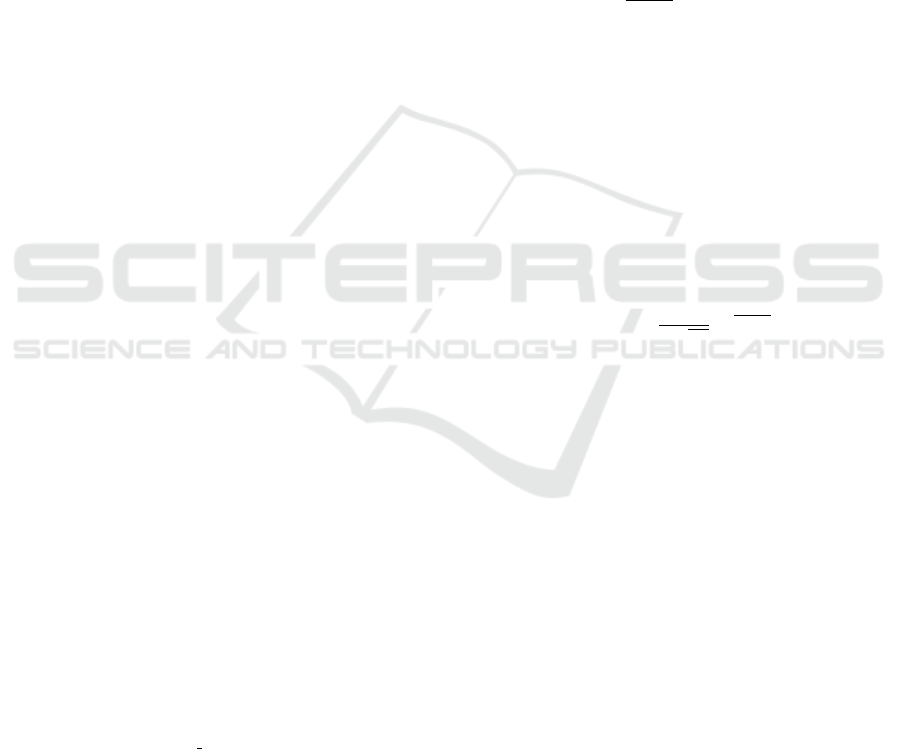
facilities versus nursing homes or the community. a
systematic review. PLOS ONE, 18(5):1–14.
Cornwell, E. Y. and Waite, L. J. (2009). Social discon-
nectedness, perceived isolation, and health among
older adults. Journal of Health and Social Behavior,
50(1):31–48. PMID: 19413133.
Ferrucci, L., Cooper, R., Shardell, M., Simonsick, E. M.,
Schrack, J. A., and Kuh, D. (2016). Age-related
change in mobility: perspectives from life course epi-
demiology and geroscience. Journals of gerontology
series a: biomedical sciences and medical sciences,
71(9):1184–1194.
Foley, D., Ancoli-Israel, S., Britz, P., and Walsh, J. (2004).
Sleep disturbances and chronic disease in older adults:
Results of the 2003 national sleep foundation sleep in
america survey. Journal of Psychosomatic Research,
56(5):497–502.
Holt-Lunstad, J., Smith, T. B., Baker, M., Harris, T.,
and Stephenson, D. (2015). Loneliness and so-
cial isolation as risk factors for mortality: A meta-
analytic review. Perspectives on Psychological Sci-
ence, 10(2):227–237. PMID: 25910392.
Kaiser, M. J., Bauer, J. M., R
¨
amsch, C., Uter, W., Guigoz,
Y., Cederholm, T., Thomas, D. R., Anthony, P. S.,
Charlton, K. E., Maggio, M., Tsai, A. C., Vellas, B.,
Sieber, C. C., and for the Mini Nutritional Assessment
International Group (2010). Frequency of malnutri-
tion in older adults: A multinational perspective using
the mini nutritional assessment. Journal of the Amer-
ican Geriatrics Society, 58(9):1734–1738.
Klompstra, L., Jaarsma, T., and Str
¨
omberg, A. (2013). An
in-depth, longitudinal examination of the daily physi-
cal activity of a patient with heart failure using a nin-
tendo wii at home: a case report. Journal of Rehabili-
tation Medicine, 45(6):599–602.
Lubitz, J., Cai, L., Kramarow, E., and Lentzner, H. (2003).
Health, life expectancy, and health care spending
among the elderly. New England Journal of Medicine,
349(11):1048–1055. PMID: 12968089.
Mowszowski, L., Hermens, D. F., Diamond, K., Norrie, L.,
Hickie, I. B., Lewis, S. J., and Naismith, S. L. (2012).
Reduced mismatch negativity in mild cognitive im-
pairment: associations with neuropsychological per-
formance. Journal of Alzheimer’s Disease, 30(1):209–
219.
Nielsen, L. (2018). Design personas – new ways, new con-
texts. Persona Studies, 4(2):1–4.
Nugraha, S. and Aprilia, T. (2019). Quality of life of the
elderly living in community and nursing home (study
on 5-dimension quality of life).
Rubenstein, L. Z. (2006). Falls in older people: epidemi-
ology, risk factors and strategies for prevention. Age
and Ageing, 35(suppl 2):ii37–ii41.
Volkert, D. (2013). Malnutrition in Older Adults - Urgent
Need for Action: A Plea for Improving the Nutritional
Situation of Older Adults. Gerontology, 59(4):328–
333.
APPENDIX
A Statistic Tools
The uniform distribution is characterized by a con-
stant probability density function over a specified in-
terval. In the context of behaviour modeling, it’s often
used to represent situations where all outcomes within
a range are equally likely. For example, it can be used
to model the probability to sit in the living room over
the afternoon. The distribution is defined by two pa-
rameters: the minimum and maximum values of the
interval.
f (x) =
1
t
2
−t
1
for t
1
≤ x ≤t
2
(4)
The Gaussian distribution, also known as the nor-
mal distribution, is one of the most widely used distri-
butions in statistics. It’s characterized by a symmetric
bell-shaped curve, with the mean (average) at the cen-
ter and the majority of the data clustered around the
mean. Many natural phenomena follow a normal dis-
tribution, making it particularly useful for modeling
behaviour when the underlying process is influenced
by multiple independent factors. An example is the
morning wake-up time, which revolves around a cer-
tain mean (µ) with a certain standard deviation (σ).
f (x) =
1
σ
√
2π
e
−
(x−µ)
2
2σ
2
(5)
behaviour modeling often involves analyzing the
time intervals between successive events. The expo-
nential distribution provides a mathematical frame-
work for modeling these inter-event times.
f (x;λ) = λe
−λx
(6)
Where λ represents the mean.
A Poisson process is a stochastic process that
models a sequence of events occurring randomly in
time or space. It is widely used in various fields such
as queueing theory, telecommunications, and relia-
bility engineering. The defining characteristics of a
Poisson process are:
1 Independence: Events occur independently of
each other.
2 Stationarity: The probability of an event occur-
ring in a given interval of time or space is the same
for all equivalent intervals.
3 Ordinariness: The process has no simultaneous
events; events occur singularly.
The key formulae associated with a Poisson pro-
cess include:
A Novel Approach in Testing Life-Monitoring Technologies for Ageing in Place: A Focus on Fall Detection and Behavioural Alerts
105