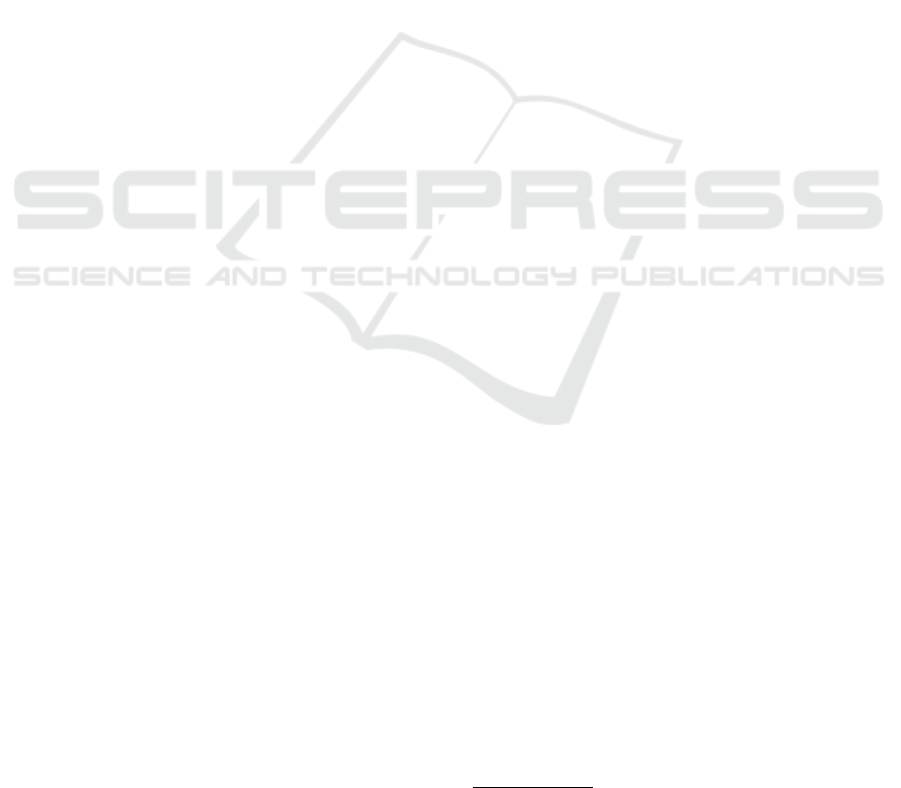
tifically sound framework describing what it means
for educators to be digitally competent” (Redecker,
2017). Since then there has been significant re-
search investigating and implementing the framework
within European educational institutions (Buckley
and Pears, 2021; Economou, 2023), including several
surveys that evaluate digital competencies using qual-
itative (Ghomi and Redecker, 2019; Rubio-Gragera
et al., 2023) means. In the context of music educa-
tion there have been several surveys published (Pabst-
Krueger and Ziegenmeyer, ), with many focussing
on the Spanish system (Guill
´
en-G
´
amez and Ramos,
2021; Garc
´
ıa et al., 2021; Cuervo et al., 2023)
The paper is structured as follows. Section 2 de-
scribes the methodology from instrument design to.
Section 3 discusses some of the results. Section 4
concludes with some overall remarks and discussion
of the next steps.
2 METHODOLOGY
2.1 Survey Design and Pilot
The survey design phase commenced with a series of
online brainstorming sessions between the 3 partner
conservatoires in an effort to provoke discussion, so-
licit ideas and generate as many possible questions as
possible. These were all collected loosely in shared
documents that allowed further review and discussion
after formal meetings via comments.
Questions were refined based on relevance or re-
dundancy and transferred to a spreadsheet were or-
ganised thematically into sections that align with the
following areas of study of the project (as well as
an introductory ”general profile” category to aid with
subgrouping and data slicing later):
1. General Profile
2. Basic and transversal digital skills
3. Online safety and addressing ethics within the
new technological environment
4. Pedagogical skills
5. Specific Skills for digital teachers
Question types (e.g. multiple choice versus mul-
tiple answer) and possible options for the questions
were captured. Next the questions were coded into a
Microsoft Forms survey. Using manual and machine-
assisted translation the surveys were localised for
Spanish and Estonian. This pilot survey was then
shared with 5 colleagues from partner institutions but
who were not associated directly with the project for
independent feedback and testing.
2.2 Data Collection
After addressing pilot feedback, the survey was re-
fined and finalised for collection from the sample pop-
ulation. The survey was shared firstly amongst col-
leagues within the three conservatory partner institu-
tions, then widened to other institutions in the net-
works of the partners. In addition we targeted spe-
cific organisations and social media channels related
to music pedagogy, particularly the Association of
European Conservatoires (AEC).
1
2.3 Data Analysis
Following the closing of the data collection window,
the survey results were downloaded in Excel format
from the Microsoft Forms backend. The data was
ingested into Python using the Pandas data analysis
and manipulation library (pandas development team,
2024; McKinney, 2010). Readable ordinal categories
such as age, years teaching and Likert ratings were
replaced with integers to aid numerical analysis.
Preliminary descriptive statistics were carried out
using pandas and numpy. Inferential statistics were
performed using statsmodel (Seabold and Perktold,
2010). Microsoft Forms helpfully prepares some sim-
ple pie and bar charts, which were supplemented with
more detailed graphs prepared in matplotlib (Hunter,
2007) and seaborn (Waskom, 2021).
3 RESULTS
3.1 Reliability
The Likert ratings were first examined to ensure suf-
ficient reliability using Cronbach’s alpha measure as
carried out in the work of. We reported a high alpha
coefficient of 0.964177 within a confidence interval
of 0.957 - 0.971 a which can be interpreted to indicate
that the scale we have used has high internal consis-
tency.
3.2 Descriptive Statistics
3.2.1 General Confidence Profiles
Figure 1 shows the distribution of responses for the
general questions gauging participants’ confidence in
the high-level category descriptors. While not as pre-
cise, these broad category questions act as a useful
1
https://aec-music.eu/news-article/digital-skills-4-
music-teachers-disk/
A Quantitative Survey of Digital Competencies of Music Teachers in the European Union
615