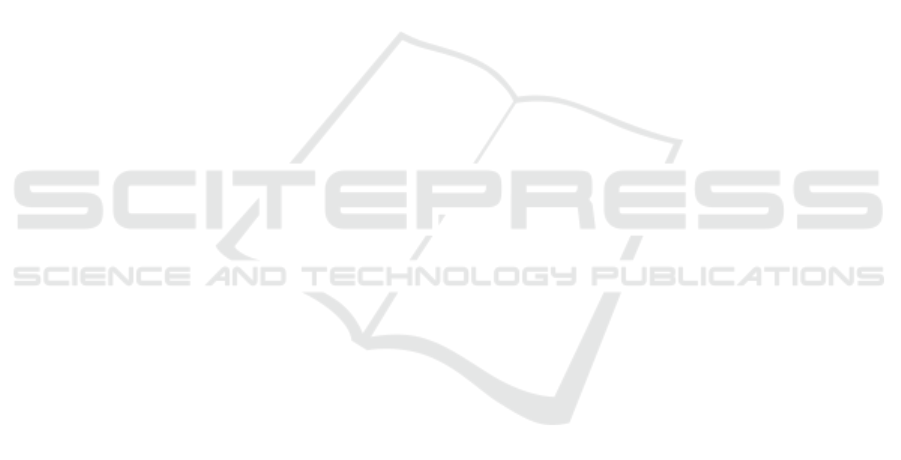
and heuristic optimization-based methods for deter-
mining the flexibility potential at vertical system in-
terconnections. In 2021 IEEE PES Innovative Smart
Grid Technologies Europe (ISGT Europe), pages 1–6.
Ghysels, E., Santa-Clara, P., and Valkanov, R. (2004). The
midas touch: Mixed data sampling regression models.
Gladyshev, P. and Patel, A. (2004). Finite state machine
approach to digital event reconstruction. Digital In-
vestigation, 1(2):130–149.
Gottschalk, M., Uslar, M., and Delfs, C. (2017). The use
case and smart grid architecture model approach: the
IEC 62559-2 use case template and the SGAM applied
in various domains. Springer.
Haarnoja, T., Zhou, A., Abbeel, P., and Levine, S. (2018).
Soft actor-critic: Off-policy maximum entropy deep
reinforcement learning with a stochastic actor.
Hopcroft, J. E., Motwani, R., and Ullman, J. D. (2001). In-
troduction to automata theory, languages, and compu-
tation. Acm Sigact News, 32(1):60–65.
Hossain, R. R., Yin, T., Du, Y., Huang, R., Tan, J., Yu,
W., Liu, Y., and Huang, Q. (2023). Efficient learn-
ing of power grid voltage control strategies via model-
based deep reinforcement learning. Machine Learn-
ing, pages 1–26.
Jagdale, D. (2021). Finite state machine in game develop-
ment. algorithms, 10(1).
Janner, M., Li, Q., and Levine, S. (2021). Offline reinforce-
ment learning as one big sequence modeling problem.
Proc. NeurIPS, page 1273–1286.
Jiang, N. and Li, L. (2016). Doubly robust off-policy value
evaluation for reinforcement learning. Proc. ICML,
page 652–661.
Kayastha, N., Niyato, D., Hossain, E., and Han, Z. (2014).
Smart grid sensor data collection, communication,
and networking: a tutorial. Wireless communications
and mobile computing, 14(11):1055–1087.
Kaygusuz, C., Babun, L., Aksu, H., and Uluagac, A. S.
(2018). Detection of compromised smart grid devices
with machine learning and convolution techniques. In
2018 IEEE International Conference on Communica-
tions (ICC), pages 1–6. IEEE.
Kidambi, R., Rajeswaran, A., Netrapalli, P., and Joachims,
T. (2020). Morel : Model-based offline reinforcement
learning. Proc. NeurIPS, page 21810–21823.
Kostrikov, I., Nair, A., and Levine, S. (2021). Offline rein-
forcement learning with implicit q-learning.
Kumar, A., Fu, J., Tucker, G., and Levine, S. (2019). Learn-
ing new attack vectors from misuse cases with deep
reinforcement learning. Proc. NeurIPS, pages 1–11.
Lange, S., Gabel, T., and Riedmiller, M. (2012). Reinforce-
ment learning, chapter Batch reinforcement learning,
page pages 45–73. Springer.
Levine, S., Kumar, A., Tucker, G., and Fu, J. (2020). Offline
reinforcement learning: Tutorial, review, and perspec-
tives on open problems.
Matchev, K. T., Roman, A., and Shyamsundar, P. (2022).
Uncertainties associated with gan-generated datasets
in high energy physics. SciPost Physics, 12(3):104.
Mayer, C., Brunekreeft, G., Blank-Babazadeh, M., Stark,
S., Buchmann, M., Dalheimer, M., et al. (2020).
Resilienz digitalisierter energiesysteme. Blackout-
Risiken Verstehen, Stromversorgung Sicher Gestalten.
Nair, A., Gupta, A., Dalal, M., and Levine, S. (2020). Awac:
Accelerating online reinforcement learning with of-
fline datasets. arXiv preprint arXiv:2006.09359.
Panaganti, K., Xu, Z., Kalathil, D., and Ghavamzadeh, M.
(2022). Robust reinforcement learning using offline
data. Advances in neural information processing sys-
tems, 35:32211–32224.
Peng, X. B., Kumar, A., Zhang, G., and Levine, S. (2019).
Advantage-weighted regression: Simple and scalable
off-policy reinforcement learning.
Precup, D., Sutton, R., and Singh, S. (2000). Eligibility
traces for off-policy policy evaluation. Proc. ICML,
page 759–766.
Prudencio, R. F., Maximo, M. R. O. A., and Colombini,
E. L. (2022). A survey on offline reinforcement learn-
ing: Taxonomy, review, and open problems.
Rhodes, J. D., Upshaw, C. R., Harris, C. B., Meehan, C. M.,
Walling, D. A., Navr
´
atil, P. A., Beck, A. L., Naga-
sawa, K., Fares, R. L., Cole, W. J., et al. (2014). Ex-
perimental and data collection methods for a large-
scale smart grid deployment: Methods and first re-
sults. Energy, 65:462–471.
Sch
¨
utz, J., Uslar, M., and Clausen, M. (2022).
Digitalisierung. Synthesebericht 3 des SINTEG
F
¨
orderprogramms, Studie im Auftrag des BMWK,
Berlin. Berlin.
Steinbrink, C., Blank-Babazadeh, M., El-Ama, A., Holly,
S., L
¨
uers, B., Nebel-Wenner, M., Ram
´
ırez Acosta,
R. P., Raub, T., Schwarz, J. S., Stark, S., Nieße, A.,
and Lehnhoff, S. (2019). Cpes testing with mosaik:
Co-simulation planning, execution and analysis. Ap-
plied Sciences, 9(5).
Trigkas, D., Ziogou, C., Voutetakis, S., and Papadopoulou,
S. (2018). Supervisory control of energy distribu-
tion at autonomous res-powered smart-grids using a
finite state machine approach. In 2018 5th Interna-
tional Conference on Control, Decision and Informa-
tion Technologies (CoDIT), pages 415–420. IEEE.
Tu, C., He, X., Shuai, Z., and Jiang, F. (2017). Big data
issues in smart grid–a review. Renewable and Sus-
tainable Energy Reviews, 79:1099–1107.
Uslar, M. (2015). Energy Informatics: Definition, State-
of-the-art and new horizons. In Kupzog, F., editor,
Proceedings der ComForEn 2015 Vienna, Wien. TU
Wien, OVE Verlag.
Uslar, M., Rohjans, S., Neureiter, C., Pr
¨
ostl Andr
´
en, F.,
Velasquez, J., Steinbrink, C., Efthymiou, V., Migli-
avacca, G., Horsmanheimo, S., Brunner, H., et al.
(2019). Applying the smart grid architecture model
for designing and validating system-of-systems in the
power and energy domain: A european perspective.
Energies, 12(2):258.
Veith, E., Balduin, S., Wenninghoff, N., Wolgast, T.,
Baumann, M., Winkler, D., Hammer, L., Salman,
A., Schulz, M., Raeiszadeh, A., Logemann, T., and
Wellßow, A. (2023). palaestrAI: A training ground
Trajectory Generation Model: Building a Simulation Link Between Expert Knowledge and Offline Learning
101