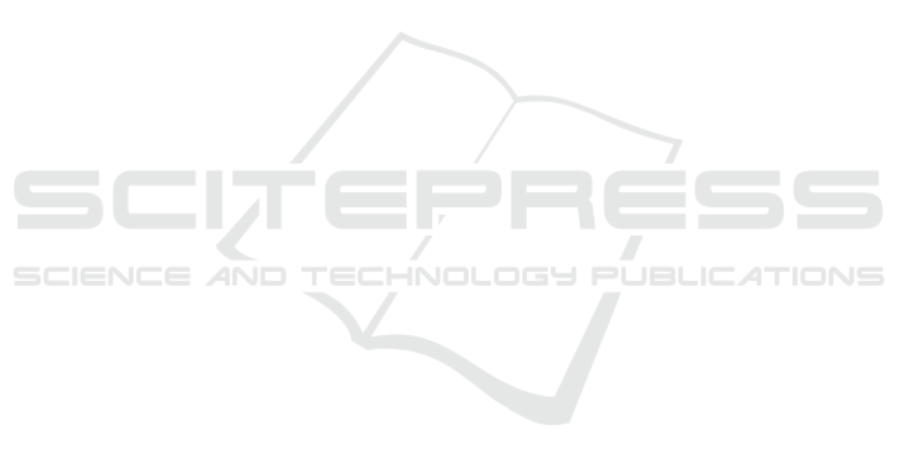
REFERENCES
Alrabaee, S., Debbabi, M., and Wang, L. (2019). On the
feasibility of binary authorship characterization. Dig-
ital Investigation, 28:S3–S11.
Alrabaee, S., Saleem, N., Preda, S., Wang, L., and Debbabi,
M. (2014). Oba2: An onion approach to binary code
authorship attribution. Digital Investigation, 11:S94–
S103. Annual DFRWS Europe.
Balcan, M.-F. and Long, P. (2013). Active and passive
learning of linear separators under log-concave distri-
butions. In COLT, volume 30 of Proceedings of Ma-
chine Learning Research, pages 288–316, Princeton,
NJ, USA. PMLR.
Burnaev, E. and Smolyakov, D. (2016). One-class svm with
privileged information and its application to malware
detection. In ICDMW, pages 273–280, Los Alamitos,
CA, USA. IEEE Computer Society.
Cohn, D., Ghahramani, Z., and Jordan, M. (1994). Active
learning with statistical models. In NIPS, volume 7.
MIT Press.
da Silva Freitas Junior, J. and Pisani, P. H. (2022). Perfor-
mance and model complexity on imbalanced datasets
using resampling and cost-sensitive algorithms. In
IWLID 2022, volume 183 of Proceedings of Machine
Learning Research, pages 83–97. PMLR.
Deng, Y., Chen, K., Shen, Y., and Jin, H. (2018). Adversar-
ial active learning for sequences labeling and genera-
tion. In IJCAI, pages 4012–4018. International Joint
Conferences on Artificial Intelligence Organization.
Garcia, S., Grill, M., Stiborek, J., and Zunino, A. (2014).
An empirical comparison of botnet detection methods.
Comput. Secur., 45:100–123.
Haddadpajouh, H., Azmoodeh, A., Dehghantanha, A., and
Parizi, R. M. (2020). Mvfcc: A multi-view fuzzy con-
sensus clustering model for malware threat attribution.
IEEE Access, 8:139188–139198.
Han, Y. and Shen, Y. (2016). Accurate spear phishing cam-
paign attribution and early detection. In ACM SAC
2016, SAC ’16, page 2079–2086, New York, NY,
USA. Association for Computing Machinery.
Jaafar, F., Avellaneda, F., and Alikacem, E.-H. (2020).
Demystifying the cyber attribution: An exploratory
study. In (DASC 2020, pages 35–40.
Karev, D., McCubbin, C., and Vaulin, R. (2017). Cyber
threat hunting through the use of an isolation forest. In
ICCST, CompSysTech ’17, page 163–170, New York,
NY, USA. Association for Computing Machinery.
Kim, J., Sim, A., Kim, J., Wu, K., and Hahm, J. (2020).
Transfer learning approach for botnet detection based
on recurrent variational autoencoder. In IWSNTAA,
SNTA ’20, page 41–47, New York, NY, USA. Associ-
ation for Computing Machinery.
Lee, I. and Choi, C. (2023). Camp2vec: Embedding cy-
ber campaign with attck framework for attack group
analysis. ICT Express, 9(6):1065–1070.
Lewis, D. D. and Catlett, J. (1994). Heterogeneous un-
certainty sampling for supervised learning. In ICML,
pages 148–156. Morgan Kaufmann.
Moya, M. M. and Hush, D. R. (1996). Network constraints
and multi-objective optimization for one-class classi-
fication. Neural Networks, 9(3):463–474.
Nisioti, A., Mylonas, A., Yoo, P. D., and Katos, V.
(2018). From intrusion detection to attacker attribu-
tion: A comprehensive survey of unsupervised meth-
ods. IEEE Communications Surveys and Tutorials,
20(4):3369–3388.
Pitropakis, N., Panaousis, E., Giannakoulias, A., Kalpakis,
G., Rodriguez, R. D., and Sarigiannidis, P. (2018). An
enhanced cyber attack attribution framework. In Fur-
nell, S., Mouratidis, H., and Pernul, G., editors, Trust,
Privacy and Security in Digital Business, pages 213–
228, Cham. Springer International Publishing.
Plessis, M. D., Niu, G., and Sugiyama, M. (2015). Convex
formulation for learning from positive and unlabeled
data. In ICML, volume 37 of Proceedings of Machine
Learning Research, pages 1386–1394.
Ren, Y., Xiao, Y., Zhou, Y., Zhang, Z., and Tian, Z. (2023).
Cskg4apt: A cybersecurity knowledge graph for ad-
vanced persistent threat organization attribution. IEEE
TKDE, 35(06):5695–5709.
Rosenberg, I., Sicard, G., and David, E. O. (2017). Deep-
apt: Nation-state apt attribution using end-to-end deep
neural networks. In Lintas, A., Rovetta, S., Verschure,
P. F., and Villa, A. E., editors, Artificial Neural Net-
works and Machine Learning – ICANN 2017, pages
91–99, Cham. Springer International Publishing.
Sahoo, D. (2022). Cyber Threat Attribution with Multi-View
Heuristic Analysis, pages 53–73. Springer Interna-
tional Publishing, Cham.
Sarhan, M., Layeghy, S., Moustafa, N., and Portmann,
M. (2020). Netflow datasets for machine learning-
based network intrusion detection systems. CoRR,
abs/2011.09144.
Silva, D., Dell’Amico, M., Hart, M., Roundy, K. A., and
Kats, D. (2018). Hierarchical incident clustering for
security operation centers. In IDEA’18, August 20,
2018, London, England.
Sinha, S., Ebrahimi, S., and Darrell, T. (2019). Variational
adversarial active learning. In ICCV, pages 5971–
5980, Los Alamitos, CA, USA. IEEE Computer So-
ciety.
Wen, Z. and Li, Y. (2021). Toward understanding the feature
learning process of self-supervised contrastive learn-
ing. In ICML, volume 139 of Proceedings of Machine
Learning Research, pages 11112–11122. PMLR.
Yang, L., Guo, W., Hao, Q., Ciptadi, A., Ahmadzadeh, A.,
Xing, X., and Wang, G. (2021). CADE: Detecting
and explaining concept drift samples for security ap-
plications. In USENIX Security 21, pages 2327–2344.
USENIX Association.
Zhang, L., Thing, V. L., and Cheng, Y. (2019). A scalable
and extensible framework for android malware detec-
tion and family attribution. Computers and Security,
80:120–133.
Zhou, D., Bousquet, O., Lal, T., Weston, J., and Sch
¨
olkopf,
B. (2003). Learning with local and global consistency.
In NIPS, volume 16. MIT Press.
SECRYPT 2024 - 21st International Conference on Security and Cryptography
102