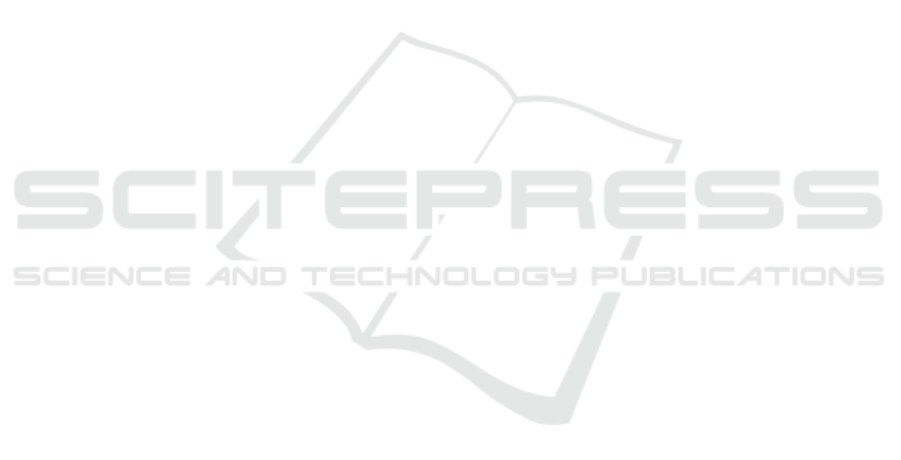
ACKNOWLEDGEMENTS
This work was partially funded by the Department of
Energy through the Office of Energy Efficiency and
Renewable Energy (EERE), Vehicle Technologies
Office, Energy Efficient Mobility Systems Program
under award number DE-EE0008209. M. Elouni ac-
knowledges the receipt of the Chenery Grant from
Randolph-Macon College. M. Menendez acknowl-
edges the support of the NYUAD Center for Interact-
ing Urban Networks (CITIES), funded by Tamkeen
under the NYUAD Research Institute Award CG001.
REFERENCES
Abdelghaffar, H. M. and Rakha, H. A. (2019). A novel de-
centralized game-theoretic adaptive traffic signal con-
troller: Large-scale testing. Sensors, 19(10).
Aik, L. E., Choon, T. W., and Abu, M. S. (2023). K-means
algorithm based on flower pollination algorithm and
calinski-harabasz index. In Journal of Physics: Con-
ference Series, volume 2643, page 012019. IOP Pub-
lishing.
Bac¸
˜
ao, F., Lobo, V., and Painho, M. (2004). Geo-self-
organizing map (geo-som) for building and explor-
ing homogeneous regions. In International Confer-
ence on Geographic Information Science, pages 22–
37. Springer.
Bac¸
˜
ao, F., Lobo, V., and Painho, M. (2005). Geo-som and
its integration with geographic information systems.
In Proc. Workshop on Self-Organizing Maps, Paris,
France.
Bichiou, Y., Elouni, M., Abdelghaffar, H. M., and Rakha,
H. A. (2020). Sliding mode network perimeter con-
trol. IEEE Transactions on Intelligent Transportation
Systems, 22(5):2933–2942.
Elouni, M., Abdelghaffar, H. M., and Rakha, H. A. (2021).
Adaptive traffic signal control: Game-theoretic decen-
tralized vs. centralized perimeter control. Sensors,
21(1):274.
Feng, Z., Xu, C., Zuo, Y., Luo, X., Wang, L., Chen, H.,
Xie, X., Yan, D., and Liang, T. (2023). Analysis of
water quality indexes and their relationships with veg-
etation using self-organizing map and geographically
and temporally weighted regression. Environmental
Research, 216:114587.
Hamel, L. and Brown, C. W. (2011). Improved inter-
pretability of the unified distance matrix with con-
nected components. In Proceedings of the Interna-
tional Conference on Data Science (ICDATA), page 1.
The Steering Committee of The World Congress in
Computer Science, Computer Engineering and Ap-
plied Computing (WorldComp).
Henriques, R., Bacao, F., and Lobo, V. (2012). Exploratory
geospatial data analysis using the geosom suite. Com-
puters, Environment and Urban Systems, 36(3):218–
232.
Kim, J.-Y., Gim, U. S., and Oh, M. T. (2014). Characteris-
tic analysis and classification of rural areas: Based on
the eup and myon areas of chungcheongnam-do. The
Korean Regional Development Association, 26(1):27–
44.
Kim, T.-S., Dhakal, T., Kim, S.-H., Lee, J.-H., Kim, S.-
J., and Jang, G.-S. (2023). Examining village char-
acteristics for forest management using self-and geo-
graphic self-organizing maps: A case from the baek-
dudaegan mountain range network in korea. Ecologi-
cal Indicators, 148:110070.
Kohonen, T. (2013). Essentials of the self-organizing map.
Neural networks, 37:52–65.
Le Thi, H. A. and Nguyen, M. C. (2014). Self-organizing
maps by difference of convex functions optimiza-
tion. Data Mining and Knowledge Discovery, 28(5-
6):1336–1365.
Lin, X. and Xu, J. (2020). Road network partition-
ing method based on canopy-kmeans clustering algo-
rithm. Archives of Transport, 54(2):95–106.
Lopez, C., Krishnakumari, P., Leclercq, L., Chiabaut, N.,
and van Lint, H. (2017). Spatiotemporal partition-
ing of transportation network using travel time data.
Transportation Research Record, 2623(1):98–107.
Lukas Amb
¨
uhl, Monica Menendez, M. C. G. (2023).
Understanding congestion propagation by combining
percolation theory with the macroscopic fundamental
diagram. Communications Physics, 6(26).
Melin, P., Monica, J. C., Sanchez, D., and Castillo, O.
(2020). Analysis of spatial spread relationships of
coronavirus (covid-19) pandemic in the world using
self organizing maps. Chaos, Solitons & Fractals,
138:109917.
Pires, F. J., Lobo, V., and Bac¸
˜
ao, F. (2007). Insights on
the interpretation of som and u-matrices with an ex-
ample clustering based in oceanographic data. In 10th
AGILE international conference on geographic infor-
mation science, Aalborg University, Denmark.
Provenzano, D. and Giambrone, R. (2023). Clustering of
tourism patterns with self-organizing maps: The case
of sicily. Tourism Analysis.
Ran, X., Zhou, X., Lei, M., Tepsan, W., and Deng, W.
(2021). A novel k-means clustering algorithm with a
noise algorithm for capturing urban hotspots. Applied
Sciences, 11(23):11202.
Saeedmanesh, M. and Geroliminis, N. (2016). Clustering of
heterogeneous networks with directional flows based
on “snake” similarities. Transportation Research Part
B: Methodological, 91:250–269.
Sahu, R. T., Verma, M. K., and Ahmad, I. (2023). Density-
based spatial clustering of application with noise ap-
proach for regionalisation and its effect on hierarchi-
cal clustering. International Journal of Hydrology Sci-
ence and Technology, 16(3):240–269.
Waters, N. (2017). Tobler’s first law of geography. The
international encyclopedia of geography, pages 1–13.
Wulandari, V., Syarif, Y., Alfian, Z., Althof, M. A., and
Mufidah, M. (2024). Comparison of density-based
spatial clustering of applications with noise (dbscan),
k-means and x-means algorithms on shopping trends
data. IJATIS: Indonesian Journal of Applied Technol-
ogy and Innovation Science, 1(1):1–8.
VEHITS 2024 - 10th International Conference on Vehicle Technology and Intelligent Transport Systems
472