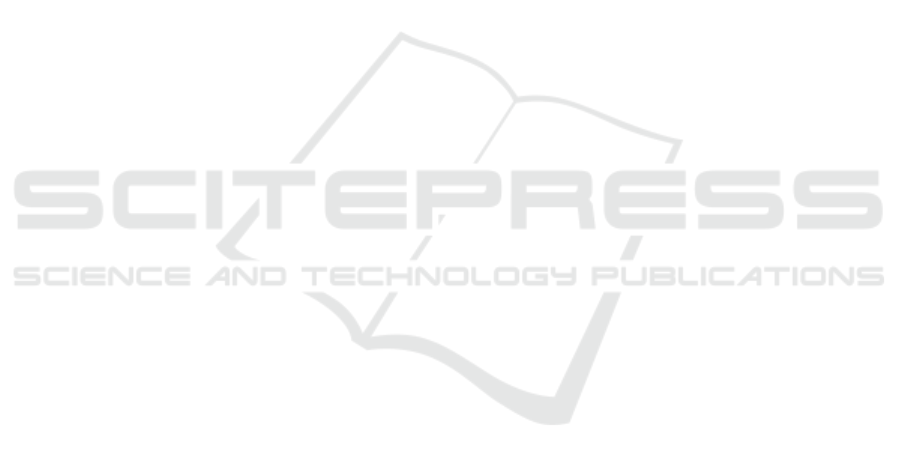
Feng, J. (2023). Designing an artificial intelligence-based
sport management system using big data. Soft Com-
puting, 27(21):16331–16352.
Fister, I., Fister, D., Deb, S., Mlakar, U., Brest, J., and Fis-
ter Jr., I. (2020). Post hoc analysis of sport perfor-
mance with differential evolution. Neural Computing
and Applications, 32(15):10799–10808.
Frevel, N., Schmidt, S. L., Beiderbeck, D., Penkert, B.,
and Subirana, B. (2020). Taxonomy of sportstech.
21st Century Sports: How Technologies Will Change
Sports in the Digital Age, pages 15–37.
Goldberger, A. L., Amaral, L. A., Glass, L., Hausdorff,
J. M., Ivanov, P. C., Mark, R. G., Mietus, J. E., Moody,
G. B., Peng, C.-K., and Stanley, H. E. (2000). Phys-
iobank, physiotoolkit, and physionet: components of
a new research resource for complex physiologic sig-
nals. circulation, 101(23):e215–e220.
Herberger, T. A. and Litke, C. (2021). The impact of big
data and sports analytics on professional football: A
systematic literature review. Digitalization, digital
transformation and sustainability in the global econ-
omy: risks and opportunities, pages 147–171.
Karim, M. R., Beyan, O., Zappa, A., Costa, I. G., Rebholz-
Schuhmann, D., Cochez, M., and Decker, S. (2021).
Deep learning-based clustering approaches for bioin-
formatics. Briefings in bioinformatics, 22(1):393–
415.
Kirchner, K., Zec, J., and Deliba
ˇ
si
´
c, B. (2016). Facili-
tating data preprocessing by a generic framework: a
proposal for clustering. Artificial Intelligence Review,
45(3):271–297.
Li, G. (2022). Construction of Sports Training Performance
Prediction Model Based on a Generative Adversarial
Deep Neural Network Algorithm. Computational In-
telligence and Neuroscience, 2022.
Li, X., Chen, X., Guo, L., and Rochester, C. A. (2022). Ap-
plication of Big Data Analysis Techniques in Sports
Training and Physical Fitness Analysis. Hindawi
Wireless Communications and Mobile Computing Vol-
ume 2022, Article ID 3741087.
Meyer, V., Al-Ghezi, A., and Wiese, L. (2023). Exploiting
clustering for sports data analysis: A study of public
and real-world datasets. In International Workshop on
Machine Learning and Data Mining for Sports Ana-
lytics, pages 191–201. Springer.
Narizuka, T. and Yamazaki, Y. (2019). Clustering algorithm
for formations in football games. Scientific Reports,
9(1):1–8.
Rend
´
on, E., Abundez, I. M., Gutierrez, C., Zagal, S. D.,
Arizmendi, A., Quiroz, E. M., and Arzate, H. E.
(2011). A comparison of internal and external cluster
validation indexes. In Proceedings of the 2011 Ameri-
can Conference, San Francisco, CA, USA, volume 29,
pages 1–10.
Rossi, A., Da Pozzo, E., Menicagli, D., Tremolanti, C.,
Priami, C., Sirbu, A., Clifton, D., Martini, C., and
Morelli, D. (2020a). Multilevel monitoring of activ-
ity and sleep in healthy people. PhysioNet.
Rossi, A., Da Pozzo, E., Menicagli, D., Tremolanti, C.,
Priami, C., S
ˆ
ırbu, A., Clifton, D. A., Martini, C.,
and Morelli, D. (2020b). A public dataset of 24-h
multi-levels psycho-physiological responses in young
healthy adults. Data, 5(4):91.
Sarlis, V., Papageorgiou, G., and Tjortjis, C. (2023). Sports
analytics and text mining nba data to assess recovery
from injuries and their economic impact. Computers,
12(12):261.
Schmidt, S. L. (2020). 21st Century Sports. Springer.
Shelly, Z., Burch, R. F., Tian, W., Strawderman, L., Piroli,
A., and Bichey, C. (2020). Using K-means Clustering
to Create Training Groups for Elite American Foot-
ball Student-athletes Based on Game Demands. Inter-
national Journal of Kinesiology and Sports Science,
8(2):47–63.
Thornton, H. R., Delaney, J. A., Duthie, G. M., and Das-
combe, B. J. (2019). Developing athlete monitoring
systems in team sports: Data analysis and visualiza-
tion. International journal of sports physiology and
performance, 14(6):698–705.
Xu, R. and Wunsch, D. (2005). Survey of Clustering Al-
gorithms. IEEE Transactions on Neural Networks,
16(3):645–678.
ICEIS 2024 - 26th International Conference on Enterprise Information Systems
160