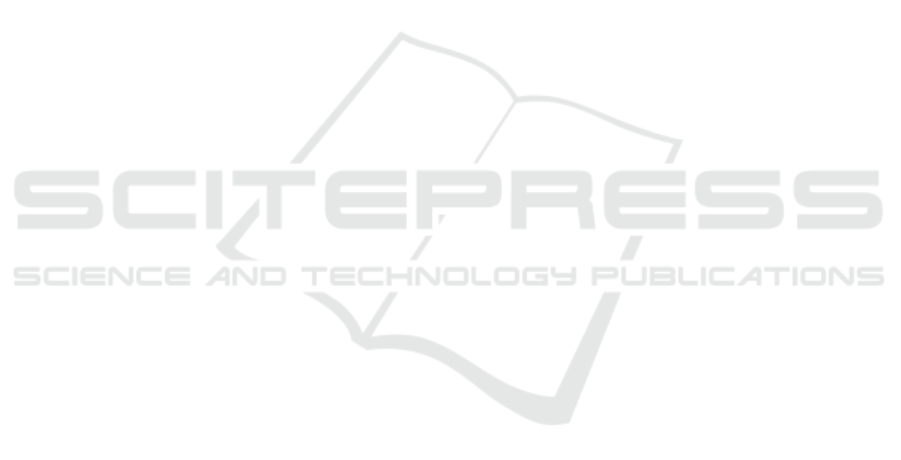
6 CONCLUSION AND
PERSPECTIVES
This paper presents a proactive solution leveraging
smartphone technology for real-time detection and
notification of road surface anomalies. Through the
integration of machine learning techniques and ac-
celerometer data, our turn-by-turn navigation sys-
tem effectively identifies and alerts drivers to po-
tential road surface anomalies, thereby enhancing
overall road safety. The evaluation of our system
demonstrated promising results, with the classifica-
tion model exhibiting high precision and recall rates
in detecting anomalies. Furthermore, the implemen-
tation of federated learning proved instrumental in re-
fining the detection model’s performance across di-
verse situations, highlighting the efficacy of collabo-
rative learning approaches in improving detection ac-
curacy while preserving user privacy. Overall, our
system offers a practical and effective approach to ad-
dressing road safety concerns, with the potential to
significantly reduce the incidence of accidents and
improve the overall driving experience. As future
work, further optimization and refinement of the de-
tection model could be explored, along with the in-
tegration of additional sensors or data sources to en-
hance anomaly detection capabilities in various road
conditions. The most promising additional sensor is
the camera technology that can augment anomaly de-
tection capabilities. Cameras can capture visual in-
formation about road surface conditions, allowing for
the detection of anomalies before vehicles encounter
them.
DISCLOSURE OF AI TOOLS
USAGE
The preparation of this manuscript involved the use
of Copilote and ChatGPT to correct and improve the
language through the manuscript. Subsequently, the
authors reviewed and edited the content as necessary,
and take full responsibility for the paper’s content.
REFERENCES
Al-Sabaeei, A. M., Souliman, M. I., and Jagadeesh, A.
(2024). Smartphone applications for pavement condi-
tion monitoring: A review. Construction and Building
Materials, 410:134207.
Chen, C., Seo, H., and Zhao, Y. (2022). A novel pavement
transverse cracks detection model using wt-cnn and
stft-cnn for smartphone data analysis. International
Journal of Pavement Engineering, 23(12):4372–4384.
Dong, D. and Li, Z. (2021). Smartphone sensing of
road surface condition and defect detection. Sensors,
21(16).
Jan, M., Khattak, K. S., Khan, Z. H., Gulliver, T. A., and
Altamimi, A. B. (2023). Crowdsensing for road pave-
ment condition monitoring: Trends, limitations, and
opportunities. IEEE Access, 11:133143–133159.
Jeong, J.-H. and Jo, H. (2024). Toward real-world
implementation of deep learning for smartphone-
crowdsourced pavement condition assessment. IEEE
Internet of Things Journal, 11(4):6328–6337.
Kim, M. J. and Kim, Y. M. (2023). Rss model improvement
considering road conditions for the application of a
variable focus function camera. Sensors, 23(2).
Kosakowska, K. (2022). Evaluation of the impact of speed
bumps on the safety of residents-selected aspects.
Transportation research procedia, 60:418–423.
Lab, P. (2016). Roadway surface dis-
ruptions dataset, [online] available:
https://www.accelerometer.xyz/pothole lab/.
Lee, T., Chun, C., and Ryu, S.-K. (2021). Detection of road-
surface anomalies using a smartphone camera and ac-
celerometer. Sensors, 21(2).
Martinelli, A., Meocci, M., Dolfi, M., Branzi, V., Morosi,
S., Argenti, F., Berzi, L., and Consumi, T. (2022).
Road surface anomaly assessment using low-cost ac-
celerometers: A machine learning approach. Sensors,
22(10).
Mazari Abdessameud, O., Cherifi, W., Kribi, M. A. E. I.,
and Dahmani, A. (2022). Navisaf: A safe navigation
system for road anomalies detection. In IECON 2022–
48th Annual Conference of the IEEE Industrial Elec-
tronics Society, pages 1–6. IEEE.
Rahiman V, A. et al. (2021). Pothole detection and volume
estimation based on disparity transformation with his-
togram thresholding. Proceedings of the Yukthi 2021-
The International Conference on Emerging Trends in
Engineering.
Sholevar, N., Golroo, A., and Esfahani, S. R. (2022).
Machine learning techniques for pavement condition
evaluation. Automation in Construction, 136:104190.
Tao, D., Cheng, J., Yu, Z., Yue, K., and Wang, L. (2018).
Domain-weighted majority voting for crowdsourcing.
IEEE transactions on neural networks and learning
systems, 30(1):163–174.
Tomiło, P. (2023). Classification of the condition of
pavement with the use of machine learning meth-
ods. Transport and Telecommunication Journal,
24(2):158–166.
Xin, H., Ye, Y., Na, X., Hu, H., Wang, G., Wu, C., and
Hu, S. (2023). Sustainable road pothole detection: A
crowdsourcing based multi-sensors fusion approach.
Sustainability, 15(8).
Yuan, W., Yang, Q., and Zhang, R. (2023). Asphalt pave-
ment surface repair areas detection based on smart-
phone sensors. International Journal of Transporta-
tion Science and Technology.
Federated Road Surface Anomaly Detection Using Smartphone Accelerometer Data
383