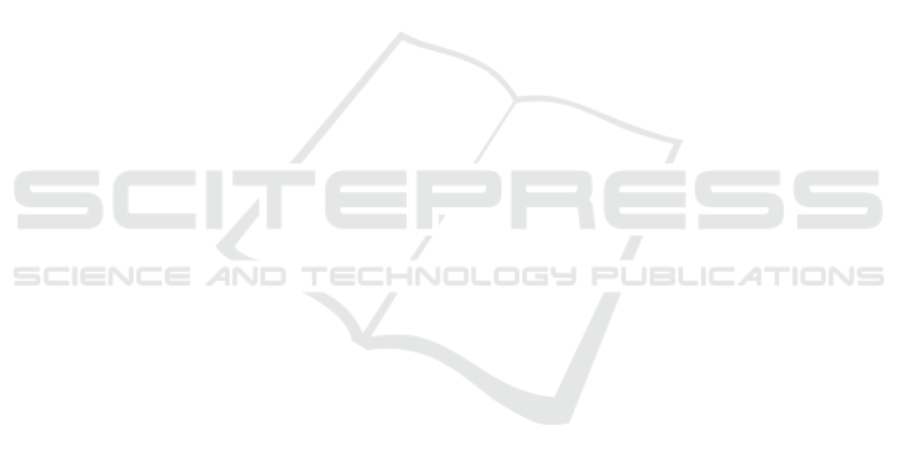
to bookings that take place between 0 and 15 days be-
fore the DOS, i.e., meteorological variables. In fact,
when the price is lower than normal, weather condi-
tions can be decisive in the choice to make a reserva-
tion or not.
5 CONCLUSIONS AND FUTURE
WORKS
In this study we have performed a benchmark of dif-
ferent machine learning methods with the aim to build
a support useful for a revenue manager working on
dynamic prices. Having a well-established dynamic
pricing strategy, the continuous monitoring and man-
ual adjustment of prices performed by a revenue man-
ager becomes costly and time-consuming. For this
purpose we built a dataset containing static, dynamic
and engineered variables and applied five machine
learning models, MLR, RF, LGB, GB and MLP to
predict the dynamic price that a revenue manager
would set every day for the next 90 days for the entry
level room of a receptive structure. The approaches
have been tested on three different hotels. Since it
emerged that the highest errors in terms of MAPE
are concentrated more in the predictions between 0-
15 days and in the lowest price range, in future works
we will try to exploit additional variables that could
influence the decision of the price in these cases.
REFERENCES
Abrate, G., Nicolau, J. L., and Viglia, G. (2019). The impact
of dynamic price variability on revenue maximization.
Tourism Management, vol. 74, pp. 224-233.
Al Shehhi, M. and Karathanasopoulos, A. (2020). Forecast-
ing hotel room prices in selected gcc cities using deep
learning. Journal of Hospitality and Tourism Manage-
ment, vol. 42, pp. 40-50.
Alshakhsheer, F., Habiballah, M., Al-Ababneh, M. M., and
Alhelalat, J. A. (2017). Improving hotel revenue through
the implementation of a comprehensive dynamic pricing
strategy: A conceptual framework and empirical inves-
tigation of jordanian hotels. Business Management Dy-
namics, vol. 7, pp.19-33.
Bandalouski, A. M., Egorova, N. G., Kovalyov, M. Y.,
Pesch, E., and Tarim, S. A. (2021). Dynamic with
demand disaggregation for hotel revenue management.
Journal of Heuristics.
Bayoumi, A., Saleh, M., Atiya, A., and Habib, H. (2013).
Dynamic pricing for hotel revenue management using
price multipliers. Journal of Revenue and Pricing Man-
agement.
Deksnyte, I. and Lydeka, Z. (2012). Dynamic pricing and its
forming factors. Journal of Business and Social Science.
El-Nemr, N., Canel-Depitre, B., and Taghipour, A. (2019).
Determinants of hotel room rates determinants of hotel
room rates. In Luxury Industries Conference.
Elreedy, D., Atiya, A. F., Fayed, H., and Saleh, M. (2019).
A framework for an agent-based dynamic pricing for
broadband wireless price rate plans. Journal of Simu-
lation, vol. 13, pp. 96-110.
Goli, F. and Haghighinasab, M. (2022). Dynamic pricing: A
bibliometric approach. Iranian Journal of Management
Studies, vol. 15, pp. 111-132.
Kalehbasti, P., Nikolenko, L., and Rezaei, H. (2019).
Airbnb price prediction using machine learning and sen-
timent analysis. In CD-MAKE, International Cross-
Domain Conference for Machine Learning and Knowl-
edge Extraction.
Lak, P., Kocak, A., Pralat, P., Bener, A., and Samarikhalaj,
A. (2015). Towards dynamic pricing for digital billboard
advertising network in smart cities. In ISC2, IEEE First
International Smart Cities Conference.
Liu, Y. (2021). Airbnb pricing based statistical machine
learning models. In CONF-SPML, International Confer-
ence on Signal Processing and Machine Learning.
Mariani, M. and Wirtz, J. (2023). A critical reflection
on analytics and artificial intelligence-based in analytics
in hospitality and tourism management research. Inter-
national Journal of Contemporary Hospitality Manage-
ment, vol. 35, pp. 2929-2943.
Pande, R. (2020). Investigation of the implementation of
machine learning within the hospitality industry. In
CAUTHE Conference.
Peng, N., Qin, Y., and Li, K. (2020). Leveraging multi-
modality data to airbnb price prediction. In ICEMME,
2
nd
International Conference on Economic Management
and Model Engineering.
Ragapriya, N., Kumar, T. A., Parthiban, R., Divya, P., Jay-
alakshmi, S., and Raman, D. R. (2023). Machine learn-
ing based house price prediction using modified extreme
boosting. AHAST, Asian Journal of Applied Science and
Technology.
Sahin, M. (2019). Optimization of dynamic ticket pricing
parameters. Journal of Revenue Pricing Management,
vol. 18, pp. 306-316.
Sahin, M. and Erol, R. (2017). A dynamic ticket pricing
approach for soccer games. Axioms, vol. 6.
Shadiqurrachman, S., Ridwan, A. Y., and Kusuma, A.
(2019). Online travel agency channel pricing policy
based on dynamic pricing model to maximize sales
profit using nonlinear integer programming approach. In
ICOEMIS, Proceedings of the 1st International Confer-
ence on Engineering and Management in Industrial Sys-
tem.
Shakya, S., Kern, M., Owusu, G., and Chin, C. M. (2012).
Neural network demand models and evolutionary opti-
misers for dynamic pricing. In 13th SGAI International
Conference on Innovative Techniques and Applications
of Artificial Intelligence.
Shehhi, M. A. and Karathanasopoulos, A. (2018). Forecast-
ing hotel prices in selected middle east and north africa
region (mena) cities with new forecasting tools. Theoret-
ical Economics Letters, vol. 8, pp. 1623-1638.
Shintani, M. and Umeno, K. (2022). General dynamic pric-
ing algorithms based on universal exponentialn booking
Dynamic Price Prediction for Revenue Management System in Hospitality Sector
227