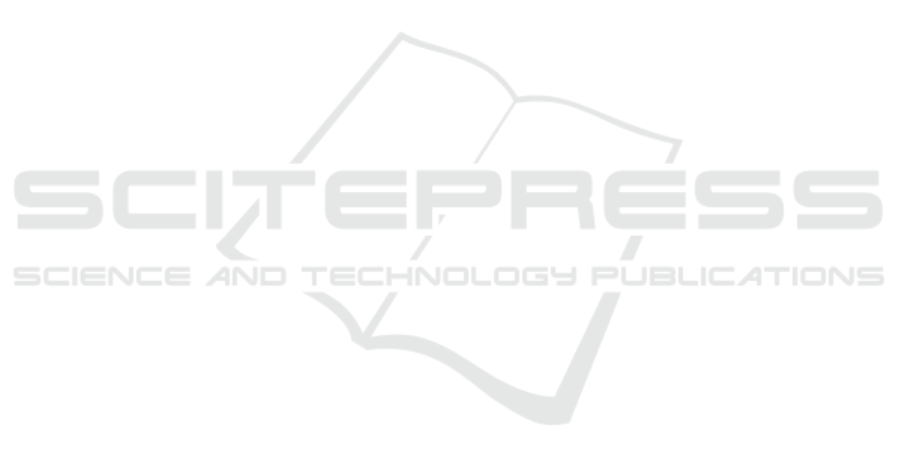
a balance between accurately portraying the system
and limiting the disclosure of information to potential
attackers becomes an important consideration that is
based on the individual company’s business.
Another limitation concerns the host-based intru-
sion detection systems (HIDS), in our experiments
OSSEC. It requires proper configuration as the default
configuration fails to provide alerts for newly created
files and the inability to recognize buffer overflows
generated in simulations as attacks. Custom rules can
be set up to configure these aspects, but it remains
unclear which other attack scenarios may not be de-
tected by OSSEC. However, this is based on the HIDS
used in the individual company, and other HIDS may
perform differently.
However, regardless of the HIDS, there is no as-
surance that the system is completely secure and that
attackers cannot exploit the honeypot; however, since
the honeypot is an isolated system, the impact of such
an incident is limited. By implementing robust se-
curity mechanisms and incorporating additional man-
ual controls (e.g., limited storage space and compu-
tational power), a high level of confidence in the sys-
tem’s security can be achieved. In addition, our exper-
iments have shown that the time span until an attacker
exploits the system is in the range of days. This means
that the risk of the honeypot being used as a dissem-
ination facility for illegal content is limited when its
monitored frequently.
7 SUMMARY AND CONCLUSION
Forensic readiness and its evaluation are important el-
ements for a holistic security architecture. The pre-
sented approach can be used to analyze forensic readi-
ness capabilities before an actual incident occurs or
even before an attack simulation is conducted. It is
based on cloning an existing system, sanitizing it to
transform it into a honeypot, and deploying it in a
cloud environment. The experiments carried out have
shown that this approach is feasible, reasonably se-
cure, and generates actual data for forensic investi-
gations as attacks on the honeypots are conducted.
Therefore, we consider our approach an important
step in enabling a forensic readiness assessment using
existing company systems and processes, especially
for small and medium enterprises.
REFERENCES
Barham, P., Dragovic, B., Fraser, K., Hand, S., Harris, T.,
Ho, A., Neugebauer, R., Pratt, I., and Warfield, A.
(2003). Xen and the art of virtualization. In Proceed-
ings of the nineteenth ACM symposium on Operating
systems principles, pages 164–177. ACM.
Biedermann, S., Mink, M., and Katzenbeisser, S. (2012).
Fast dynamic extracted honeypots in cloud comput-
ing. In Proceedings of the 2012 ACM Workshop on
Cloud computing security workshop, pages 13–18.
ACM.
Dodson, M., Beresford, A. R., and Vingaard, M. (2020).
Using global honeypot networks to detect targeted ics
attacks. In 2020 12th International Conference on Cy-
ber Conflict (CyCon), volume 1300, pages 275–291.
Kebande, V. R., Karie, N. M., and Venter, H. S. (2016).
A generic digital forensic readiness model for BYOD
using honeypot technology. In 2016 IST-Africa Week
Conference, pages 1–12.
Kelly, C., Pitropakis, N., Mylonas, A., McKeown, S., and
Buchanan, W. J. (2021). A comparative analysis
of honeypots on different cloud platforms. Sensors,
21(7).
Mansfield-Devine, S. (2018). The best form of defence–the
benefits of red teaming. Computer Fraud & Security,
2018(10):8–12.
Oakley, J. G. (2019). Professional Red Teaming: Conduct-
ing Successful Cybersecurity Engagements. Apress.
Priya, V. D. and Chakkaravarthy, S. S. (2023). Container-
ized cloud-based honeypot deception for tracking at-
tackers. Scientific Reports, 13(1):1437.
Setianto, F., Tsani, E., Sadiq, F., Domalis, G., Tsakalidis,
D., and Kostakos, P. (2022). Gpt-2c: A parser for hon-
eypot logs using large pre-trained language models.
In Proceedings of the 2021 IEEE/ACM International
Conference on Advances in Social Networks Analysis
and Mining, page 649–653, New York, NY, USA. As-
sociation for Computing Machinery.
Singh, K. D. (2021). Securing of cloud infrastructure using
enterprise honeypot. In 2021 3rd Intl. Conference on
Advances in Computing, Communication Control and
Networking (ICAC3N), pages 1388–1393.
Taiwo, A. and Claims, I. (2022). An extended digital foren-
sic readiness and maturity model. Forensic Science
International: Digital Investigation, 40:301348.
Tan, J. (2001). Forensic readiness. Cambridge, MA:@
Stake, 1. Publisher: Citeseer.
Verma, A. S. and Dubey, A. (2020). A review on honey-
pot deployment. LJP London Journal of Research in
Computer Science and Technology, 20(1).
Washofsky, A. D. (2021). Deploying and analyzing con-
tainerized honeypots in the cloud with T-Pot. PhD the-
sis, Monterey, CA; Naval Postgraduate School.
Evaluating Digital Forensic Readiness: A Honeypot Approach
621