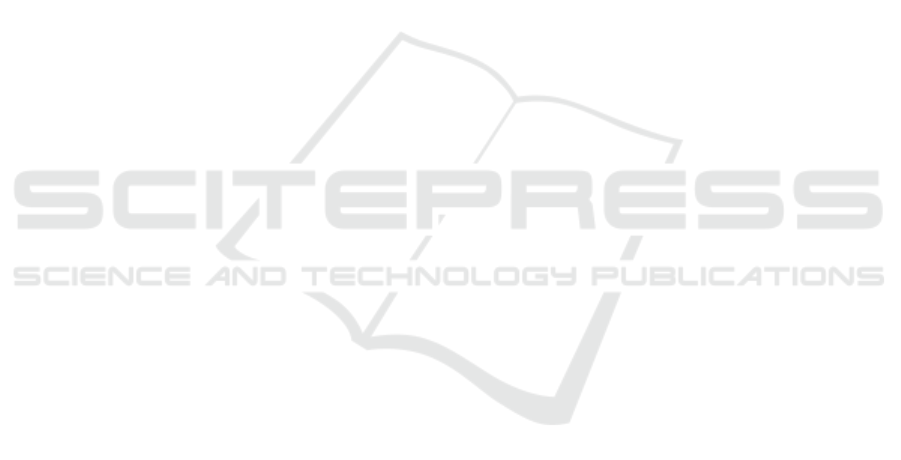
Therefore, our ontology alignment approach remains
a prospective when we use the maximum of the ontol-
ogy schema elements. In fact, we will consider other
ontology elements such as sub-classes, individuals, to
enhance our approach’s accuracy.
6 CONCLUSION AND
PERSPECTIVES
In this paper, we have proposed an ontology align-
ment approach based on several schema matching
techniques and machine learning models. We have
detailed the different phases and steps that compose
this alignment approach, namely the Pre-Processing
phase, the Ontology Element Extraction step, the
Similarity Value Calculation step, the Training and
Testing phase and the Quality Evaluation phase. The
pre-processing phase involves building similarity ma-
trices using individual matching tools executed on the
reference ontologies provided by the OAEI competi-
tion, in particular the Conference track and the Bench-
mark track. The training and testing phase consists of
determining the final aggregated alignment of a pair
of ontologies. The Quality Evaluation phase consists
of comparing the results obtained by our approach
with those of various OAEI participants, in order to
validate or invalidate the used machine learning mod-
els. We have validated our approach by performing
experimental results, which give better accuracy than
the approaches described in (Bulygin and Stupnikov,
2019; Huber et al., 2011; Bock et al., 2011; David,
2011; Xue and Huang, 2023; David, 2007; Eckert
et al., 2009; Straccia and Troncy, 2005; Euzenat et al.,
2005).
As future work, we propose to enrich the align-
ment approach by adding another set of ontology el-
ements, such as sub-classes and individuals. In ad-
dition, we will test other machine learning models
and select the best performing model for the ontology
alignment task.
REFERENCES
Bock, J., D
¨
anschel, C., and Stumpp, M. (2011). Mappso
and mapevo results for oaei 2011. Ontology Matching,
179(10.5555):2887541–2887559.
Bulygin, L. (2018). Combining lexical and semantic sim-
ilarity measures with machine learning approach for
ontology and schema matching problem. In Proceed-
ings of the XX International Conference “Data An-
alytics and Management in Data Intensive Domains
”(DAMDID/RCDL’2018), pages 245–249.
Bulygin, L. and Stupnikov, S. A. (2019). Applying of
machine learning techniques to combine string-based,
language-based and structure-based similarity mea-
sures for ontology matching. In DAMDID/RCDL,
pages 129–147.
David, J. (2007). Association rule ontology matching ap-
proach. International Journal on Semantic Web and
Information Systems (IJSWIS), 3(2):27–49.
David, J. (2011). Aroma results for oaei 2011. Ontology
Matching, 122.
Eckert, K., Meilicke, C., and Stuckenschmidt, H. (2009).
Improving ontology matching using meta-level learn-
ing. In The Semantic Web: Research and Applica-
tions: 6th European Semantic Web Conference, ESWC
2009 Heraklion, Crete, Greece, May 31–June 4, 2009
Proceedings 6, pages 158–172. Springer.
Euzenat, J., Gu
´
egan, P., and Valtchev, P. (2005). Ola in the
oaei 2005 alignment contest. In Proc. K-Cap 2005
workshop on Integrating ontology, pages 97–102. No
commercial editor.
Euzenat, J., Shvaiko, P., et al. (2007). Ontology matching,
volume 18. Springer.
Gruber, T. R. and Olsen, G. R. (1994). An ontology for
engineering mathematics. In Principles of Knowledge
Representation and Reasoning, pages 258–269. Else-
vier.
Huber, J., Sztyler, T., Noessner, J., and Meilicke, C.
(2011). Codi: Combinatorial optimization for data
integration–results for oaei 2011. Ontology Matching,
134.
Ond
ˇ
rej Zamazal, Jana Vata
ˇ
s
ˇ
cinov
´
a, L. Z. Initiative
d’
´
evaluation de l’alignement des ontologies - cam-
pagne oaei-2021.
Rahm, E. and Bernstein, P. A. (2001). A survey of ap-
proaches to automatic schema matching. the VLDB
Journal, 10(4):334–350.
Shvaiko, P. and Euzenat, J. (2005). A survey of schema-
based matching approaches. In Journal on data se-
mantics IV, pages 146–171. Springer.
Straccia, U. and Troncy, R. (2005). omap: Combining clas-
sifiers for aligning automatically owl ontologies. In
International Conference on Web Information Systems
Engineering, pages 133–147. Springer.
Xue, X. and Huang, Q. (2023). Generative adversarial
learning for optimizing ontology alignment. Expert
Systems, 40(4):e12936.
KEOD 2024 - 16th International Conference on Knowledge Engineering and Ontology Development
118