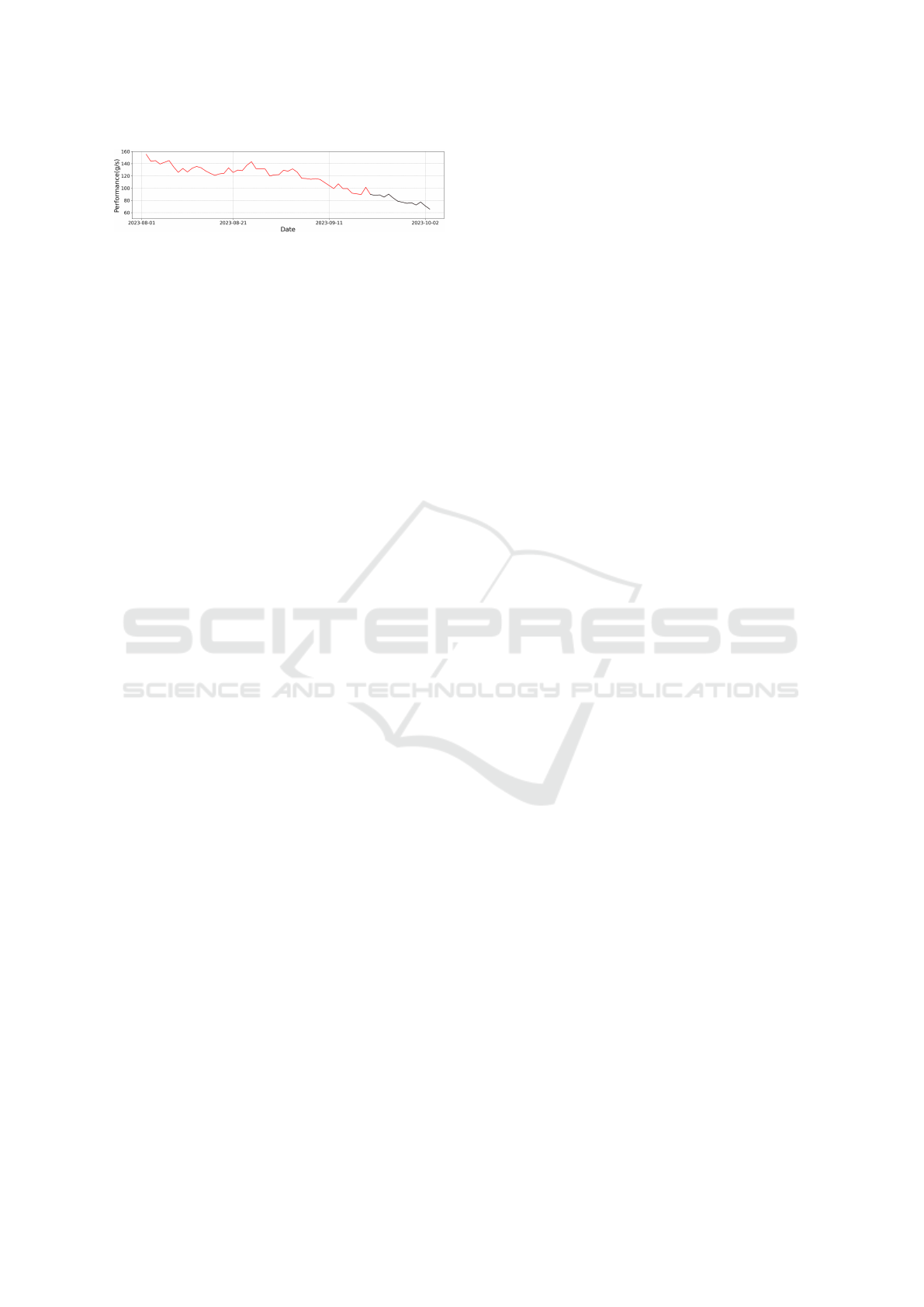
Figure 10: Model prediction graph start 01-08-2023 - pre-
diction time 17-09-2023.
calculations have determined the threshold for Pump
Maintenance Alert Level (PMAL) at 83.37. In light of
these findings, the estimated value of the impeller in
the FIP has been assessed at 80.02. This value, falling
below the established PMAL threshold, indicates the
necessity for maintenance intervention.
Consequently, our model’s predictions enable
maintenance and repair teams to plan effectively,
thereby increasing the efficiency and reliability of the
business. The identified situation is presented to the
relevant departments with reports containing visual
and statistical data from the trained model. This anal-
ysis process is conducted in accordance with a three-
day windowing logic. This method allows adaptation
to the dynamics of factory operations and enhances
the ability to respond quickly to changes in machine
performance.
6 CONCLUSIONS
In summary, we underscore the critical impact of
impeller wear in FIP on the textile dyeing process
and liquid chemical weighting systems through our
study. We emphasize the necessity for regular mon-
itoring and predictive maintenance of these pumps,
especially considering the wear patterns typically ob-
served within 4-6 months. Our use of the Facebook
Prophet model and time series data for early detection
of wear presents a proactive approach to maintaining
pump efficiency without installing new hardware and
high cost.
Our future research will involve applying the
Prophet model to time-series data we have already
collected from equipments in fabric dyeing machines
to develop predictive maintenance solutions.
ACKNOWLEDGEMENTS
This work is supported by The Scientific and Techno-
logical Research Council of Turkey (T
¨
UB
˙
ITAK) un-
der the project number №3200916.
REFERENCES
Almazrouei, S. M., Dweiri, F., Aydin, R., and Alnaqbi, A.
(2023). A review on the advancements and challenges
of artificial intelligence based models for predictive
maintenance of water injection pumps in the oil and
gas industry. SN Applied Sciences.
Chen, L., Wei, L., Wang, Y., Wang, J., and Li, W. (2022).
Monitoring and predictive maintenance of centrifugal
pumps based on smart sensors. Sensors, 22:2106.
Chhabria, S., Ghata, R., Mehta, V., Ghosekar, A., Araspure,
M., and Pakhide, N. (2022). Predictive maintenance
using machine learning on industrial water pumps. In-
ternational Journal of Innovations in Engineering and
Science, 7(9):76–81.
Daraz, A., Alabied, S., Gu, F., and Ball, A. D. (2019). Mod-
ulation signal bispectrum analysis of acoustic signals
for the impeller wear detection of centrifugal pumps.
In 2019 25th International Conference on Automation
and Computing (ICAC), pages 1–6.
Demirkiran, C. N.,
¨
Ust
¨
un, E., and Urhan, O. (2022).
Time series forecasting performance analysis of the
prophet method for predictive maintenance appli-
cations. ELECO 2022 Electrical-Electronics and
Biomedical Engineering Conference.
Emir
ˇ
Zuni
´
c, Kemal Korjeni
´
c, K. H., D. Ð. (2020). Ap-
plication of facebook’s probhet algorithm for success-
ful sales forecasting based on real-world data. Inter-
national Journal of Computer Science & Information
Technology (IJCSIT) Vol 12, No 2.
G
¨
org
¨
ul, A., C¸ om, M., and Sultano
˘
glu, S. (2023). Ker-
nel ridge regression based modelling and anomaly de-
tection for temperature control in textile dyeing pro-
cesses. In 27th International Conference on System
Theory, Control and Computing, pages 392–397.
Hooton, S. (2019). Flexible impeller pump
guide. https://www.northridgepumps.com/
article-141\_flexible-impeller-pump-guide.
Khoie, R., Gopaluni, B., Olson, J. A., and Stoeber, B.
(2015). A magnetic sensor to measure wear in cen-
trifugal pumps.
Qu, J., Miao, C., Hoseini, M., and Zuo, M. J. (2009).
Wear degree prognostics for slurry pumps using sup-
port vector machines. In 2009 8th International Con-
ference on Reliability, Maintainability and Safety.
Sanayha, M. and Vateekul, P. (2017). Fault detection for
circulating water pump using time series forecasting
and outlier detection.
Sarkar, A., Johnson, I., and Cohen, A. (2023). Jj piz-
zuto’s fabric science. https://books.google.com.
tr/books?id=5APXEAAAQBAJ.
Sugiyama, K., Nagasaka, H., Enomoto, T., and Hattori, S.
(2009). Prediction of wear depth distribution by slurry
on a pump impeller. International Journal of Fluid
Machinery and Systems, 2(1).
Taylor, S. J. and Letham, B. (2017). Forecasting at scale.
PeerJ Preprints.
Wang, C.-C. and Li, Y.-H. (2022). Machine-learning-based
system for the detection of entanglement in dyeing
and finishing processes. Sustainability 2022.
DATA 2024 - 13th International Conference on Data Science, Technology and Applications
252