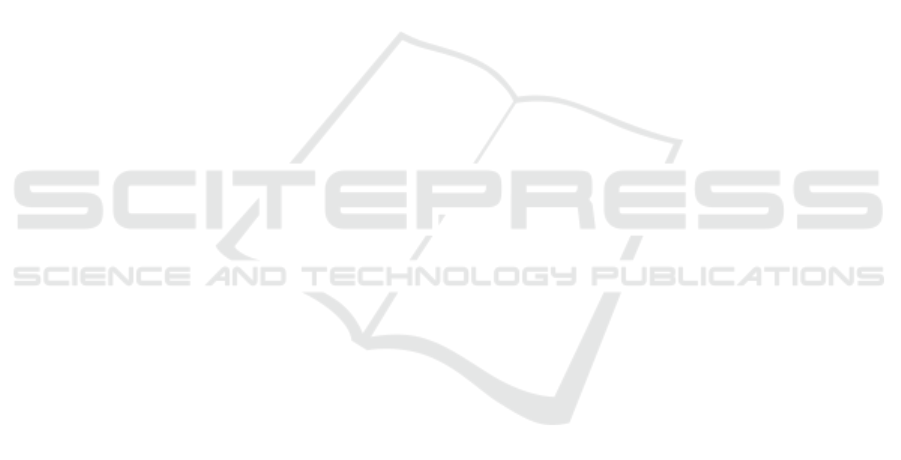
15-17, 2023, Leuven, Belgium, volume 220 of Proce-
dia Computer Science, pages 553–560. Elsevier.
Belanche, D., Casal
´
o, L. V., and Flavi
´
an, C. (2012). Inte-
grating trust and personal values into the technology
acceptance model: The case of e-government services
adoption. Cuadernos de Econom
´
ıa y Direcci
´
on de la
Empresa, 15(4):192–204.
Blanco, J. M., Ge, M., del Alamo, J. M., Due
˜
nas, J. C.,
and Cuadrado, F. (2023). A formal model for reli-
able digital transformation of water distribution net-
works. In Knowledge-Based and Intelligent Informa-
tion & Engineering Systems: Proceedings of the 27th
International Conference KES-2023, Athens, Greece,
6-8 September 2023, volume 225 of Procedia Com-
puter Science, pages 2076–2085. Elsevier.
B
¨
ockle, M., Yeboah-Antwi, K., and Kouris, I. (2021). Can
you trust the black box? the effect of personality traits
on trust in ai-enabled user interfaces. In International
Conference on Human-Computer Interaction, pages
3–20. Springer.
Davis, F. D. et al. (1989). Technology acceptance model:
Tam. Al-Suqri, MN, Al-Aufi, AS: Information Seeking
Behavior and Technology Adoption, pages 205–219.
Evans, A. M. and Krueger, J. I. (2009). The psychology
(and economics) of trust. Social and Personality Psy-
chology Compass, 3(6):1003–1017.
Falcone, R. and Castelfranchi, C. (2004). Trust dynamics:
How trust is influenced by direct experiences and by
trust itself. In Proceedings of the Third International
Joint Conference on Autonomous Agents and Multia-
gent Systems, 2004, pages 740–747. IEEE.
Frost, L. and Bauer, M. (2018). European trends in stan-
dardization for smart cities and society 5.0. NEC Tech-
nical Journal, 13(1):58–63.
Ge, M. and Buhnova, B. (2022). DISDA: digital ser-
vice design architecture for smart city ecosystems.
In Proceedings of the 12th International Conference
on Cloud Computing and Services Science, CLOSER
2022,, Online Streaming, April 27-29, 2022, pages
207–214. SCITEPRESS.
Guzman, A. L. and Lewis, S. C. (2020). Artificial intelli-
gence and communication: A human–machine com-
munication research agenda. New Media & Society,
22(1):70–86.
G
¨
uemes, C. and Herreros, F. (2019). Education and trust:
A tale of three continents. International Political Sci-
ence Review, 40(5):676–693.
Hillesheim, A. J., Rusnock, C. F., Bindewald, J. M., and
Miller, M. E. (2017). Relationships between user de-
mographics and user trust in an autonomous agent. In
Proceedings of the Human Factors and Ergonomics
Society Annual Meeting, volume 61, pages 314–318.
SAGE Publications Sage CA: Los Angeles, CA.
Kravtsova, M., Oshchepkov, A. Y., and Welzel, C. (2018).
The shadow of the family: Historical roots of social
capital in europe. (WP BRP 82/SOC/2018).
K
¨
uc¸
¨
uktabak, E. B., Kim, S. J., Wen, Y., Lynch, K., and
Pons, J. L. (2021). Human-machine-human interac-
tion in motor control and rehabilitation: a review.
Journal of neuroengineering and rehabilitation, 18:1–
18.
Labonne, J., Biller, D., and Chase, R. (2007). Inequality and
Relative Wealth: Do They Matter for Trust?: Evidence
from Poor Communities in the Philippines. Social De-
velopment Department, Community Driven Develop-
ment.
Lansing, A. E., Romero, N. J., Siantz, E., Silva, V., Cen-
ter, K., Casteel, D., and Gilmer, T. (2023). Building
trust: Leadership reflections on community empower-
ment and engagement in a large urban initiative. BMC
Public Health, 23(1):1252.
Le, S. H. (2013). Societal trust and geography. Cross-
Cultural Research, 47(4):388–414.
Lee, J., Baig, F., and Li, X. (2021). Media influence,
trust, and the public adoption of automated vehicles.
IEEE Intelligent Transportation Systems Magazine,
14(6):174–187.
Lemmers-Jansen, I. L., Fett, A.-K. J., van Os, J., Veltman,
D. J., and Krabbendam, L. (2020). Trust and the city:
Linking urban upbringing to neural mechanisms of
trust in psychosis. Australian & New Zealand Jour-
nal of Psychiatry, 54(2):138–149.
Ohnesorg, J., Fakhoury, N., Eltahawi, N., and Ge, M.
(2024). A review of ai-based trust management in
smart cities. In ITM Web of Conferences, volume 62,
page 01003. EDP Sciences.
Pagliaro, A. and Sangiorgi, P. (2023). Ai in experiments:
Present status and future prospects. Applied Sciences,
13(18):10415.
Persia, F., Pilato, G., Ge, M., Bolzoni, P., D’Auria, D.,
and Helmer, S. (2020). Improving orienteering-based
tourist trip planning with social sensing. Future
Gener. Comput. Syst., 110:931–945.
Sarker, I. (2022). Ai-based modeling: Techniques, applica-
tions and research issues towards automation, intelli-
gent and smart systems. SN Computer Science, 3.
Sechi, G., Borri, D., De Lucia, C., and Skilters, J. (2023).
How are personal wealth and trust correlated? a social
capital–based cross-sectional study from latvia. Inter-
national Social Science Journal.
Sundermier, A. (2023). New ai-driven tool streamlines ex-
periments. SLAC.
Thanetsunthorn, N. and Wuthisatian, R. (2019). Under-
standing trust across cultures: an empirical investiga-
tion. review of international business and strategy. 29
(4), 286-314.
Walletzk
´
y, L., Bayarsaikhan, O., Ge, M., and Schwarzov
´
a,
Z. (2022). Evaluation of smart city models: A concep-
tual and structural view. In Proceedings of the 11th
International Conference on Smart Cities and Green
ICT Systems, SMARTGREENS 2022, Online Stream-
ing, April 27-29, 2022, pages 56–65. SCITEPRESS.
Zhou, J., Luo, S., and Chen, F. (2020). Effects of personality
traits on user trust in human–machine collaborations.
Journal on Multimodal User Interfaces, 14:387–400.
Customizing Trust Systems: Personalized Communication to Address AI Adoption in Smart Cities
79