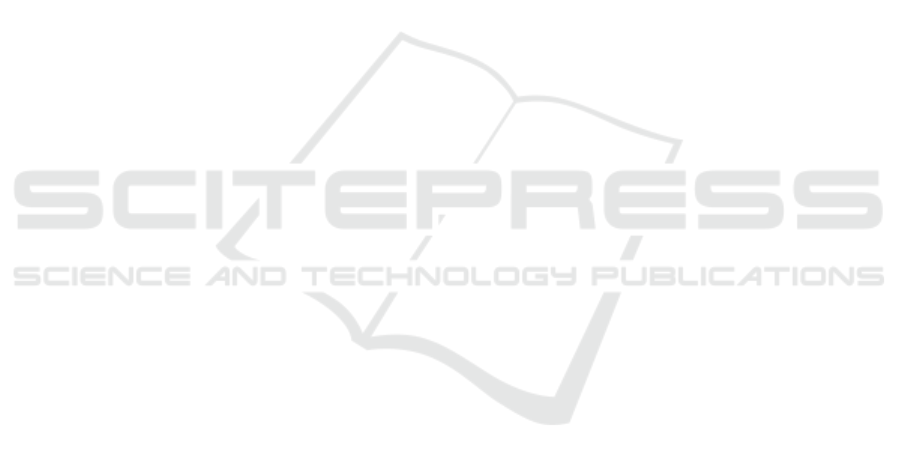
detection on data streams. Expert Systems with Appli-
cations, 117:90–102.
Ditzler, G. and Polikar, R. (2011). Hellinger distance based
drift detection for nonstationary environments. In
IEEE Symp. CIDUE, pages 41–48. IEEE.
dos Reis, D. M., Flach, P., Matwin, S., and Batista, G.
(2016). Fast unsupervised online drift detection using
incremental kolmogorov-smirnov test. In Proc. 22nd
ACM SIGKDD Int. Conf. KDD, pages 1545–1554.
Gama, J.,
ˇ
Zliobait
˙
e, I., Bifet, A., Pechenizkiy, M., and
Bouchachia, A. (2014). A survey on concept drift
adaptation. ACM computing surveys, 46(4):1–37.
Gemaque, R. N., Costa, A. F. J., Giusti, R., and Santos,
E. M. D. (2020). An overview of unsupervised drift
detection methods. Wiley Interdisciplinary Reviews:
Data Mining and Knowledge Discovery, 10(6):e1381.
Gocht, A., Sch
¨
one, R., and Frenzel, J. (2021). Advanced
python performance monitoring with score-p. In Tools
for High Performance Computing, pages 261–270.
Springer.
G
¨
oz
¨
uac¸ık,
¨
O., B
¨
uy
¨
ukc¸akır, A., Bonab, H., and Can, F.
(2019). Unsupervised concept drift detection with a
discriminative classifier. In Proc. 28th ACM Int. Conf.
CIKM, pages 2365–2368.
G
¨
oz
¨
uac¸ık,
¨
O. and Can, F. (2021). Concept learning us-
ing one-class classifiers for implicit drift detection in
evolving data streams. Artificial Intelligence Review,
54:3725–3747.
Greco, S. and Cerquitelli, T. (2021). Drift lens: Real-
time unsupervised concept drift detection by evaluat-
ing per-label embedding distributions. In Proc. IEEE
Int. Conf. ICDMW, pages 341–349. IEEE.
Grubitzsch, P., Werner, E., Matusek, D., Stojanov, V., and
H
¨
ahnel, M. (2021). Ai-based transport mode recogni-
tion for transportation planning utilizing smartphone
sensor data from crowdsensing campaigns. In Proc.
IEEE 24th Int. Conf. ITSC, pages 1306–1313. IEEE.
Gu, F., Zhang, G., Lu, J., and Lin, C. (2016). Concept drift
detection based on equal density estimation. In Proc.
Int. Conf. IJCNN, pages 24–30. IEEE.
Haque, A., Khan, L., and M. Baron, B. Thuraisingham,
C. A. (2016). Efficient handling of concept drift and
concept evolution over stream data. In Proc. IEEE
32nd Int. Conf. ICDE, pages 481–492. IEEE.
Harries, M., Wales, N. S., et al. (1999). Splice-2 compara-
tive evaluation: Electricity pricing.
Hawkins, D. M. (2004). The problem of overfitting. Jour-
nal of chemical information and computer sciences,
44(1):1–12.
Hoens, T. R., Polikar, R., and Chawla, N. V. (2012). Learn-
ing from streaming data with concept drift and imbal-
ance: an overview. Progress in Artificial Intelligence,
1:89–101.
Hulten, G., Spencer, L., and Domingos, P. (2001). Min-
ing time-changing data streams. In Proc. 7th ACM
SIGKDD Int. Conf. KDD, pages 97–106.
Ikonomovska, E., Gama, J., and D
ˇ
zeroski, S. (2011). Learn-
ing model trees from evolving data streams. Data
Mining and Knowledge Discovery, 23:128–168.
Jin, G., Song, L., Shi, X., Scherpelz, J., and Lu, S. (2012).
Understanding and detecting real-world performance
bugs. ACM SIGPLAN Notices, 47(6):77–88.
Kifer, D., Ben-David, S., and Gehrke, J. (2004). Detecting
change in data streams. In Proc. 30th Int. Conf VLDB,
volume 4, pages 180–191. Toronto, Canada.
Kim, Y. and Park, C. H. (2017). An efficient concept drift
detection method for streaming data under limited la-
beling. IEICE Transactions on Information and sys-
tems, 100(10):2537–2546.
Kn
¨
upfer, A., Brunst, H., Doleschal, J., Jurenz, M., Lieber,
M., Mickler, H., M
¨
uller, M. S., and Nagel, W. E.
(2008). The vampir performance analysis tool-set. In
Resch, M., Keller, R., Himmler, V., Krammer, B., and
Schulz, A., editors, Tools for High Performance Com-
puting, pages 139–155. Springer Berlin Heidelberg.
Kn
¨
upfer, A., R
¨
ossel, C., Mey, D., Biersdorff, S., Diethelm,
K., Eschweiler, D., Geimer, M., Gerndt, M., Lorenz,
D., Malony, A., et al. (2012). Score-p: A joint
performance measurement run-time infrastructure for
periscope, scalasca, tau, and vampir. In Proc. 5th Int.
Workshop on Parallel Tools for HPC, pages 79–91.
Springer.
Kumar, N.,
ˇ
Segvi
´
c, S., Eslami, A., and Gumhold, S. (2023).
Normalizing flow based feature synthesis for outlier-
aware object detection. In Proc. IEEE Conf. CVPR,
pages 5156–5165.
Langenk
¨
amper, D., Kevelaer, R. V., Purser, A., and Nat-
tkemper, T. W. (2020). Gear-induced concept drift in
marine images and its effect on deep learning classifi-
cation. Frontiers in Marine Science, 7:506.
Leskovec, J., Lang, K. J., Dasgupta, A., and Mahoney,
M. W. (2009). Community structure in large net-
works: Natural cluster sizes and the absence of large
well-defined clusters. Internet Mathematics, 6(1):29–
123.
Liu, A., J.Lu, Liu, F., and Zhang, G. (2018). Accumulat-
ing regional density dissimilarity for concept drift de-
tection in data streams. Pattern Recognition, 76:256–
272.
Liu, A., Song, Y., Zhang, G., and Lu, J. (2017). Regional
concept drift detection and density synchronized drift
adaptation. In Proc. 26th Int. Conf. IJCAI.
Lu, N., Zhang, G., and Lu, J. (2014). Concept drift detec-
tion via competence models. Artificial Intelligence,
209:11–28.
Lughofer, E., Weigl, E., Heidl, W., Eitzinger, C., and
Radauer, T. (2016). Recognizing input space and tar-
get concept drifts in data streams with scarcely la-
beled and unlabelled instances. Information Sciences,
355:127–151.
Lukats, D. and Stahl, F. (2023). On reproducible implemen-
tations in unsupervised concept drift detection algo-
rithms research. In Proc. 43rd Int. Conf. SGAI, pages
204–209. Springer.
Mahgoub, M., Moharram, H., Elkafrawy, P., and Awad, A.
(2022). Benchmarking concept drift detectors for on-
line machine learning. In Proc. 11th Int. Conf. MEDI,
pages 43–57. Springer.
Towards Computational Performance Engineering for Unsupervised Concept Drift Detection: Complexities, Benchmarking, Performance
Analysis
327