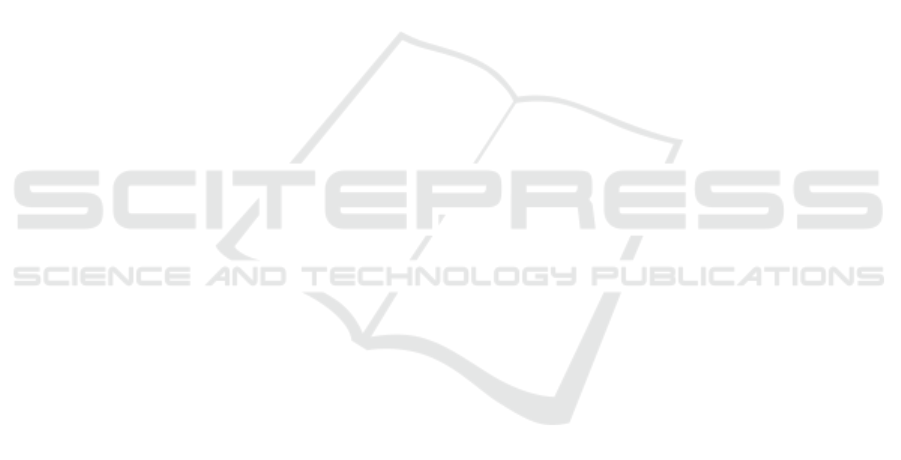
Hou, F. and Jansen, S. (2023). A systematic literature re-
view on trust in the software ecosystem. Empirical
Software Engineering, 28(1):8.
Illi, E., Qaraqe, M., Althunibat, S., Alhasanat, A., Alsafas-
feh, M., de Ree, M., Mantas, G., Rodriguez, J., Aman,
W., and Al-Kuwari, S. (2023). Physical layer security
for authentication, confidentiality, and malicious node
detection: a paradigm shift in securing iot networks.
IEEE Communications Surveys & Tutorials.
Iqbal, D. and Buhnova, B. (2022). Model-based approach
for building trust in autonomous drones through digi-
tal twins. In 2022 IEEE International Conference on
Systems, Man, and Cybernetics (SMC), pages 656–
662. IEEE.
Jafarian, B., Yazdani, N., and Haghighi, M. S. (2020).
Discrimination-aware trust management for social in-
ternet of things. Computer Networks, 178:107254.
Jøsang, A., Ismail, R., and Boyd, C. (2007). A survey of
trust and reputation systems for online service provi-
sion. Decision support systems, 43(2):618–644.
Kamvar, S. D., Schlosser, M. T., and Garcia-Molina, H.
(2003). The eigentrust algorithm for reputation man-
agement in p2p networks. In Proceedings of the 12th
international conference on World Wide Web, pages
640–651.
Karthik, N. and Ananthanarayana, V. (2017). A hybrid trust
management scheme for wireless sensor networks.
Wireless Personal Communications, 97:5137–5170.
Katina, P. F. and Keating, C. B. (2018). Cyber-physical sys-
tems governance: a framework for (meta) cybersecu-
rity design. Security by Design: Innovative Perspec-
tives on Complex Problems, pages 137–169.
Katina, P. F., Keating, C. B., Gheorghe, A. V., and Masera,
M. (2017). Complex system governance for critical
cyber-physical systems. International Journal of Crit-
ical Infrastructures, 13(2-3):168–183.
Khan, M. S., Midi, D., Khan, M. I., and Bertino, E. (2015).
Adaptive trust update frequency in manets. In 2015
IEEE 21st International Conference on Parallel and
Distributed Systems (ICPADS), pages 132–139. IEEE.
Khan, W. Z., Hakak, S., Khan, M. K., et al. (2020). Trust
management in social internet of things: Architec-
tures, recent advancements, and future challenges.
IEEE Internet of Things Journal, 8(10):7768–7788.
Khatun, M. A., Chowdhury, N., and Uddin, M. N. (2019).
Malicious nodes detection based on artificial neural
network in iot environments. In 2019 22nd Inter-
national Conference on Computer and Information
Technology (ICCIT), pages 1–6. IEEE.
K
¨
okciyan, N. and Yolum, P. (2020). Turp: Managing trust
for regulating privacy in internet of things. IEEE In-
ternet Computing, 24(6):9–16.
Li, B., Ye, R., Gu, G., Liang, R., Liu, W., and Cai, K.
(2020). A detection mechanism on malicious nodes
in iot. Computer Communications, 151:51–59.
Liu, L., Ma, Z., and Meng, W. (2019). Detec-
tion of multiple-mix-attack malicious nodes using
perceptron-based trust in iot networks. Future gen-
eration computer systems, 101:865–879.
Liu, Z., Yang, D.-s., Wen, D., Zhang, W.-m., and Mao, W.
(2011). Cyber-physical-social systems for command
and control. IEEE Intelligent Systems, 26(4):92–96.
Lochner, M. and Smilek, D. (2023). The uncertain advi-
sor: trust, accuracy, and self-correction in an auto-
mated decision support system. Cognitive Processing,
24(1):95–106.
Magdich, R., Jemal, H., Nakti, C., and Ayed, M. B.
(2021). An efficient trust related attack detection
model based on machine learning for social internet
of things. In 2021 International Wireless Communica-
tions and Mobile Computing (IWCMC), pages 1465–
1470. IEEE.
Mahmood, A., Butler, B., Zhang, W. E., Sheng, Q. Z.,
and Siddiqui, S. A. (2019). A hybrid trust manage-
ment heuristic for vanets. In 2019 IEEE International
Conference on Pervasive Computing and Communi-
cations Workshops (PerCom Workshops), pages 748–
752. IEEE.
Malik, S., Dedeoglu, V., Kanhere, S. S., and Jurdak, R.
(2019). Trustchain: Trust management in blockchain
and iot supported supply chains. In 2019 IEEE In-
ternational Conference on Blockchain (Blockchain),
pages 184–193. IEEE.
Marche, C. and Nitti, M. (2020). Trust-related attacks and
their detection: A trust management model for the so-
cial iot. IEEE Transactions on Network and Service
Management, 18(3):3297–3308.
Masmoudi, M., Abdelghani, W., Amous, I., and S
`
edes, F.
(2020). Deep learning for trust-related attacks de-
tection in social internet of things. In Advances in
E-Business Engineering for Ubiquitous Computing:
Proceedings of the 16th International Conference on
e-Business Engineering (ICEBE 2019), pages 389–
404. Springer.
Mechanic, D. (1996). The logic and limits of trust. Con-
temporary Sociology, 25(4):455.
Meena Kowshalya, A. and Valarmathi, M. (2017). Trust
management for reliable decision making among so-
cial objects in the social internet of things. IET Net-
works, 6(4):75–80.
Mehdizadeh, N. and Farzaneh, N. (2022). An evidence
theory based approach in detecting malicious con-
troller in the multi-controller software-defined inter-
net of things network. Adhoc & Sensor Wireless Net-
works, 51(4).
Mendoza, C. V. and Kleinschmidt, J. H. (2015). Mit-
igating on-off attacks in the internet of things us-
ing a distributed trust management scheme. In-
ternational Journal of Distributed Sensor Networks,
11(11):859731.
Muzammal, S. M., Murugesan, R. K., and Jhanjhi, N. Z.
(2020). A comprehensive review on secure routing
in internet of things: Mitigation methods and trust-
based approaches. IEEE Internet of Things Journal,
8(6):4186–4210.
Namal, S., Gamaarachchi, H., MyoungLee, G., and Um,
T.-W. (2015). Autonomic trust management in cloud-
based and highly dynamic iot applications. In 2015
ITU Kaleidoscope: Trust in the Information Society
(K-2015), pages 1–8. IEEE.
Taxonomy of Governance Mechanisms for Trust Management In Smart Dynamic Ecosystems
709