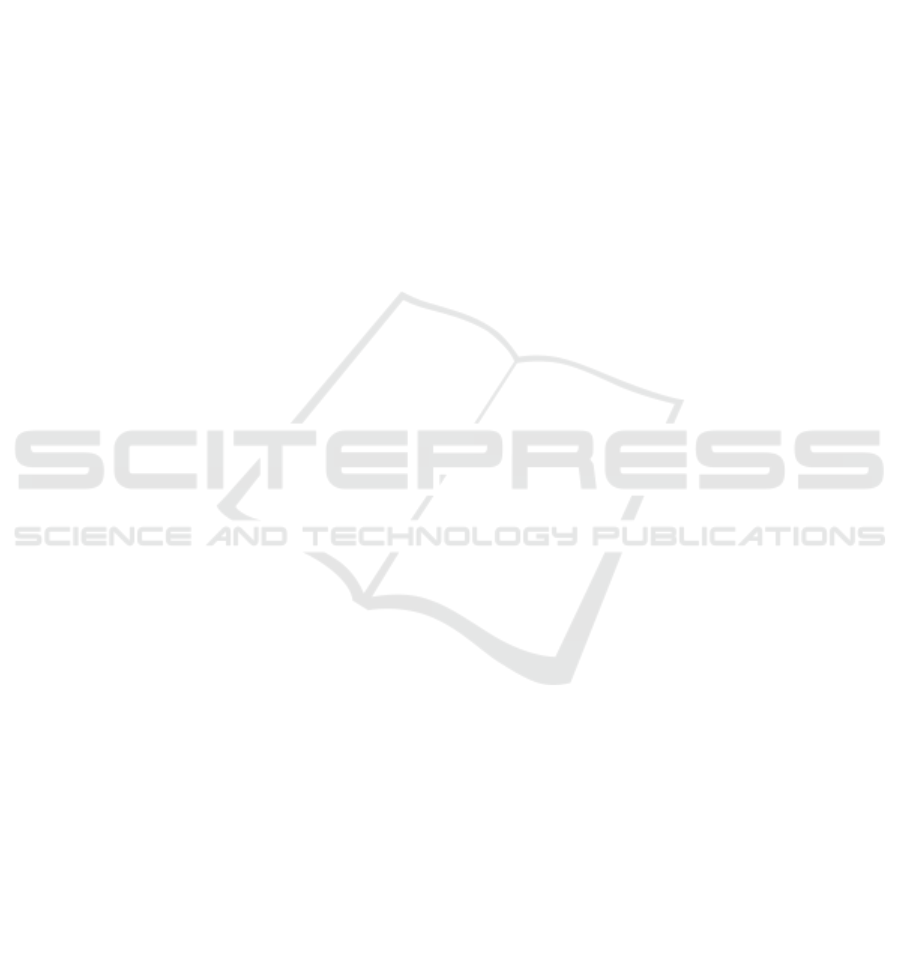
environments. Longitudinal studies could assess
students' perceptions of enjoyment and usefulness
with VR to determine if their high regard for it
persists beyond novelty. Additionally, extending
training sessions, duration, and incorporating
feedback strategies are essential future aims in both
research and practice.
Communicating effectively is an ability that needs
to be developed and mastered through the years. The
earlier we start teaching these skills, the easier it will
be to apply them properly in any communicative
situation. By nurturing these skills early on, educators
and parents empower young learners to communicate
effectively, collaborate productively, and navigate
the world with confidence and competence.
REFERENCES
Anderson, P. L., Zimand, E., Hodges, L. F., & Rothbaum,
B. O. (2005). Cognitive behavioral therapy for public-
speaking anxiety using virtual reality for exposure.
Depression and Anxiety, 22(3), 156-158.
Baños, R. M., Guillen, V., Quero, S., García-Palacios, A.,
Alcañiz, M., & Botella, C. (2011). A Virtual Reality
System for the Treatment of Stress-Related Disorders:
A Preliminary Analysis of Efficacy Compared to a
Standard Cognitive Behavioral Program. International
Journal of Human-Computer Studies, 69(9), 602-613.
Bianchi-Berthouze, N. (2013). Understanding the Role of
Body Movement in Player Engagement, Human-
Computer Interaction, 28(1), 40-75.
Boersma, P., & Weenink, D. (2007). PRAAT: Doing
Phonetics by Computer (Version 5.2.34). [Computer
software].
Bourdin, P., Martini, M., & Sanchez-Vives, M. V. (2019).
Altered visual feedback from an embodied avatar
unconsciously influences movement amplitude and
muscle activity. Scientific Reports, 9(1), [19747]. doi:
10.1038/s41598-019-56034-5
Daniels, M. M., Palaoag, T., & Daniels, M. (2020). Efficacy
of virtual reality in reducing fear of public speaking: A
systematic review. In International Conference on
Information Technology and Digital Applications,
803(1). doi: 10.1088/1757-899X/803/1/012003
De Jong, N. H., & Wempe, T. (2009). Praat script to detect
syllable nuclei and measure speech rate automatically.
Behavior research methods, 41(2), 385-390.
Ekman, P., & Friesen, W.V. (1969). The Repertoire or
Nonverbal Behavior: Categories, Origins, Usage and
Coding. Semiotica, 1, 49-98. doi:
10.1515/semi.1969.1.1.49
Ford, W. S. Z., & Wolvin, A. D. (1993). The differential
impact of a basic communication course on perceived
communication competencies in class, work, and social
contexts. Communication Education, 42, 215-233.
Hartshorne, J. K., Tenenbaum, J. B., & Pinker, S. (2018). A
critical period for second language acquisition:
Evidence from 2/3 million English speakers, Cognition,
177, 263-277. doi:10.1016/j.cognition.2018.04.007.
Huang, B. H., & Jun, S. A. (2011). The Effect of Age on the
Acquisition of Second Language Prosody. Language
and Speech, 54(3), 387-414. doi:
10.1177/0023830911402599
Hui Lim, M., Aryadoust, V., & Esposito, G. (2022). A
meta-analysis of the effect of virtual reality on reducing
public speaking anxiety. Current Psychology. Advance
online publication. doi: 10.1007/s12144-021-02684-6
Iberri-Shea, G. (2009). Using Public Speaking Tasks in
English Language Teaching. English Teaching Forum,
47(2), 18-23.
Kaufmann, R., & Tatum, N.T. (2017). Do we know what
we think we know? On the importance of replication in
instructional communication research. Communication
Education, 66, 479-481.
Kahlon, S., Lindner, P., & Nordgreen, T. (2019). Virtual
reality exposure therapy for adolescents with fear of
public speaking: A non-randomized feasibility and pilot
study. Child and Adolescent Psychiatry and Mental
Health, 13(47), 1-10.
Kendon, A. (2004) Gesture: Visible Action as Utterance.
Cambridge University Press, Cambridge.
King, P., Young, M., & Behnke, R. (2000). Public speaking
performance improvement as a function of information
processing in immediate and delayed feedback
interventions. Communication Education, 49(4), 365-
374. doi: 10.1080/03634520009379224
Liao, H. (2014). Examining the Role of Collaborative
Learning in a Public Speaking Course. College
Teaching, 62(2), 47-54, doi:
10.1080/87567555.2013.855891
Liu, X. & Xu, Y. (2014). Body size projection by voice
quality in emotional speech-Evidence from Mandarin
Chinese. Proceedings of the International Conference
on Speech Prosody, 974-977.
McNeill, D. (1992). Hand and mind: What gestures reveal
about thought. University of Chicago Press.
Morreale, S. P., Osborn, M. M., & Pearson, J. C. (2000).
Why communication is important: A rationale for the
centrality of the study of communication. Journal of the
Association for Communication Administration, 29(1),
1-25.
Munby, I. (2011). The oral presentation: An EFL teachers'
toolkit. Studies in Culture, 99, 143-168.
Nguyen, H. (2015). Student perceptions of the use of Pecha
Kucha presentations for EFL reading classes. Language
in Education in Asia, 6(2), 135-149.
Niebuhr, O. & Taghva, N. (2022, in review). Posture and
Gestures can affect the prosodic speaker impact in a
remote presentation. In M. Oliveira (Ed.), Prosodic
Interfaces. Berlin/New York: de Gruyter.
Niebuhr, O. & Michalsky, J. (2018). Virtual reality
simulations as a new tool for practicing presentations
and refining public-speaking skills. In Proceedings of
the 9th International Conference on Speech Prosody
2018, 309-313. International Speech Communication
Association (ISCA).
ERSeGEL 2024 - Workshop on Extended Reality and Serious Games for Education and Learning
692