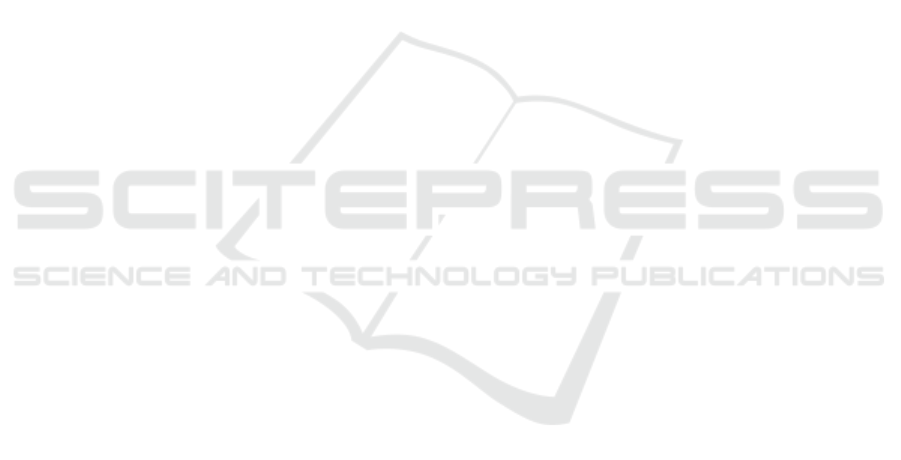
facturers and retailers included in the survey and the
seven expert interviews was not representative. Al-
though survey and the interviews were conducted with
companies of different sizes and focal points, it would
be beneficial to validate them within a larger sample
in order to achieve more meaningful results for the
development of the AI-based prediction and recom-
mendation system. A field test with customers who are
confronted with the concrete ideas of the present study,
such as the introduction of return fees depending on
the probability of returns determined by the system,
could also be recommended. This would allow the
effectiveness of the proposed measures to be tested.
Nevertheless, AI-based prediction and recommenda-
tion systems are not sufficient to address the issue of
returns alone. Consequently, it is essential that future
research also concentrates on topics such as process
optimisation in the context of returns processes and
reverse logistics.
ACKNOWLEDGEMENTS
This research was funded in part by the Ger-
man Federal Ministry of Education and Research
(BMBF) under the project OptiRetouren (grant num-
ber 01IS22046B). It is a joint project of the August-
Wilhelm Scheer Institut, INTEX, HAIX and h+p.
August-Wilhelm Scheer Institut is mainly entrusted
with conducting research in AI for predicting returns
volume and for recommendations based on AI.
REFERENCES
Asdecker, B. and Karl, D. (2018). Big data analytics in
returns management-are complex techniques necessary
to forecast consumer returns properly? In 2nd Interna-
tional Conference on Advanced Research Methods and
Analytics. Proceedings, pages 39–46.
Asdecker, B., Karl, D., and Sucky, E. (2017). Examining
drivers of consumer returns in e-tailing with real shop
data. In Hawaii International Conference on System
Sciences, pages 4192–4201.
Bimschleger, C., Patel, K., and Leddy, M. (2019). Bringing
it back: Retailers need a synchronized reverse logistics
strategy. Technical report, Deloitte Development LLC.
Deges, F. (2021). Retourencontrolling im online-handel.
Controlling – Zeitschrift f
¨
ur erfolgsorientierte Un-
ternehmenssteuerung, 2/2021:61–68.
Fitzpatrick, R. (2013). The Mom Test: How to talk to cus-
tomers & learn if your business is a good idea when
everyone is lying to you. Robfitz Ltd.
Forschungsgruppe Retourenmanagement (2022).
Ergebnisse des europ
¨
aischen retourentachos
ver
¨
offentlicht. https://www.retourenforschung.de/info-
ergebnisse-des-europaeischen-retourentachos-
veroeffentlicht.html. Online; accessed 2024-03-05.
Gry, S., Niederlaender, M., Lodi, A., Mutz, M., and Werth,
D. (2023). Advances in ai-based garment returns pre-
diction and processing: A conceptual approach for an
ai-based recommender system. In Proceedings of the
20th International Conference on Smart Business Tech-
nologies - ICSBT, pages 15–25. INSTICC, SciTePress.
Lepthien, A. and Clement, M. (2019). Shipping fee sched-
ules and return behavior. Marketing Letters, 30(2):151–
165.
Lohmeier (2024). E-commerce in deutschland:
Daten und fakten zum boomenden onli-
negesch
¨
aft. https://de.statista.com/themen/247/e-
commerce/
♯
topicOverview. Online; accessed
2024-02-29.
Makkonen, M., Frank, L., and Kemppainen, T. (2021).
The effects of consumer demographics and payment
method preference on product return frequency and rea-
sons in online shopping. In Bled eConference, pages
567–580. University of Maribor.
Morgan Stanley (2022). Here’s why e-commerce growth can
stay stronger for longer. https://www.morganstanley.
com/ideas/global-ecommerce-growth-forecast-2022/.
Online; accessed 2024-02-29.
Niederlaender, M., Lodi, A., Gry, S., Biswas, R., and Werth,
D. (2024). Garment returns prediction for ai-based
processing and waste reduction in e-commerce. In
Proceedings of the 16th International Conference on
Agents and Artificial Intelligence - Volume 2: ICAART,
pages 156–164. INSTICC, SciTePress.
Saarij
¨
arvi, H., Sutinen, U.-M., and Harris, L. C. (2017). Un-
covering consumers’ returning behaviour: a study of
fashion e-commerce. The International Review of Re-
tail, Distribution and Consumer Research, 27(3):284–
299.
Sahoo, N., Dellarocas, C., and Srinivasan, S. (2018). The
impact of online product reviews on product returns.
Information Systems Research, 29(3):723–738.
Shehu, E., Papies, D., and Neslin, S. A. (2020). Free shipping
promotions and product returns. Journal of Marketing
Research, 57(4):640–658.
Statista Market Insights (2024). ecommerce - weltweit.
https://de.statista.com/outlook/emo/ecommerce/
weltweit? currency=eur. Online; accessed 2024-02-29.
Sutinen, U.-M., Saarij
¨
arvi, H., and Yrj
¨
ol
¨
a, M. (2022). Shop
at your own risk? consumer activities in fashion e-
commerce. International Journal of Consumer Studies,
46(4):1299–1318.
Yan, R. and Cao, Z. (2017). Product returns, asymmetric
information, and firm performance. International Jour-
nal of Production Economics, 185:211–222.
Enhancing Returns Management in Fashion E-Commerce: Industry Insights on AI-Based Prediction and Recommendation Systems
73