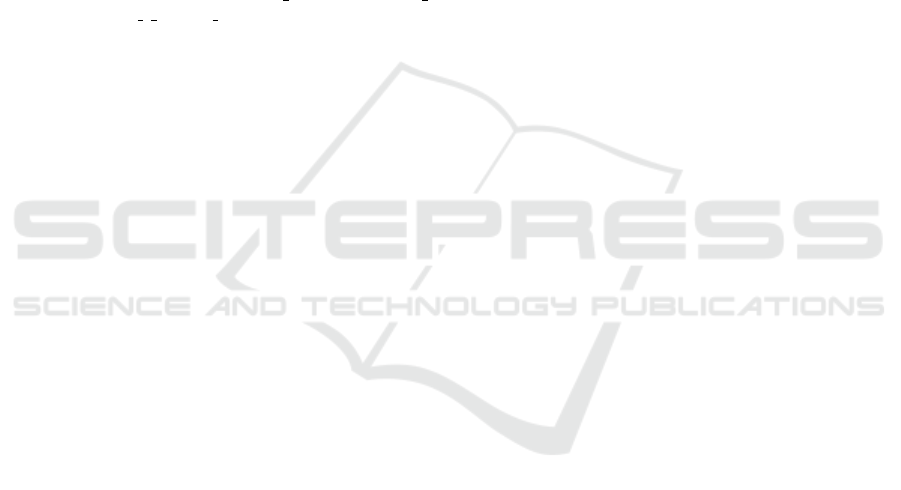
REFERENCES
Brownlee, A., Wallace, A., and Cairns, D. (2021). Min-
ing markov network surrogates to explain the results of
metaheuristic optimisation. In SICSA eXplainable Artif-
ical Intelligence Workshop 2021, pages 64–70. CEUR
Workshop Proceedings.
Deb, K., Bandaru, S., Greiner, D., Gaspar-Cunha, A., and
Tutum, C. C. (2014). An integrated approach to auto-
mated innovization for discovering useful design prin-
ciples: Case studies from engineering. Applied Soft
Computing, 15:42–56.
Do
ˇ
silovi
´
c, F. K., Br
ˇ
ci
´
c, M., and Hlupi
´
c, N. (2018). Ex-
plainable artificial intelligence: A survey. In 2018 41st
International convention on information and commu-
nication technology, electronics and microelectronics
(MIPRO), pages 0210–0215. IEEE.
European Space Agency (2022). ESA - cosmic vi-
sion. https://www.esa.int/Science Exploration/Space
Science/ESA s Cosmic Vision.
Fyvie, M., McCall, J. A., and Christie, L. A. (2021). To-
wards explainable metaheuristics: Pca for trajectory
mining in evolutionary algorithms. In International
Conference on Innovative Techniques and Applications
of Artificial Intelligence, pages 89–102. Springer.
Grießbach, D., Witteck, U., and Paproth, C. (2021). The fine
guidance system of the PLATO mission. In Cugny, B.,
Sodnik, Z., and Karafolas, N., editors, International
Conference on Space Optics — ICSO 2020, volume
11852, page 118523H. International Society for Optics
and Photonics, SPIE.
Harris, C. R., Millman, K. J., van der Walt, S. J., Gommers,
R., Virtanen, P., Cournapeau, D., Wieser, E., Taylor,
J., Berg, S., Smith, N. J., Kern, R., Picus, M., Hoyer,
S., van Kerkwijk, M. H., Brett, M., Haldane, A., del
R
´
ıo, J. F., Wiebe, M., Peterson, P., G
´
erard-Marchant,
P., Sheppard, K., Reddy, T., Weckesser, W., Abbasi,
H., Gohlke, C., and Oliphant, T. E. (2020). Array
programming with NumPy. Nature, 585(7825):357–
362.
Holzinger, A. (2021). The next frontier: Ai we can re-
ally trust. In Joint European conference on machine
learning and knowledge discovery in databases, pages
427–440. Springer.
Islam, M. R., Ahmed, M. U., Barua, S., and Begum, S.
(2022). A systematic review of explainable artificial
intelligence in terms of different application domains
and tasks. Applied Sciences, 12(3):1353.
Lipton, Z. C. (2018). The mythos of model interpretability:
In machine learning, the concept of interpretability is
both important and slippery. Queue, 16(3):31–57.
Martin Pertenais, P. P. T. (2022). Instrument technical re-
quirement document. PLATO-DLR-PL-RS-0001.
Mirjalili, S., Lewis, A., and Mostaghim, S. (2015). Con-
fidence measure: a novel metric for robust meta-
heuristic optimisation algorithms. Information Sci-
ences, 317:114–142.
Mouret, J.-B. and Clune, J. (2015). Illuminating search
spaces by mapping elites. arXiv e-prints, pages arXiv–
1504.
Pedregosa, F., Varoquaux, G., Gramfort, A., Michel, V.,
Thirion, B., Grisel, O., Blondel, M., Prettenhofer, P.,
Weiss, R., Dubourg, V., Vanderplas, J., Passos, A.,
Cournapeau, D., Brucher, M., Perrot, M., and Duch-
esnay, E. (2011). Scikit-learn: Machine learning
in Python. Journal of Machine Learning Research,
12:2825–2830.
Plotly Technologies Inc. (2015). Collaborative data science.
https://plot.ly.
Reris, R. and Brooks, J. P. (2015). Principal component
analysis and optimization: A tutorial. 14th INFORMS
Computing Society Conference, pages 212–225.
Shlens, J. (2014). A tutorial on principal component analysis.
arXiv preprint arXiv:1404.1100.
Stambke, J. (2023). Principal component analysis for ex-
plainability of genetic test generation in satellite image
processing.
The HDF Group (2006). Hierarchical Data Format, version
5. https://github.com/HDFGroup/hdf5.
The pandas development team (2022). pandas-dev/pandas:
Pandas. https://doi.org/10.5281/zenodo.4309786.
Urquhart, N., Guckert, M., and Powers, S. (2019). Increasing
trust in meta-heuristics by using map-elites. In Pro-
ceedings of the Genetic and Evolutionary Computation
Conference Companion, pages 1345–1348.
Urquhart, N., H
¨
ohl, S., and Hart, E. (2021). Automated,
explainable rule extraction from map-elites archives.
In International Conference on the Applications of
Evolutionary Computation (Part of EvoStar), pages
258–272. Springer.
Wallace, A., Brownlee, A. E., and Cairns, D. (2021). To-
wards explaining metaheuristic solution quality by data
mining surrogate fitness models for importance of vari-
ables. In International Conference on Innovative Tech-
niques and Applications of Artificial Intelligence, pages
58–72. Springer.
Witteck, U., Grießbach, D., and Herber, P. (2019). Equiva-
lence class definition for automated testing of satellite
on-board image processing. In International Confer-
ence on Software Technologies, pages 3–25. Springer.
Witteck, U., Grießbach, D., and Herber, P. (2020). A genetic
algorithm with tournament selection for automated
testing of satellite on-board image processing. In Inter-
national Conference on Software Technologies, pages
134–157. Springer.
Wu, W., Massart, D., and de Jong, S. (1997). The kernel
pca algorithms for wide data. part i: Theory and al-
gorithms. Chemometrics and Intelligent Laboratory
Systems, 36(2):165–172.
Xu, F., Uszkoreit, H., Du, Y., Fan, W., Zhao, D., and Zhu,
J. (2019). Explainable ai: A brief survey on history,
research areas, approaches and challenges. In CCF in-
ternational conference on natural language processing
and Chinese computing, pages 563–574. Springer.
ICSOFT 2024 - 19th International Conference on Software Technologies
218