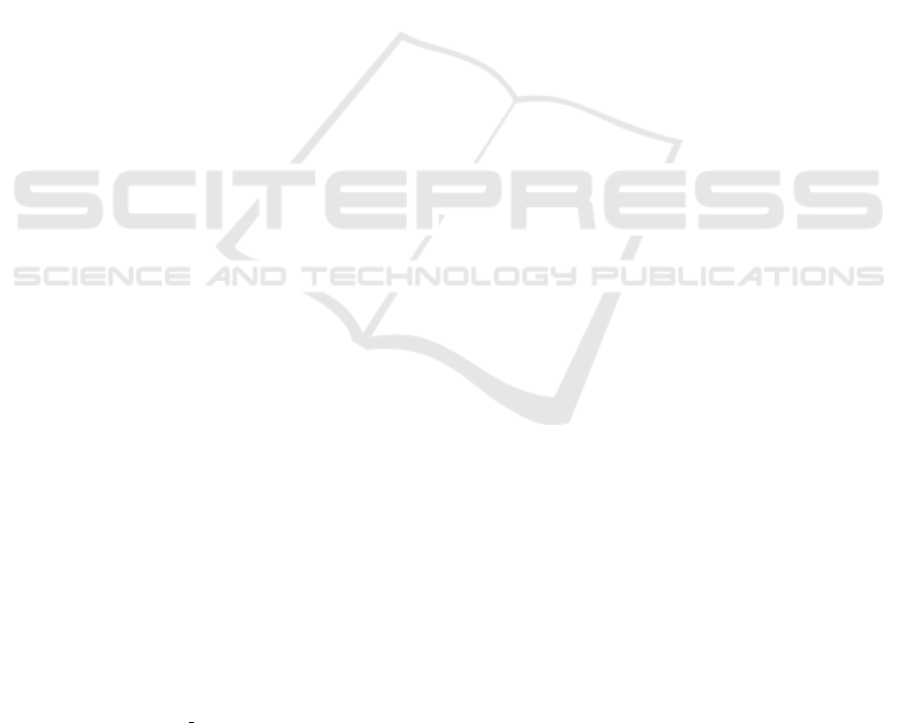
based on geospatial techniques. ISPRS International
Journal of Geo-Information, 11(4):260.
Horsman, G. and Conniss, L. R. (2015). Investigating evi-
dence of mobile phone usage by drivers in road traffic
accidents. Digital Investigation, 12:S30–S37.
Hu, L., Bao, X., Wu, H., and Wu, W. (2020). A study on cor-
relation of traffic accident tendency with driver char-
acters using in-depth traffic accident data. Journal of
advanced transportation, 2020:1–7.
Jianfeng, X., Hongyu, G., Jian, T., Liu, L., and Haizhu, L.
(2019). A classification and recognition model for the
severity of road traffic accident. Advances in Mechan-
ical Engineering, 11(5):1687814019851893.
Kasatkina, E. and Vavilova, D. (2023). Identification and
mitigation of road traffic accident concentration areas
by using cluster analysis. In 2023 5th International
Conference on Control Systems, Mathematical Mod-
eling, Automation and Energy Efficiency (SUMMA),
pages 247–251. IEEE.
Kudryavtsev, A. V., Kleshchinov, N., Ermolina, M., Lund,
J., Grjibovski, A. M., Nilssen, O., and Ytterstad, B.
(2013). Road traffic fatalities in arkhangelsk, russia in
2005–2010: Reliability of police and healthcare data.
Accident Analysis & Prevention, 53:46–54.
Lindsay, V. and Baldock, M. (2008). Medical conditions as
a contributing factor in crash causation.
Main Directorate for Traffic Safety of the Ministry of In-
ternal Affairs of Russia (2024). Information on road
safety indicators. retrieved february 22, 2024. http:
//stat.gibdd.ru/.
Marcillo, P., Valdivieso Caraguay,
´
A. L., and Hern
´
andez-
´
Alvarez, M. (2022). A systematic literature review
of learning-based traffic accident prediction models
based on heterogeneous sources. Applied Sciences,
12(9):4529.
Mooney, P., Minghini, M., et al. (2017). A review of open-
streetmap data. Mapping and the citizen sensor, pages
37–59.
Moosavi, S., Samavatian, M. H., Parthasarathy, S., Teodor-
escu, R., and Ramnath, R. (2019). Accident risk
prediction based on heterogeneous sparse data: New
dataset and insights. In Proceedings of the 27th ACM
SIGSPATIAL International Conference on Advances
in Geographic Information Systems, pages 33–42.
M
´
asilkov
´
a, M. (2017). Health and social consequences of
road traffic accidents. Kontakt, 19(1):e43–e47.
Open-Meteo contributors (2024). Open-meteo, official
website. retrieved february 22, 2024. https://open-m
eteo.com/en/docs/historical-weather-api.
OpenStreetMap Contributors (2024a). Openstreetmap. re-
trieved february 22, 2024. https://www.openstreetma
p.org.
OpenStreetMap Contributors (2024b). Overpass api. re-
trieved february 22, 2024. https://wiki.openstreetmap
.org/wiki/Overpass\ API.
Parker, D., Reason, J. T., Manstead, A. S., and Stradling,
S. G. (1995). Driving errors, driving violations and
accident involvement. Ergonomics, 38(5):1036–1048.
Rabbani, M. B. A., Musarat, M. A., Alaloul, W. S., Ayub,
S., Bukhari, H., and Altaf, M. (2022). Road accident
data collection systems in developing and developed
countries: a review. International Journal of Inte-
grated Engineering, 14(1):336–352.
Rodionova, M., Skhvediani, A., and Kudryavtseva, T.
(2022). Prediction of crash severity as a way of road
safety improvement: The case of saint petersburg, rus-
sia. Sustainability, 14(16):9840.
Rolison, J. J. (2020). Identifying the causes of road traffic
collisions: Using police officers’ expertise to improve
the reporting of contributory factors data. Accident
Analysis & Prevention, 135:105390.
Santos, D., Saias, J., Quaresma, P., and Nogueira, V. B.
(2021). Machine learning approaches to traffic ac-
cident analysis and hotspot prediction. Computers,
10(12):157.
Suat-Rojas, N., Gutierrez-Osorio, C., and Pedraza, C.
(2022). Extraction and analysis of social networks
data to detect traffic accidents. Information, 13(1):26.
Taylor, A. H. and Dorn, L. (2006). Stress, fatigue, health,
and risk of road traffic accidents among professional
drivers: the contribution of physical inactivity. Annu.
Rev. Public Health, 27:371–391.
The Federal Autonomous Institution ”Russian Road
Scientific-Research Institute” (2024). Road fund con-
trol system. retrieved february 22, 2024. https://xn--d
1aluo.xn--p1ai/.
Vogel, L. and Bester, C. (2005). A relationship between
accident types and causes. SATC 2005.
Wang, C., Quddus, M. A., and Ison, S. G. (2013). The effect
of traffic and road characteristics on road safety: A
review and future research direction. Safety science,
57:264–275.
Wang, X., Qu, Z., Song, X., Bai, Q., Pan, Z., and Li, H.
(2021). Incorporating accident liability into crash risk
analysis: A multidimensional risk source approach.
Accident Analysis & Prevention, 153:106035.
Zhang, Y., Lu, H., and Qu, W. (2020). Geographical detec-
tion of traffic accidents spatial stratified heterogeneity
and influence factors. International journal of envi-
ronmental research and public health, 17(2):572.
Zou, Y., Zhang, Y., and Cheng, K. (2021). Exploring the
impact of climate and extreme weather on fatal traffic
accidents. Sustainability, 13(1):390.
Zovak, G., Ku
ˇ
cini
´
c, T., and
ˇ
Sevo, I. (2016). Importance of
technical inspection of vehicles after traffic accidents.
Trans Motauto World, 1(4):3–6.
Overview of Publicly-Available Data Sources on Road Traffic Accidents in Russia
487