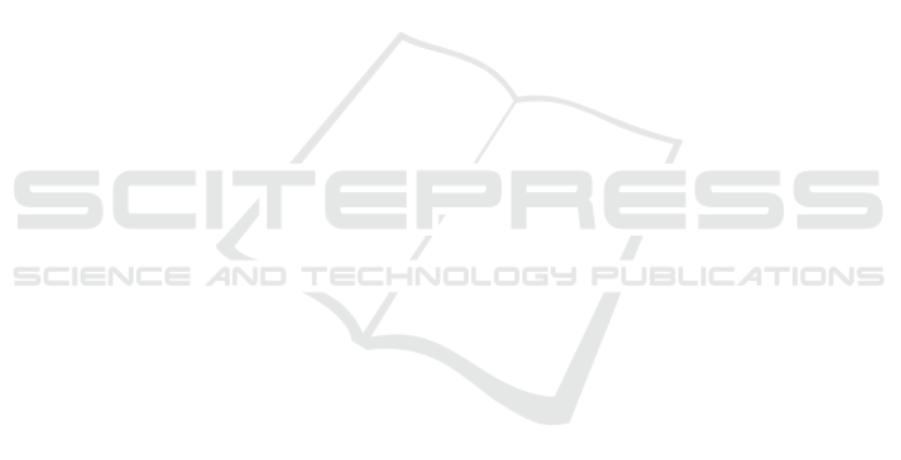
6 CONCLUSION AND FUTURE
WORK
Economic globalization has meant that business part-
ners (or companies) based in various parts of the
world can participate in the global product’s value
chains. As a result, products often travel many kilo-
meters around the world, involving different trans-
port companies. This phenomenon amplifies the
complexity of regulatory frameworks and legal juris-
dictions governing product manufacturing. Conse-
quently, within a product’s value chain, there may be
highly automated companies using IoT devices and
Artificial intelligence (AI), and also companies with-
out any form of digitalization.
Regardless of the level of digitalization of the
companies involved in the value chain, for imple-
menting the traceability of a product and store infor-
mation for calculating its sustainability index, there is
the need to collect and integrate data from all partici-
pants in the value chain, from the creation of raw ma-
terials, transport, manufacturing, etc. until it reaches
the final consumer. Before integrating the collected
data, in the moment of collecting it, the quality and
veracity of the data must be ensured. Thus, the data
must be validated in an homogeneous manner regard-
less of the level of digital maturity of the business
partner company.
This article has presented a solution for validating
the data collected by any of the business partners in-
volved in the T&C value chain, before integrating it in
a traceability platform. This article arises within the
scope of a project whose objective is to collect infor-
mation throughout the value chain of the textile and
clothing industries, in order to implement the digital
passport of products and allow the calculation of the
product’s sustainability index.
As future work, we intend to use Machine Learn-
ing algorithms, trained with the dataset being built
with the presented service, allowing for more dy-
namic and accurate data validation.
ACKNOWLEDGEMENTS
This contribution has been developed in the context
of Project ”BE@T: Bioeconomia Sustent
´
avel fileira
T
ˆ
extil e Vestu
´
ario-Medida 1”, funded by ”Plano de
Recuperac¸
˜
ao e Resili
ˆ
encia” (PRR), through mea-
sure TC-C12-i01 of the Portuguese Environmen-
tal Fund (”Fundo Ambiental”). For improving the
manuscript’s text some AI-based tools have been
used, such as Google Translator and Writefull. The
plots presented in the manuscript have been based on
a Chat-GPT suggested program in Python.
REFERENCES
Alves, L., Cruz, E. F., Lopes, S. I., Faria, P. M., and da Cruz,
A. M. R. (2022a). Towards circular economy in the
textiles and clothing value chain through blockchain
technology and iot: A review. Waste Management &
Research, 40(1):3–23. PMID: 34708680.
Alves, L., Cruz, E. F., and Rosado Da Cruz, A. M. (2022b).
Tracing sustainability indicators in the textile and
clothing value chain using blockchain technology. In
2022 17th Iberian Conference on Information Systems
and Technologies (CISTI), pages 1–7.
Alves, L., S
´
a, M., Cruz, E. F., Alves, T., Alves, M., Oliveira,
J., Santos, M., and Rosado da Cruz, A. M. (2024).
A traceability platform for monitoring environmen-
tal and social sustainability in the textile and clothing
value chain: Towards a digital passport for textiles and
clothing. Sustainability, 16(1).
Cichy, C. and Rass, S. (2019). An overview of data quality
frameworks. IEEE Access, 7:24634–24648.
Cruz, E. F. and da Cruz, A. M. R. (2020). Design science
research for is/it projects: Focus on digital transfor-
mation. In 15th Iberian Conf. on Information Systems
and Technologies (CISTI), pages 1–6.
Dias, R., Cruz, E. F., and Rosado Da Cruz, A. M. (2023).
Traceability platform for the textile and clothing value
chain. In 23 Confer
ˆ
encia da Associac¸
˜
ao Portuguesa
de Sistemas de Informac¸
˜
ao (CAPSI’2023), pages 1–7.
Even, A. and Shankaranarayanan, G. (2007). Utility-
driven assessment of data quality. SIGMIS Database,
38(2):75–93.
Hevner, A. R., March, S. T., Park, J., and Ram, S. (2004).
Design science in information systems research. MIS
Quarterly, 28(1):75–105.
Pipino, L. L., Lee, Y. W., and Wang, R. Y. (2002). Data
quality assessment. Commun. ACM, 45(4):211–218.
Sebastian-Coleman, L. (2012). Measuring Data Quality for
Ongoing Improvement: A Data Quality Assessment.
Morgan Kaufmann.
Wang, R. Y. and Strong, D. M. (1996). Beyond accuracy:
What data quality means to data consumers. J. Man-
age. Inf. Syst., 12(4):5–33.
Williams, A., Hodges, N., and Watchravesringkan, K.
(2023). An index is worth a thousand words: Con-
sidering consumer perspectives in the development of
a sustainability label. Cleaner and Responsible Con-
sumption, 11:100148.
Data Quality Assessment for the Textile and Clothing Value-Chain Digital Product Passport
295