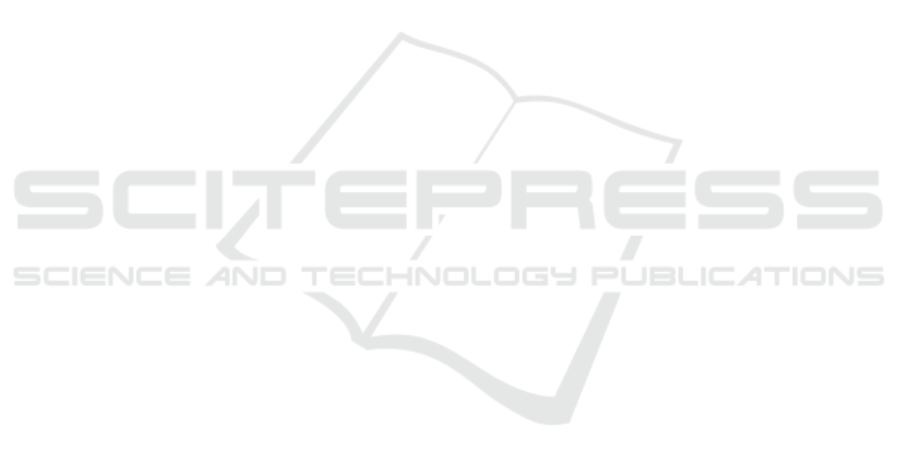
Competencies. Journal of Technology and Teacher
Education.
Gekara, V, Snell, D, Molla, A, K. . T. (2019). Skilling the
Australian Workforce for the Digital Economy - The
Australian Workforce Digital Skills Framework.
Government of Singapore, S. (2022). Skills Framework for
Infocomm Technology.
Haendler, T. and Neumann, G. (2019). A framework for the
assessment and training of software refactoring com-
petences. In KMIS, pages 307–316.
Hazar, E. (2019). A Comparison between European digi-
tal competence framework and the Turkish ICT cur-
riculum. Universal Journal of Educational Research,
7(4):954–962.
Ilom
¨
aki, L., Paavola, S., Lakkala, M., and Kantosalo, A.
(2016). Digital competence – an emergent boundary
concept for policy and educational research. Educa-
tion and Information Technologies, 21:655–679.
INTEF (2017). Common Digital Competence Framework
for Teachers (CDCFT).
Jacobs, L., Carboni, I., Hartley, B., Lindsey, D., Sibthorpe,
C., and Tiel Groenestege, M. (2021). Developing mo-
bile digital skills in low- and middle-income coun-
tries.
Johnson, A. (2022). Here’s What To Know About OpenAI’s
ChatGPT — What It’s Disrupting And How To Use It.
Kelentri
´
c, M., Karianne, H., and Arstorp, A.-T. (2017). Pro-
fessional Digital Competence Framework for Teach-
ers.
Kitchenham, B. and Charters, S. (2007). Guidelines for per-
forming Systematic Literature Reviews in Software
Engineering. EBSE Technical Report.
Krathwohl, D. R. (2002). A revision of bloom’s taxonomy:
An overview. Theory into practice, 41(4):212–218.
Law, N., Woo, D., de la Torre, J., and Wong, G. (2018). A
global framework of reference on digital literacy skills
for indicator 4.4. 2.
Llaneras, K., Rizzi, A., and
´
Alvarez, J. A. (2023). ChatGPT
is just the beginning: Artificial intelligence is ready to
transform the world.
Long, D., Teachey, A., and Magerko, B. (2022). What is AI
literacy? Competencies and design considerations. In
Proc. of ACM CHI, pages 1–20.
Mart
´
ınez, M. C., S
´
adaba, C., and Serrano-Puche, J. (2021).
Meta-framework of digital literacy: a comparative
analysis of 21st-century skills frameworks. Revista
Latina de Comunicacion Social, 79:76–110.
Mattar, J., Santos, C. C., and Cuque, L. M. (2022). Anal-
ysis and comparison of international digital compe-
tence frameworks for education. Education Sciences,
12(12).
Mediasmarts (2022). USE, UNDERSTAND & ENGAGE:
A Digital Media Literacy Framework for Canadian
Schools.
Minist
`
ere de l’
´
Education et de l’Enseignement sup
´
erieur
(2019). Digital Competency Framework.
Mishra, P. and Koehler, M. J. (2006). Technological peda-
gogical content knowledge: A framework for teacher
knowledge. Teachers College Record, 108:1017–
1054.
Nature Machine Intelligence (2023). What’s the next word
in large language models?
Ng, D. T. K., Leung, J. K. L., Chu, S. K. W., and Qiao, M. S.
(2021). Conceptualizing AI literacy: An exploratory
review. Computers and Education: Artificial Intelli-
gence, 2:100041.
Ng, D. T. K., Leung, J. K. L., Su, J., Ng, R. C. W., and Chu,
S. K. W. (2023). Teachers’ AI digital competencies
and twenty-first century skills in the post-pandemic
world. Educational technology research and devel-
opment, 71:137–161.
N
´
arosy, T., Schm
¨
olz, A., Proinger, J., and Domany-Funtan,
U. (2022). Digitales Kompetenzmodell f
¨
ur
¨
Osterreich.
Medienimpulse.
Object Management Group (2017). Unified Modeling Lan-
guage – version 2.5.1. https://www.omg.org/spec/
UML/2.5.1.
OpenAI (2022). Introducing ChatGPT.
Petersen, K., Feldt, R., Mujtaba, S., and Mattsson, M.
(2008). Systematic mapping studies in software en-
gineering. In Proc. of EASE, EASE’08, page 68–77.
BCS Learning & Development Ltd.
Ralph, P. et al. (2021). Empirical Standards for Software
Engineering Research.
Redecker, C. (2017). DigCompEdu.
Rosado, E. and Belisle, C. (2006). Analysing Digital Lit-
eracy Frameworks. A European framework for digital
literacy (eLearning Programme 2005-2006).
S
´
anchez-Canut, S., Usart-Rodr
´
ıguez, M., Grimalt-
´
Alvaro,
C., Mart
´
ınez-Requejo, S., Lores-G
´
omez, B., et al.
(2023). Professional Digital Competence: Definition,
Frameworks, Measurement, and Gender Differences:
A Systematic Literature Review. Human Behavior
and Emerging Technologies, 2023.
Santana, M. and D
´
ıaz-Fern
´
andez, M. (2023). Competen-
cies for the artificial intelligence age: visualisation of
the state of the art and future perspectives. Review of
Managerial Science, 17:1971–2004.
Sattelmaier, L. and Pawlowski, J. M. (2023). Towards a
Generative Artificial Intelligence Competence Frame-
work for Schools. In Proc. of ICOEINS), pages 291–
307. Atlantis Press.
SFIA Foundation (2021). Skills Framework for the Infor-
mation Age (SFIA - 8).
Shiohira, K. (2021). Understanding the Impact of Artificial
Intelligence on Skills Development. Education 2030.
Siddiq, F. (2018). A comparison between digital compe-
tence in two Nordic countries’ national curricula and
an international framework: Inspecting their readiness
for 21st century education. Seminar.net.
Siina, C., Fuller, S., and Sakamoto, J. (2022). Educators’
Digital Competency Framework.
Tomczyk, L. and Fedeli, L. (2021). Digital Literacy
among Teachers - Mapping Theoretical Frameworks:
TPACK, DigCompEdu, UNESCO, NETS-T, DigiLit
Leicester. Proc. of IBIMA, 23-24 November 2021,
Seville, Spain, pages 244–252.
EKM 2024 - 7th Special Session on Educational Knowledge Management
666