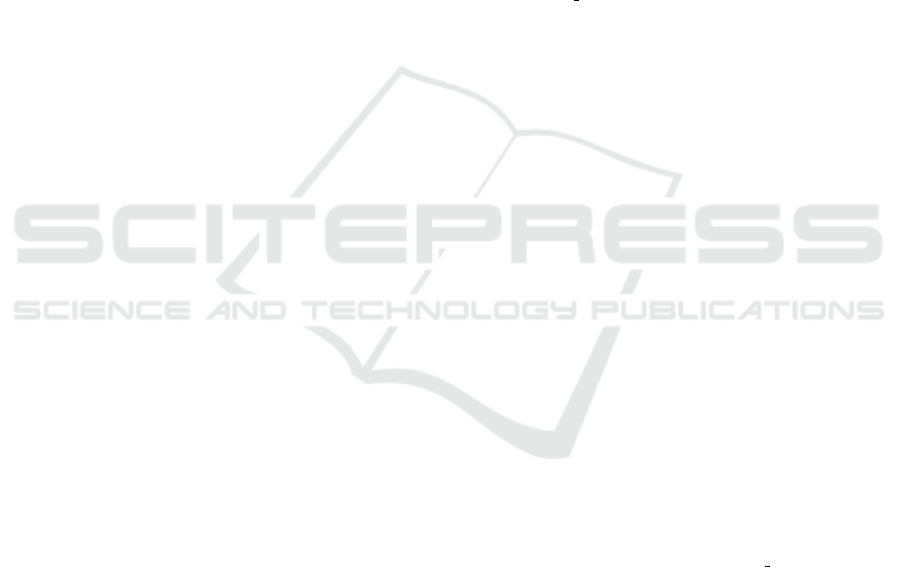
sentiment” is to inflate all positive sentiment figures
by 4%, leaving negative sentiment figures unchanged.
The results of this study are significant for two rea-
sons. First, quantification of negative bias using au-
tomated methods coupled with exhaustive data min-
ing is a much more reliable than one-off survey meth-
ods. Therefore, we can place considerable reliance on
the final proposed figure of 4%. Second, the discrep-
ancy between the positive/negative sentiment balance
shows that the context in which measurement takes
place (sources and media), and geographical region
are both important. Generalisation to other contexts
and regions is unsafe.
ACKNOWLEDGEMENTS
We acknowledge the continuing support and assis-
tance of the staff of Penta Group.
REFERENCES
Antypas, D., P. A. and Camacho-Collados, J. (2023). Nega-
tivity spreads faster: A large-scale multilingual twitter
analysis on the role of sentiment in political commu-
nication. In Online Social Networks and Media 33.
doi:10.1016/j.osnem.2023.100242.
Bellovary, A., Y. N. and Goldenberg, A. (2021). Left-
and right-leaning news organizations’ negative tweets
are more likely to be shared. In PsyArXiv 24.
doi:10.31234/osf.io/2er67.
Carter, C. K. and Kohn, R. (1994). On gibbs sampling for
state space models. In Biometrika, 81(3), pp. 541–53.
doi:/10.2307/2337125.
Chomsky, N. (1957). Syntactic structures. Mouton.
Ferrara, E. and Yang, Z. (2015). Quantifying the effect
of sentiment on information diffusion in social media.
In Jnl. Compututer Science 1(51). doi:10.7717/peerj-
cs.26.
Finkelstein, S. R. and Fishbach, A. (2012). Tell me what i
did wrong: Experts seek and respond to negative feed-
back. In Journal of Consumer Research, 39(1), 22–38.
https://doi.org/10.1086/661934.
Fruehwirth-Schnatter, S. (1994). Data augmentation and
dynamic linear models. In Journal of Time Se-
ries Analysis, 15(2), pp. 183–202. doi:10.1111/j.1467-
9892.1994.tb00184.x.
Harvey, A. (1990). Forecasting, structural time series mod-
els and the kalman filter. In Cambridge University
Press.
Hu, N, . P. P. and Zhang, J. (2007). Why do online prod-
uct reviews have a j-shaped distribution? NYU Stern
Business School http://hdl.handle.net/2451/14951.
Liu, B. (2015). Sentiment Analysis: Mining Opinions, Sen-
timents and Emotions. CUP, New York, 1st edition.
Loke, R. and Kisoen, Z. (2022). The role of fake review
detection in managing online corporate reputation. In
Proc, 11th International Conference on Data Science,
Technology and Applications (DATA 2022), pp 245-
256. ScitePress doi:10.5220/0011144600003269.
Loke, R. and Vergeer, J. (2022). Exploring corporate repu-
tation based on sentiment polarities that are related to
opinions in dutch online reviews. In Proc, 11th Inter-
national Conference on Data Science, Technology and
Applications (DATA 2022), pp 423-431. ScitePress
doi:10.5220/0011285500003269.
Mitic, P. (2015). Improved goodness-of-fit tests for opera-
tional risk. In Journal of Operational Risk, 15(1), pp
77-126. Incisive Media doi:10.21314/JOP.2015.159.
Mitic, P. (2017). Standardised reputation measure-
ment. In IDEAL 2017, Guilin China (H. Yin
et al., eds.), LNCS 10585 534-542. Springer:
https://link.springer.com/chapter/10.1007/978-3-319-
68935-7 58.
Moe, W. and Schweidel, D. (2012). Online prod-
uct opinions: Incidence, evaluation and evolu-
tion. In Marketing Science, 31(3) , pp. 372–386.
https://doi.org/10.1287/mksc.1110.0662.
Moe, W. and Schweidel, D. (2013). Positive, negative
or not at all? what drives consumers to post (accu-
rate) product reviews? In Insights 5(2), pp. 8–12.
doi:10.2478/gfkmir-2014-0011.
OpenAI (2019). Better language models and their impli-
cations. https://openai.com/research/better-language-
models.
Park, K., C. M. and Rhim, E. (2018). Positiv-
ity bias in customer satisfaction ratings. In
Proc. WWW ’18, Lyon France, pp. 631-638.
doi.org/10.1145/3184558.3186579.
Powell, D., Y. J. D. M. and Holyoak, K. J. (2017). The
love of large numbers: A popularity bias in consumer
choice. In Psychological Science, 28(10), pp. 1432-
1442. doi.org/10.1177/0956797617711291.
Reichheld, F. F. (2003). The one number you need
to grow. In Harvard Business Review 81(12)
pp. 46–54. https://hbr.org/2003/12/the-one-number-
you-need-to-grow.
Rozin, P. and Royzman, E. B. (2001). Negativity bias,
negativity dominance, and contagion. In Personal-
ity and Social Psychology Review. 5(4) pp. 296–320.
doi:10.1207/S15327957PSPR0504 2.
Shumway, R. and Stoffer, D. (2016). Time Series Analy-
sis and Its Applications With R Examples: Chapter 6.
Springer.
Stieglitz, S. and Dang-Xuan, L. (2013). Emotions
and information diffusion in social media–sentiment
of microblogs and sharing behavior. In Jnl.
Management Info. Systems 29(4) pp. 217–247.
doi:10.2753/MIS0742-1222290408.
Tsugawa, S. and Ohsaki, H. (2017). On the relation be-
tween message sentiment and its virality on social me-
dia. In Social Network Analysis and Mining 7(1):19.
doi:10.1007/s13278-017-0439-0.
Yeo, I.-K. and Johnson, R. A. (2000). A new fam-
ily of power transformations to improve normal-
DATA 2024 - 13th International Conference on Data Science, Technology and Applications
80