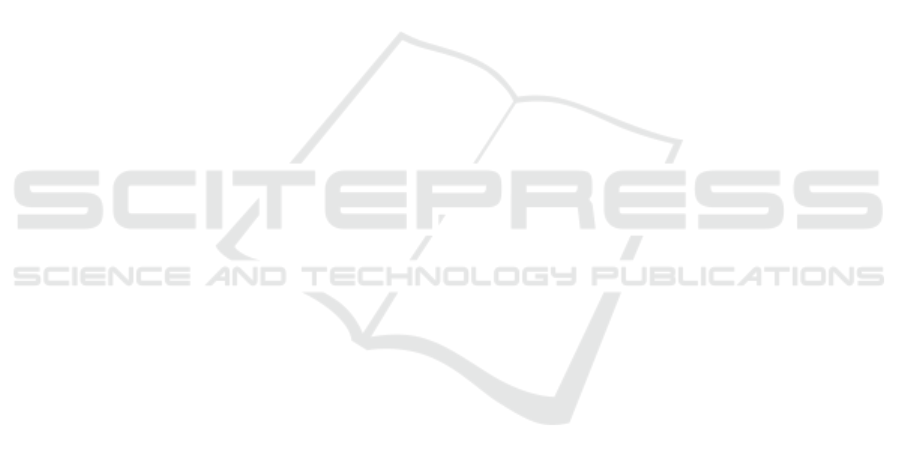
road users seek communication from others when
facing uncertainty (Portouli et al., 2014). Our results
corroborate these findings.
Finally, communication in both rulesets gave
statistically significant payoff improvements to both
Vehicle (the issuer) and Vehicle (the recipient).
Thus, cooperative communication can emerge from
selfish motives. These improvements were also
statistically significant with implicit communication
over control, meaning that active participation by
Vehicle was not necessary for Vehicle to make
better decisions based on implicitly communicated
information. This is an important finding considering
autonomous vehicles, which may be able to make use
of freely advertised information such as acceleration
to better understand human drivers’ intent.
6 CONCLUSIONS
We have conducted a series of experiments which
demonstrate that non-cooperative game theory is a
viable framework to study, model and characterise
the exchange of implicit and explicit communication
between interacting road users. More importantly, we
show that it is possible to produce meaningfully safer
interactions and fewer non-ideal outcomes without
the need to assume common goals a priori. By
foregoing this assumption, non-cooperative game
theory provides a more robust framework for
modelling communication descriptively and
prescriptively in a variety of situations and scenarios.
Therefore, in a strict game-theoretic sense, effective
communication is a viable non-cooperative strategy
which can benefit both the sender and the recipient.
REFERENCES
AASHTO. (2011). A policy on geometric design of
highways and streets, 6th edition. Washington, D.C.:
American Association of State Highway and
Transportation Officials
Ali, Y., Zheng, Z., Haque, M. M., & Wang, M. (2019). A
game theory-based approach for modelling mandatory
lane-changing behaviour in a connected environment.
Transportation Research Part C: Emerging
Technologies, 106, 220-242.
Axelrod, R., & Hamilton, W. D. (1981). The evolution of
cooperation. Science, 211(4489), 1390.
Bitar, I., Watling, D., & Romano, R. (2022). How can
autonomous road vehicles coexist with human-driven
vehicles? An evolutionary-game-theoretic perspective.
In Proceedings of the 8th International Conference on
Vehicle Technology and Intelligent Transport Systems
- VEHITS,
Bitar, I., Watling, D., & Romano, R. (2023). Sensitivity
analysis of the spatial parameters in modelling the
evolutionary interaction between autonomous vehicles
and other road users. SN Computer Science, 4(4), 336.
Bokare, P. S., & Maurya, A. K. (2017). Acceleration-
deceleration behaviour of various vehicle types.
Transportation Research Procedia, 25, 4733-4749.
Camara, F., Dickinson, P., Merat, N., & Fox, C. W. (2019).
Towards game theoretic av controllers: Measuring
pedestrian behaviour in virtual reality. In Proceedings
of TCV2019: Towards Cognitive Vehicles,
DfT. (2022). Mobile phone use by drivers: Great britain,
2021 (Seatbelt and mobile phone use surveys: 2021,
Issue. D. f. Transport.
Elvik, R. (2014). A review of game-theoretic models of
road user behaviour. Accident Analysis & Prevention,
62, 388-396.
Fernández Domingos, E., Loureiro, M., Álvarez-López, T.,
Burguillo, J., Covelo, J., Peleteiro, A., & Byrski, A.
(2017). Emerging cooperation in n-person iterated
prisoner's dilemma over dynamic complex networks.
Computing and Informatics, 36, 493-516.
Finnegan, P., & Green, P. (1990). Time to change lanes: A
literature review.
Harsanyi, J. C. (1968). Games with incomplete information
played by "bayesian" players, i-iii. Part iii. The basic
probability distribution of the game. Management
Science, 14(7), 486-502.
Ji, A., & Levinson, D. (2020). A review of game theory
models of lane changing. Transportmetrica A:
Transport Science, 16(3), 1628-1647.
Jin, P. J., Yang, D., Ran, B., Cebelak, M., & Walton, C. M.
(2013). Bidirectional control characteristics of general
motors and optimal velocity car-following models:
Implications for coordinated driving in a connected
vehicle environment. Transportation Research Record,
2381(1), 110-119.
Joyce, J. (2021). Bayes’ theorem. Metaphysics Research
Lab, Stanford University. Retrieved 2024-02-22 from
https://plato.stanford.edu/archives/fall2021/entries/bay
es-theorem/
Kita, H. (1999). A merging–giveway interaction model of
cars in a merging section: A game theoretic analysis.
Transportation Research Part A: Policy and Practice,
33(3), 305-312.
Orzan, N., Acar, E., Grossi, D., & Radulescu, R. (2023,
2023/5). Emergent cooperation and deception in public
good games. In 2023 Adaptive and Learning Agents
Workshop at AAMAS,
Osborne, M. J. (2003). An introduction to game theory.
Oxford University Press.
Portouli, E., Nathanael, D., & Marmaras, N. (2014).
Drivers' communicative interactions: On-road
observations and modelling for integration in future
automation systems. Ergonomics, 57(12), 1795-1805.
Rubenstein, D. R., & Kealey, J. (2010). Cooperation,
conflict, and the evolution of complex animal societies.
Nature Education Knowledge, 3(10), 78.
Modelling Implicit and Explicit Communication Between Road Users from a Non-Cooperative Game-Theoretic Perspective: An
Exploratory Study
463